Learning sparse auto-encoders for green AI image coding
arxiv(2022)
摘要
Recently, convolutional auto-encoders (CAE) were introduced for image coding. They achieved performance improvements over the state-of-the-art JPEG2000 method. However, these performances were obtained using massive CAEs featuring a large number of parameters and whose training required heavy computational power.\\ In this paper, we address the problem of lossy image compression using a CAE with a small memory footprint and low computational power usage. In order to overcome the computational cost issue, the majority of the literature uses Lagrangian proximal regularization methods, which are time consuming themselves.\\ In this work, we propose a constrained approach and a new structured sparse learning method. We design an algorithm and test it on three constraints: the classical $\ell_1$ constraint, the $\ell_{1,\infty}$ and the new $\ell_{1,1}$ constraint. Experimental results show that the $\ell_{1,1}$ constraint provides the best structured sparsity, resulting in a high reduction of memory and computational cost, with similar rate-distortion performance as with dense networks.
更多查看译文
关键词
classical ℓ1,1 constraint,computational cost reduction,computational power usage,convolutional auto-encoders,green AI image coding,heavy computational power,lossy image compression,massive CAE,memory footprint,performance improvements,rate-distortion performance,sparse auto-encoders,state-of-the-art JPEG2000 method,structured sparse learning method
AI 理解论文
溯源树
样例
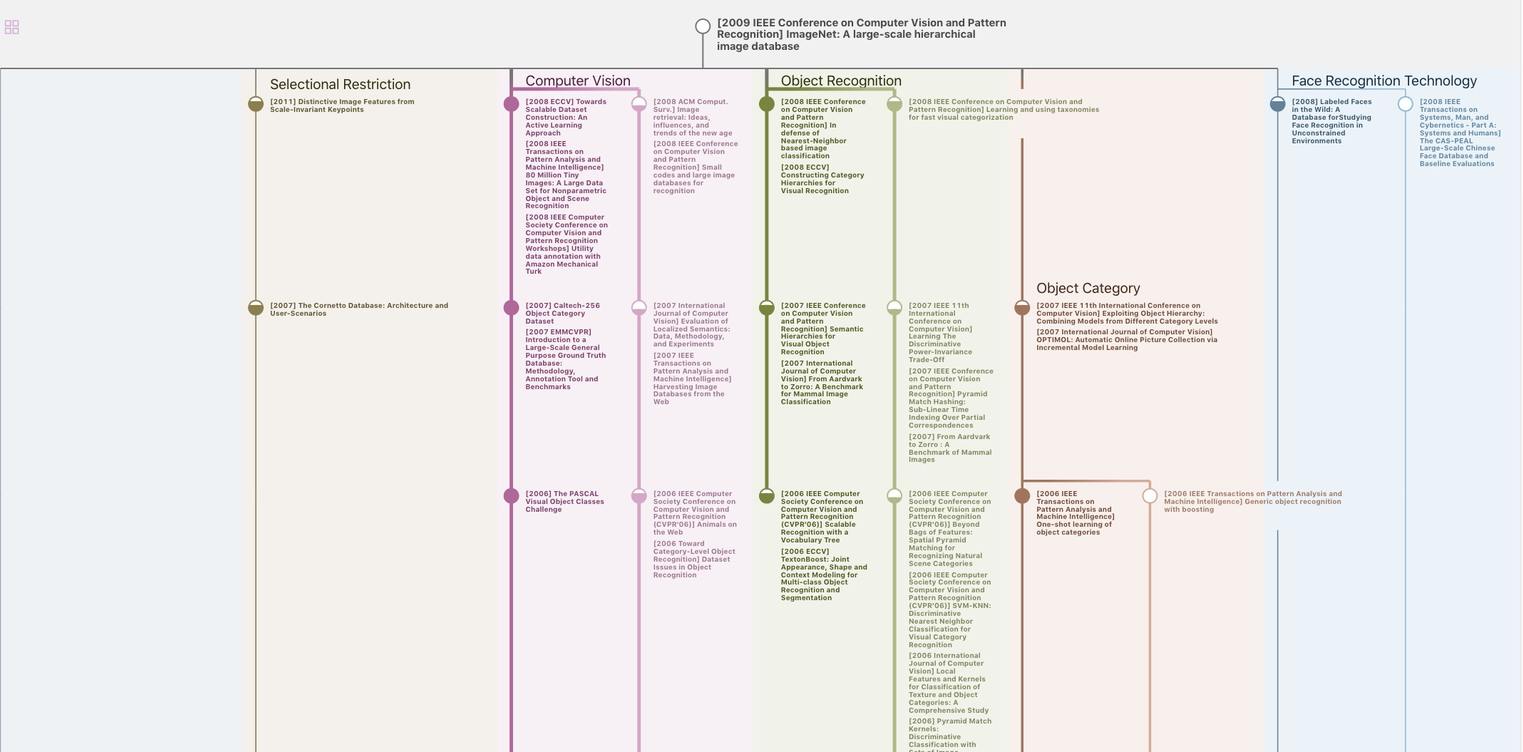
生成溯源树,研究论文发展脉络
Chat Paper
正在生成论文摘要