Simple and Powerful Architecture for Inductive Recommendation Using Knowledge Graph Convolutions
arxiv(2022)
摘要
Using graph models with relational information in recommender systems has shown promising results. Yet, most methods are transductive, i.e., they are based on dimensionality reduction architectures. Hence, they require heavy retraining every time new items or users are added. Conversely, inductive methods promise to solve these issues. Nonetheless, all inductive methods rely only on interactions, making recommendations for users with few interactions sub-optimal and even impossible for new items. Therefore, we focus on inductive methods able to also exploit knowledge graphs (KGs). In this work, we propose SimpleRec, a strong baseline that uses a graph neural network and a KG to provide better recommendations than related inductive methods for new users and items. We show that it is unnecessary to create complex model architectures for user representations, but it is enough to allow users to be represented by the few ratings they provide and the indirect connections among them without any user metadata. As a result, we re-evaluate state-of-the-art methods, identify better evaluation protocols, highlight unwarranted conclusions from previous proposals, and showcase a novel, stronger baseline for this task.
更多查看译文
关键词
knowledge graph convolutions,inductive recommendation
AI 理解论文
溯源树
样例
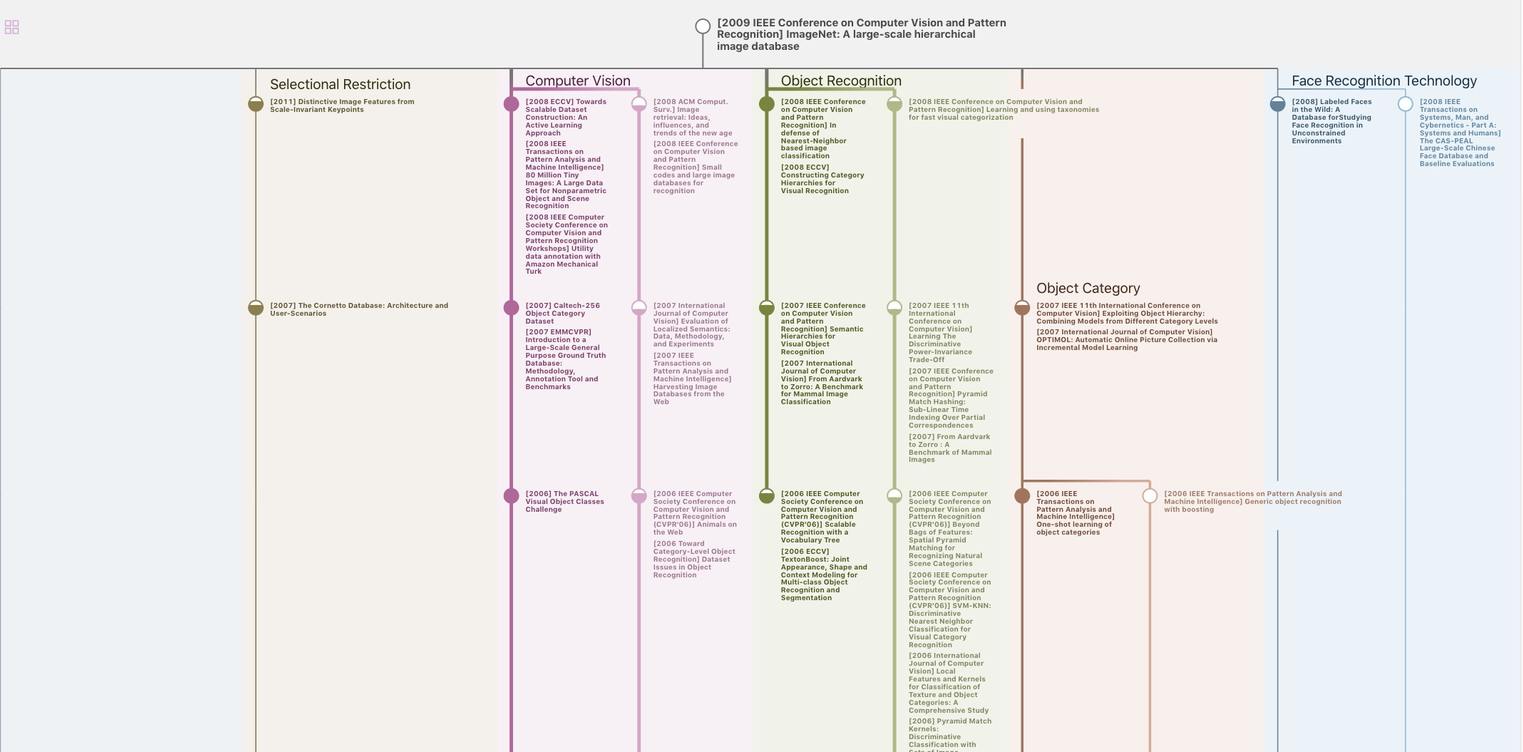
生成溯源树,研究论文发展脉络
Chat Paper
正在生成论文摘要