Robust-by-Design Classification via Unitary-Gradient Neural Networks
arxiv(2022)
摘要
The use of neural networks in safety-critical systems requires safe and robust models, due to the existence of adversarial attacks. Knowing the minimal adversarial perturbation of any input x, or, equivalently, knowing the distance of x from the classification boundary, allows evaluating the classification robustness, providing certifiable predictions. Unfortunately, state-of-the-art techniques for computing such a distance are computationally expensive and hence not suited for online applications. This work proposes a novel family of classifiers, namely Signed Distance Classifiers (SDCs), that, from a theoretical perspective, directly output the exact distance of x from the classification boundary, rather than a probability score (e.g., SoftMax). SDCs represent a family of robust-by-design classifiers. To practically address the theoretical requirements of a SDC, a novel network architecture named Unitary-Gradient Neural Network is presented. Experimental results show that the proposed architecture approximates a signed distance classifier, hence allowing an online certifiable classification of x at the cost of a single inference.
更多查看译文
关键词
classification,neural networks,robust-by-design,unitary-gradient
AI 理解论文
溯源树
样例
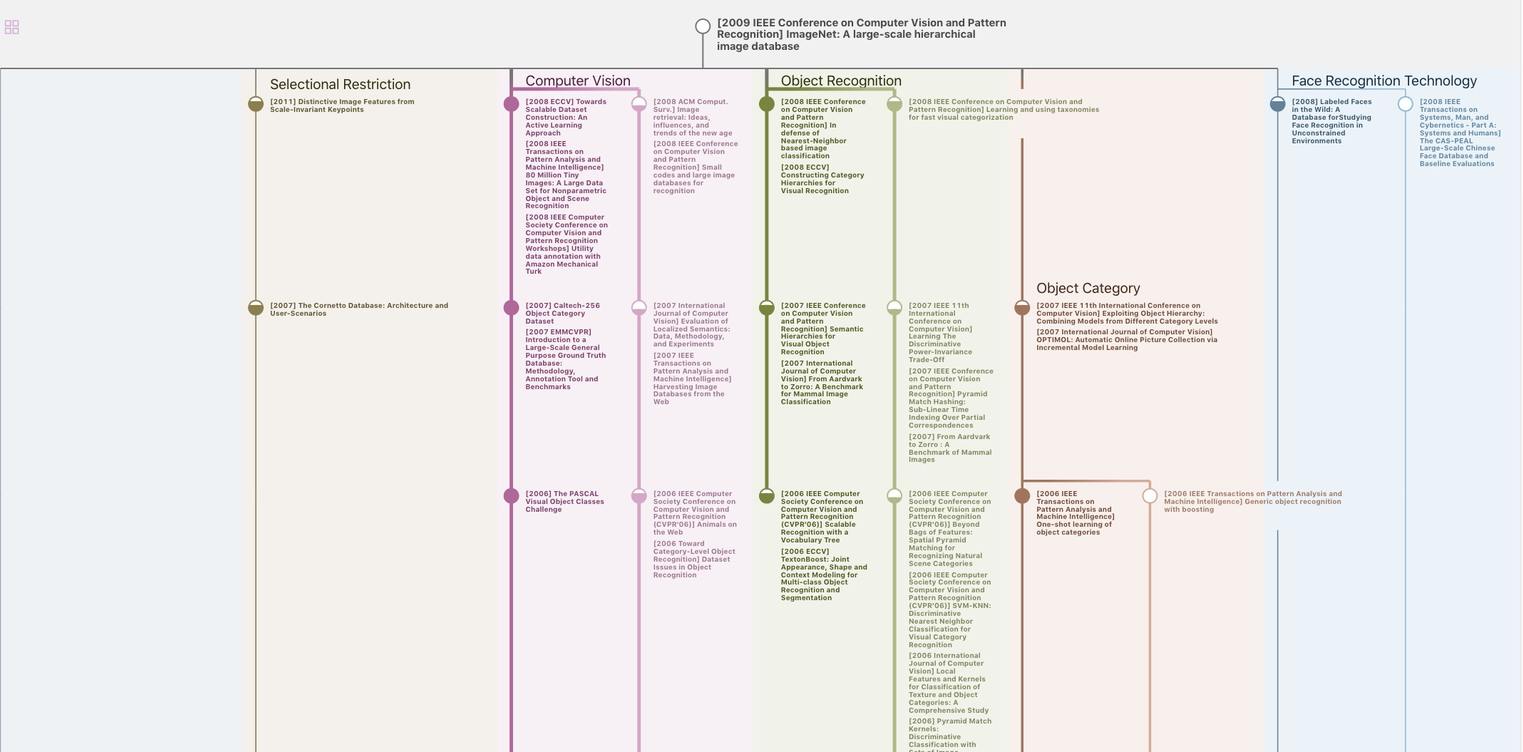
生成溯源树,研究论文发展脉络
Chat Paper
正在生成论文摘要