Hardware-Accelerated Real-time Drift-awareness for Robust Deep Learning on Wireless RF Data
ACM Transactions on Reconfigurable Technology and Systems(2022)
摘要
Proactive and intelligent management of network resource utilization (RU) using deep learning (DL) can significantly improve the efficiency and performance of the next generation of wireless networks. However, variations in wireless RU are often affected by uncertain events and change points due to the deviations of real data distribution from that of the original training data. Such deviations which are known as dataset drifts can subsequently lead to a shift in the corresponding decision boundary degrading the DL model prediction performance. To address these challenges, we present hardware-accelerated real-time radio frequency (RF) analytics and drift-awareness modules for robust DL predictions. We have prototyped the proposed design on a Zynq-7000 System-on-Chip which contains an FPGA and an embedded ARM processor. We have used Xilinx Vivado design suite for synthesis and analysis of the HDL design for the proposed solution. To detect dataset drifts, the proposed solution adopts a distance-based technique on FPGA to quantify in real-time the change between the prediction distribution obtained from DL predictions and data distribution of input streaming samples. Using various performance metrics, we have extensively evaluated the performance of the proposed solution and shown that it can significantly improve the DL model robustness in the presence of dataset drifts.
更多查看译文
关键词
robust deep learning,deep learning,hardware-accelerated,real-time,drift-awareness
AI 理解论文
溯源树
样例
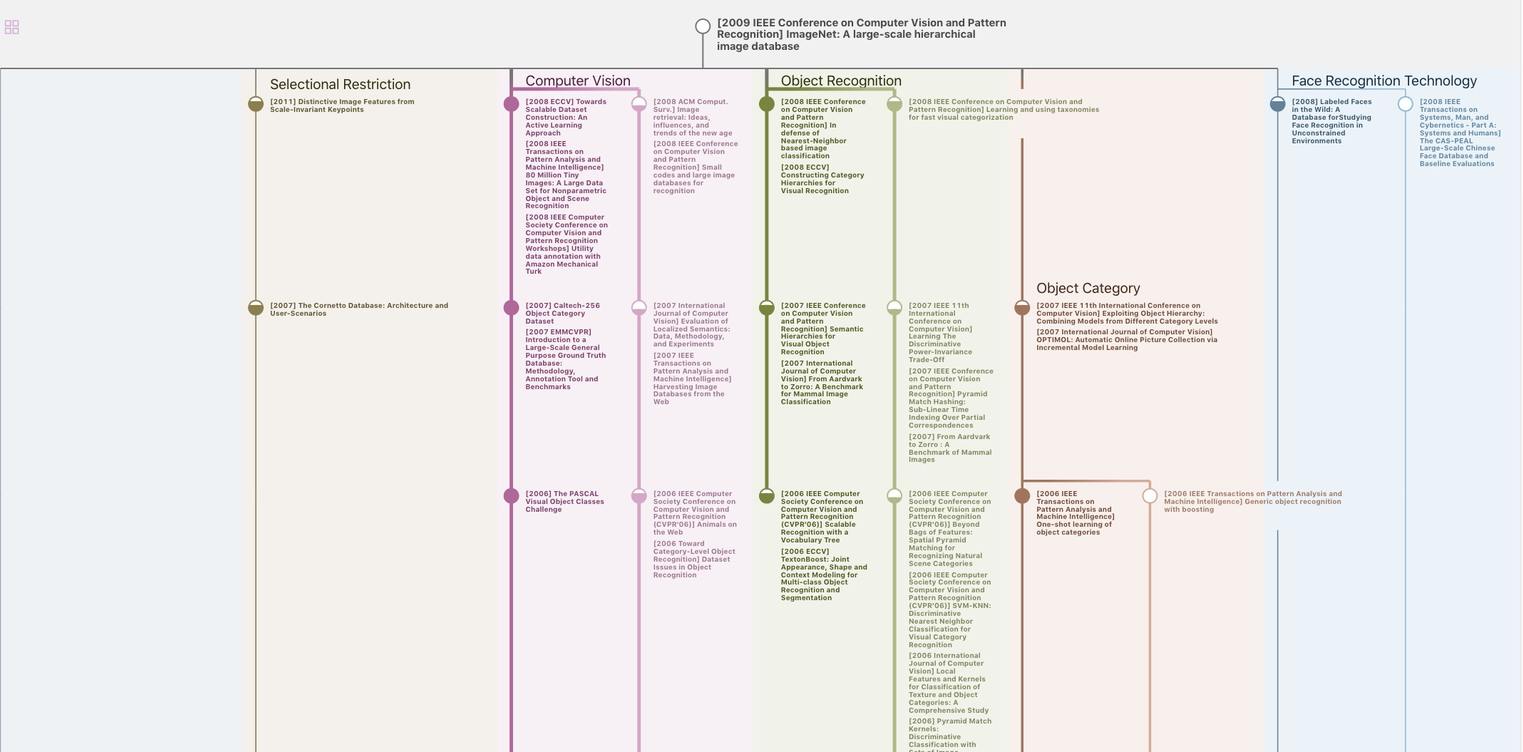
生成溯源树,研究论文发展脉络
Chat Paper
正在生成论文摘要