Hybrid Spatiotemporal Graph Convolutional Network for Detecting Landscape Pattern Evolution From Long-Term Remote Sensing Images
IEEE TRANSACTIONS ON GEOSCIENCE AND REMOTE SENSING(2022)
摘要
The remote sensing time series change detection algorithm based on the pixel or single landscape patch ignores the change analysis of spatial structure information. Inspired by graph convolutional network (GCN) modeling, a set of landscapes (nodes) and their relationships (edges) are proposed. In this study, a parallel strategy in spatial-GCN and progressive strategy in temporal-GCN, called the hybrid GCN model network as a holistic framework, was proposed to accurately capture both spatial and temporal variations in landscape patterns based on yearly Landsat time series. A superpatch (i.e., fixed patch class surrounded by a one-hop neighbor patch) was selected as the input of the GCN network. First, a spatial-GCN model with three parallel graph convolutional layers was adopted to classify landscape pattern types. Three dominant categories of landscape patterns over the past three decades have been identified. Second, four landscape metrics in superpatches were proposed for the quantitative characterization of changes in landscape patterns. Finally, a temporal-GCN model with two progressive graph convolutional layers was used to detect six types of patch changes, which were applied to continuously detect the landscape pattern evolution processes. Regardless of the spatial-GCN and temporal-GCN, they provided satisfactory performance using the training and validation sets with overall accuracy >92% and Kappa coefficient >0.90, and loss values converging to 0.049 and 0.128, respectively, based on NLLLoss function until 500 epochs. It is believed that the hybrid GCN model has great potential for mining possible implicit spatiotemporal relationships and future evolution of landscape patterns.
更多查看译文
关键词
Graph convolutional network (GCN),Landsat time series,landscape pattern,superpatch spatial graph
AI 理解论文
溯源树
样例
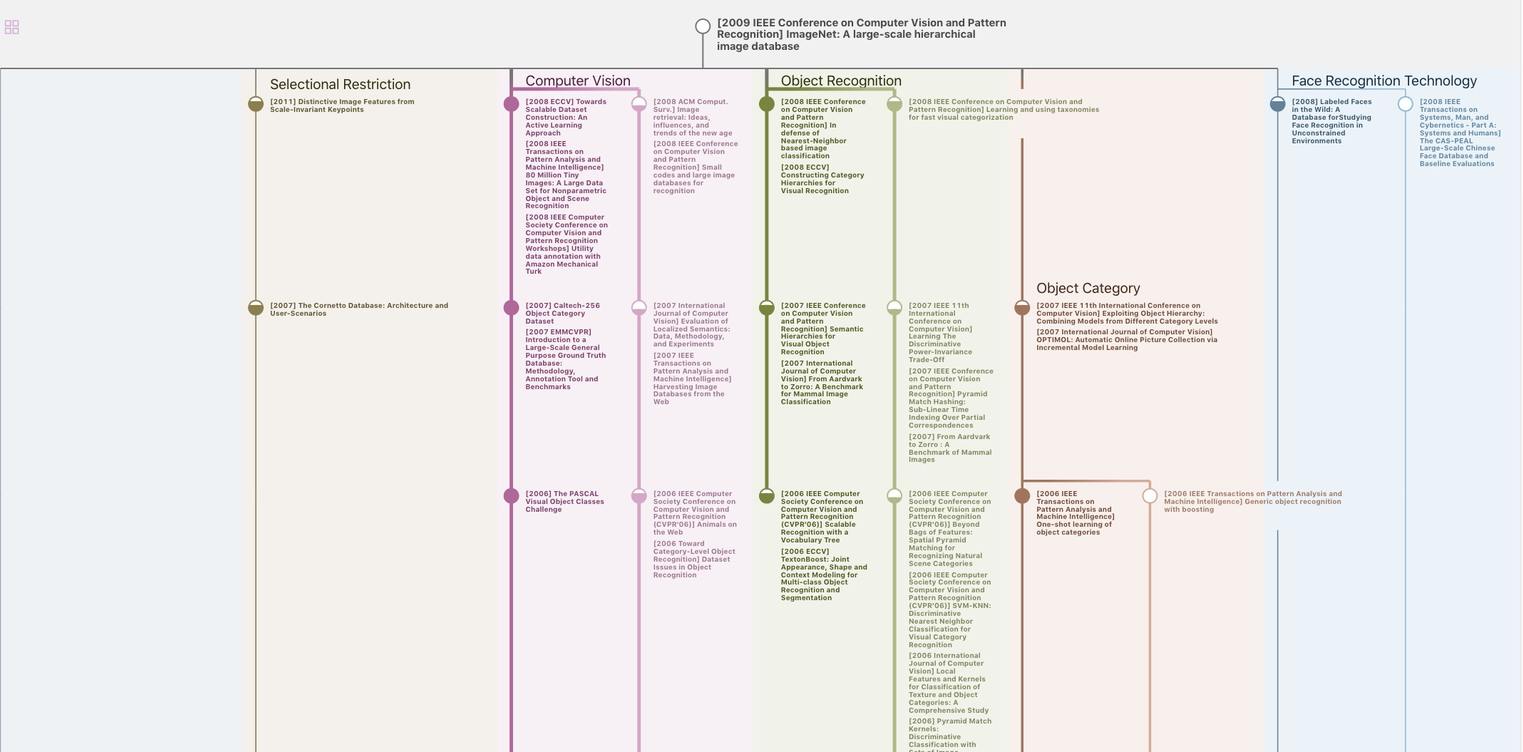
生成溯源树,研究论文发展脉络
Chat Paper
正在生成论文摘要