Population-Based Hierarchical Non-Negative Matrix Factorization for Survey Data
2022 IEEE/ACM International Conference on Big Data Computing, Applications and Technologies (BDCAT)(2022)
摘要
Motivated by the problem of identifying potential hierarchical population structure on modern survey data containing a wide range of complex data types, we introduce population-based hierarchical non-negative matrix factorization (PHNMF). PHNMF is a variant of hierarchical non-negative matrix factorization based on feature similarity. As such, it enables an automatic and interpretable approach for identifying and understanding hierarchical structure in a data matrix constructed from a wide range of data types. Our numerical experiments on synthetic and real survey data demonstrate that PHNMF can recover latent hierarchical population structure in complex data with high accuracy. Moreover, the recovered subpopulation structure is meaningful and can be useful for improving downstream inference.
更多查看译文
关键词
Non-negative matrix factorization,hierarchical clustering,survey data,latent classes,population structure
AI 理解论文
溯源树
样例
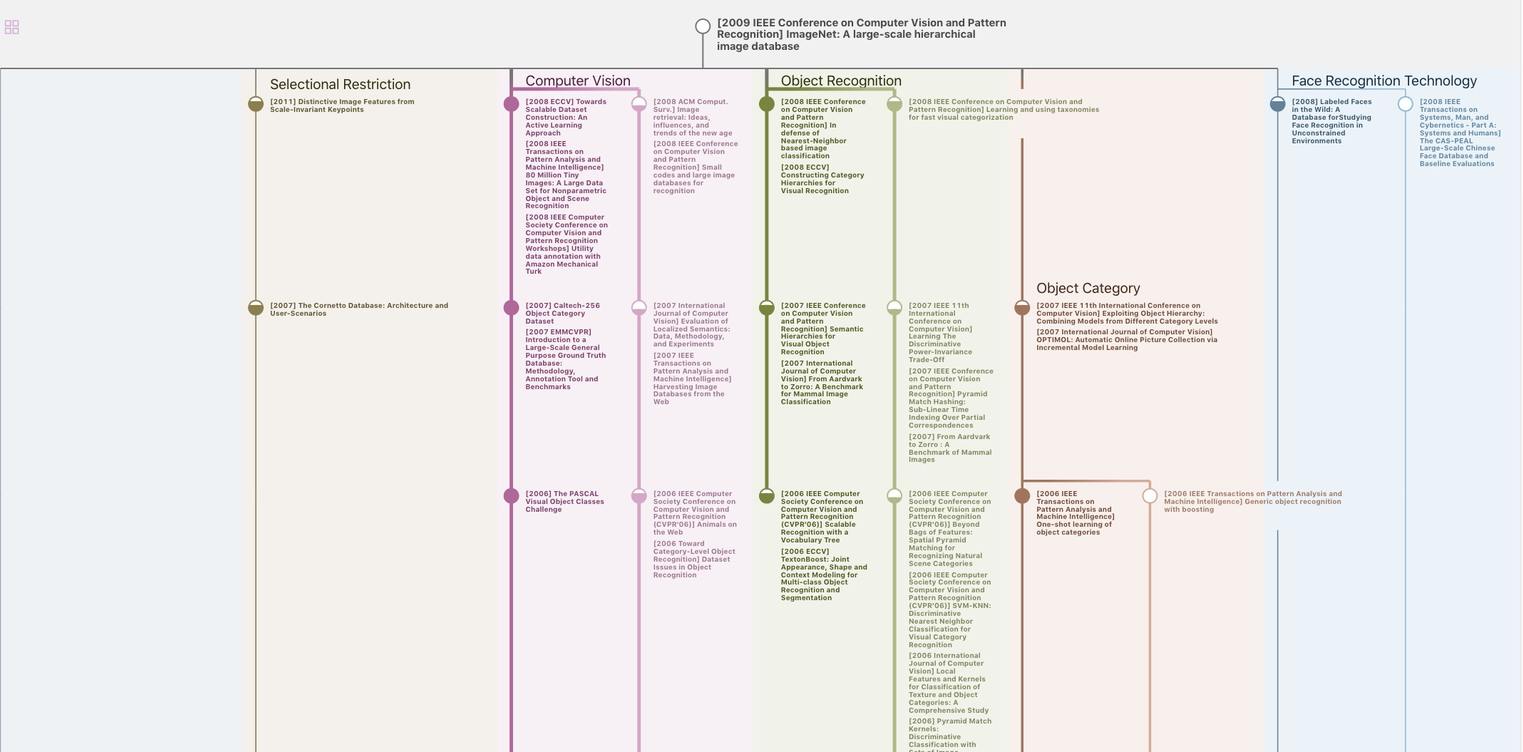
生成溯源树,研究论文发展脉络
Chat Paper
正在生成论文摘要