Learning Enabled Fast Planning and Control in Dynamic Environments with Intermittent Information
2022 IEEE/RSJ INTERNATIONAL CONFERENCE ON INTELLIGENT ROBOTS AND SYSTEMS (IROS)(2022)
Abstract
This paper addresses a safe planning and control problem for mobile robots operating in communication- and sensor-limited dynamic environments. In this case the robots cannot sense the objects around them and must instead rely on intermittent, external information about the environment, as e.g., in underwater applications. The challenge in this case is that the robots must plan using only this stale data, while accounting for any noise in the data or uncertainty in the environment. To address this challenge we propose a compositional technique which leverages neural networks to quickly plan and control a robot through crowded and dynamic environments using only intermittent information. Specifically, our tool uses reachability analysis and potential fields to train a neural network that is capable of generating safe control actions. We demonstrate our technique both in simulation with an underwater vehicle crossing a crowded shipping channel and with real experiments with ground vehicles in communicationand sensor-limited environments.
MoreTranslated text
AI Read Science
Must-Reading Tree
Example
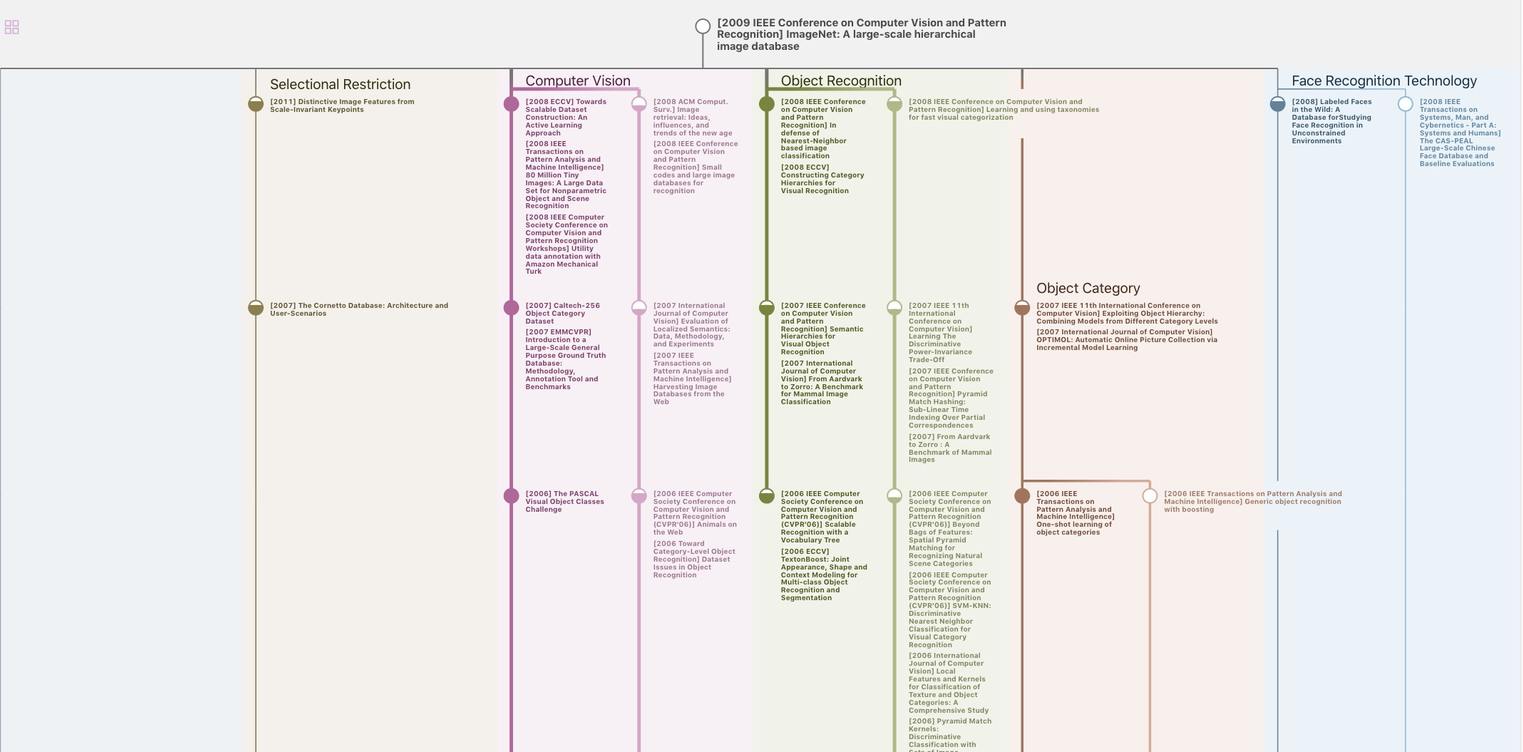
Generate MRT to find the research sequence of this paper
Chat Paper
Summary is being generated by the instructions you defined