Spotting Virus From Satellites: Modeling the Circulation of West Nile Virus Through Graph Neural Networks
CoRR(2023)
摘要
The occurrence of West Nile virus (WNV) represents one of the most common mosquito-borne zoonosis viral infections. Its circulation is usually associated with climatic and environmental conditions suitable for vector proliferation and virus replication. On top of that, several statistical models have been developed to shape and forecast WNV circulation: in particular, the recent massive availability of Earth observation (EO) data coupled with the continuous advances in the field of artificial intelligence offer valuable opportunities. In this article, we seek to predict WNV circulation by feeding deep neural networks (DNNs) with satellite images, which have been extensively shown to hold environmental and climatic features. Notably, while previous approaches analyze each geographical site independently, we propose a spatial-aware approach that considers also the characteristics of close sites. Specifically, we build upon graph neural networks (GNNs) to aggregate features from neighboring places and further extend these modules to consider multiple relations, such as the difference in temperature and soil moisture between two sites, as well as the geographical distance. Moreover, we inject time-related information directly into the model to take into account the seasonality of virus spread. We design an experimental setting that combines satellite images—from Landsat and Sentinel missions—with ground-truth observations of WNV circulation in Italy. We show that our proposed multiadjacency graph attention network (MAGAT) consistently leads to higher performance when paired with an appropriate pretraining stage. Finally, we assess the importance of each component of MAGAT in our ablation studies.
更多查看译文
关键词
west nile
AI 理解论文
溯源树
样例
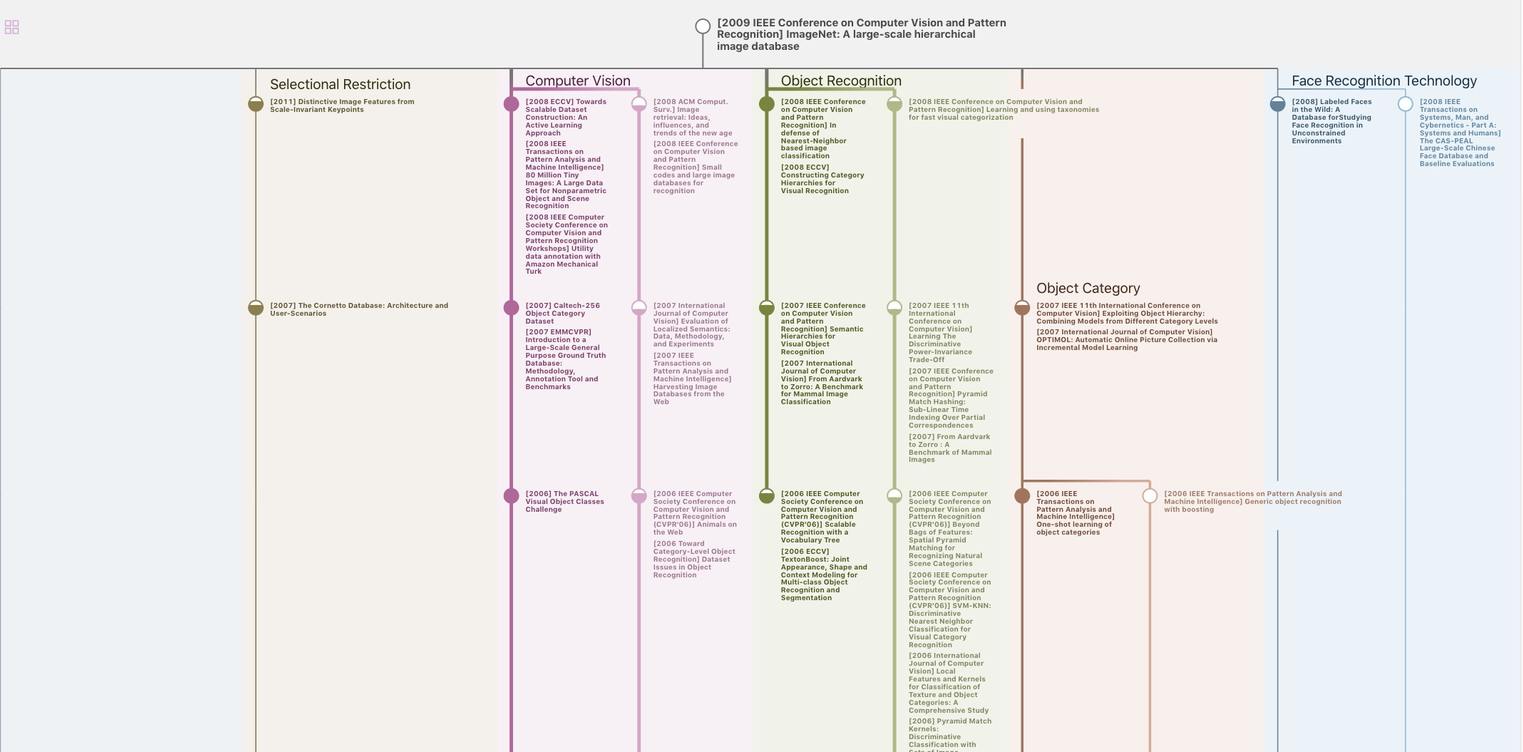
生成溯源树,研究论文发展脉络
Chat Paper
正在生成论文摘要