Ensemble learning algorithms with feature reduction mechanism for intrusion detection system
INTERNATIONAL JOURNAL OF INFORMATION AND COMPUTER SECURITY(2022)
摘要
One of the most significant requirements for improving the accuracy and performance of the detection engine in an intrusion detection system (IDS) is to identify and pick just the most important and relevant features. Due to the advancement of some ubiquitous technologies such as cloud computing, internet of things (IoTs), etc. large volumes of data are generated and shared in the network in every fraction of seconds that needs to be gathered and correctly analysed by the IDS. Although, every dataset consists of many features, altogether it may not contribute to identify legitimate traffic; therefore, reduction of irrelevant features and selection of only relevant attributes may enhance the accuracy and increase the speed of IDS. Thus, in order to eliminate the irrelevant features, we have proposed a features selection technique which recognises the important features and increase the performance of the detection engine based on the score of features establishment.
更多查看译文
关键词
intrusion detection system,random forest,gradient boosting,adaboost,extra-trees classifier,RFE,NSL-KDD dataset
AI 理解论文
溯源树
样例
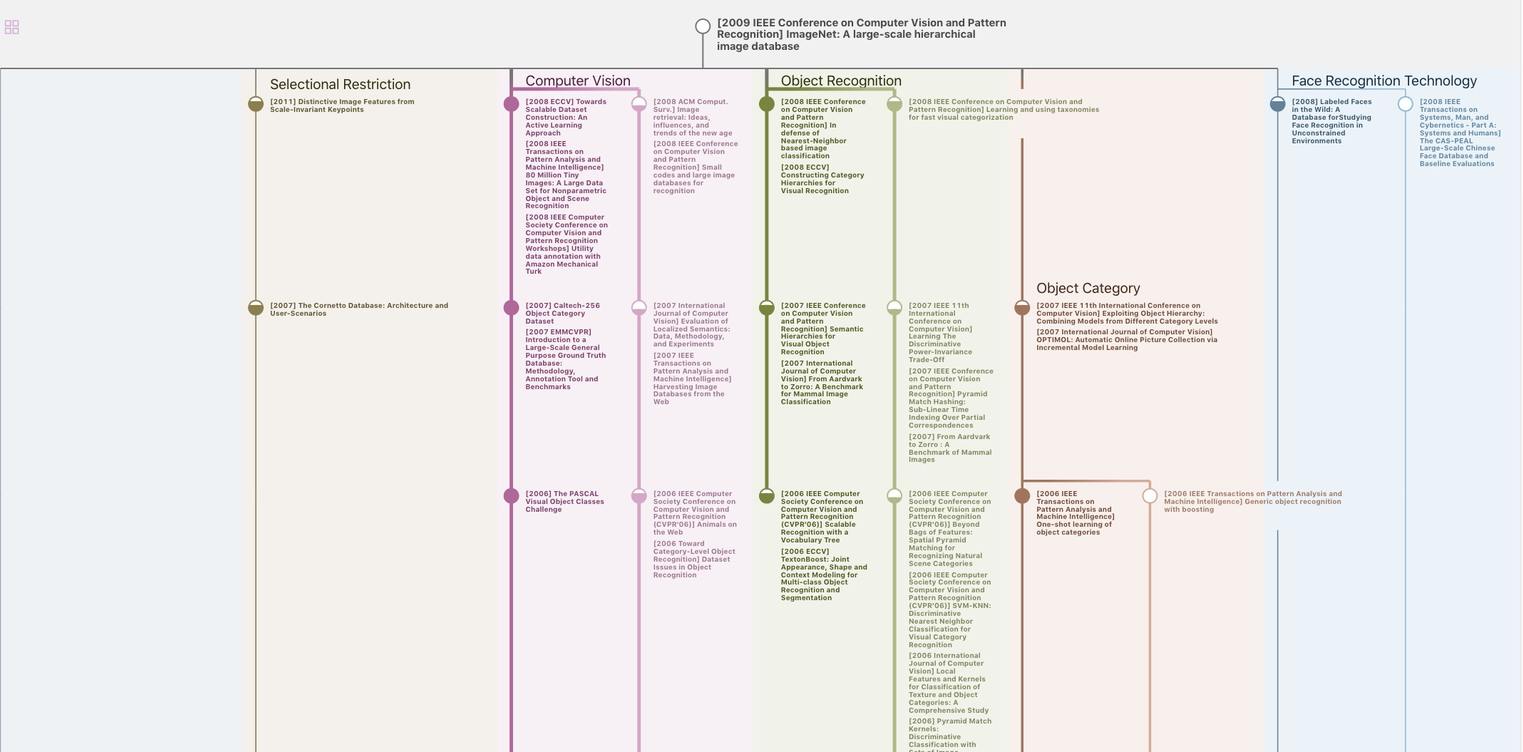
生成溯源树,研究论文发展脉络
Chat Paper
正在生成论文摘要