Tensor Completion via Tensor Train Based Low-Rank Quotient Geometry under a Preconditioned Metric
arXiv (Cornell University)(2023)
Abstract
This paper investigates the low-rank tensor completion problem, which is about recovering a tensor from partially observed entries. We consider this problem in the tensor train format and extend the preconditioned metric from the matrix case to the tensor case. The first-order and second-order quotient geometry of the manifold of fixed tensor train rank tensors under this metric is studied in detail. Algorithms, including Riemannian gradient descent, Riemannian conjugate gradient, and Riemannian Gauss-Newton, have been proposed for the tensor completion problem based on the quotient geometry. It has also been shown that the Riemannian Gauss-Newton method on the quotient geometry is equivalent to the Riemannian Gauss-Newton method on the embedded geometry with a specific retraction. Empirical evaluations on random instances as well as on function-related tensors show that the proposed algorithms are competitive with other existing algorithms in terms of recovery ability, convergence performance, and reconstruction quality.
MoreTranslated text
Key words
tensor completion,tensor train,low-rank
AI Read Science
Must-Reading Tree
Example
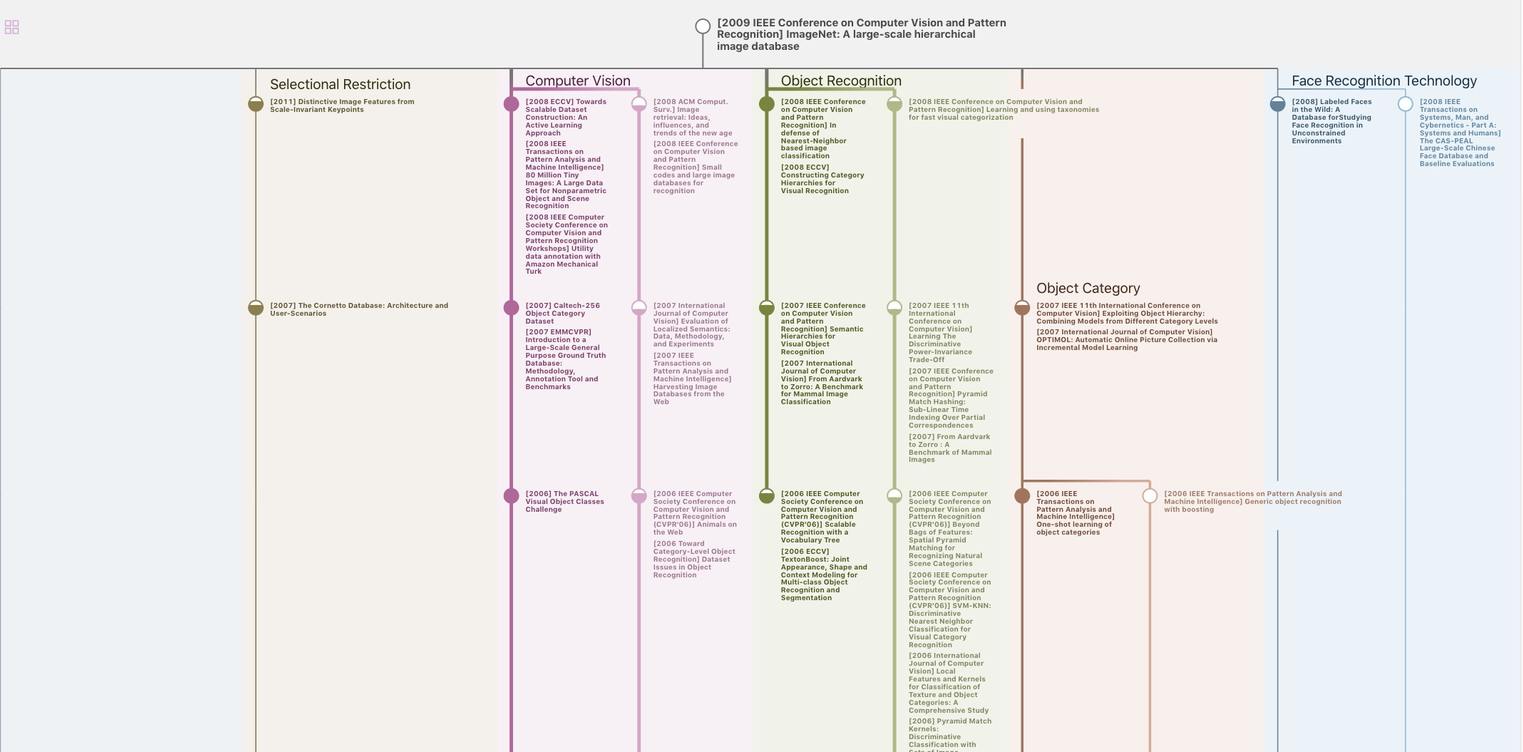
Generate MRT to find the research sequence of this paper
Chat Paper
Summary is being generated by the instructions you defined