Self-discharge prediction method for lithium-ion batteries based on improved support vector machine
Journal of Energy Storage(2022)
摘要
An improved support vector regression (SVR) method is proposed for predicting the self-discharge voltage drop (SDV-drop) in lithium-ion batteries. Multiple features were extracted according to the charge and discharge curves of lithium-ion batteries, and the three features having the strongest correlation with the SDV-drop were identified via grey relational analysis. Then, these three features were assigned different weight parameters to obtain composite features which were input into the improved support vector machine through differential evolution algorithm parameter optimization training. Finally, the improved SVR model was obtained. Model training and testing were performed via a battery charge and discharge experiment and battery static experimental data of a new energy vehicle company, and the results indicated that the proposed method had a higher prediction accuracy than the neural-network model and the Gaussian process regression model.
更多查看译文
关键词
Battery self-discharge, Support vector regression, Data-driven models, Charge-discharge curves
AI 理解论文
溯源树
样例
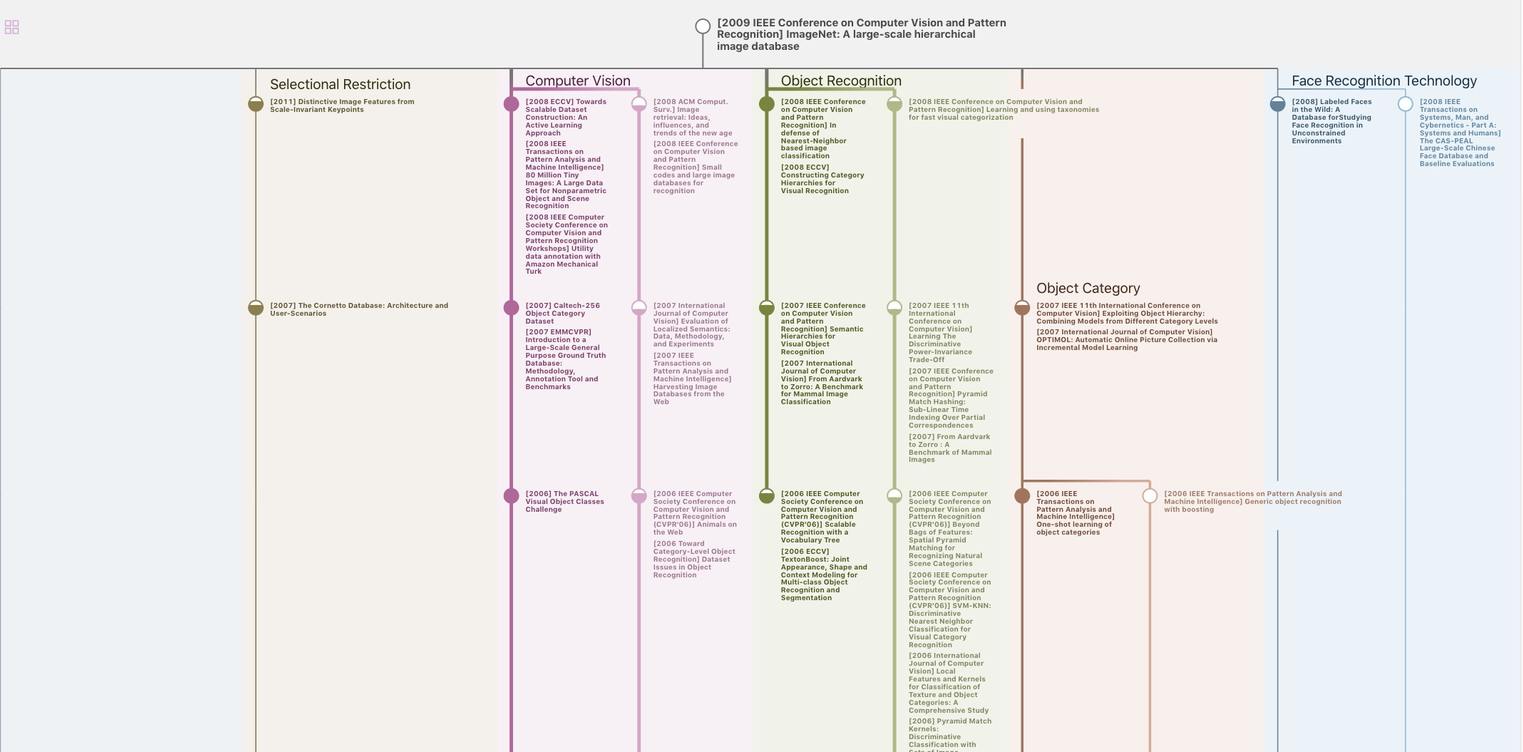
生成溯源树,研究论文发展脉络
Chat Paper
正在生成论文摘要