Adversarial Grammatical Error Generation: Application to Persian Language
International Journal on Natural Language Computing(2022)
摘要
Grammatical error correction (GEC) greatly benefits from large quantities of high-quality training data. However, the preparation of a large amount of labelled training data is time-consuming and prone to human errors. These issues have become major obstacles in training GEC systems. Recently, the performance of English GEC systems has drastically been enhanced by the application of deep neural networks that generate a large amount of synthetic data from limited samples. While GEC has extensively been studied in languages such as English and Chinese, no attempts have been made to generate synthetic data for improving Persian GEC systems. Given the substantial grammatical and semantic differences of the Persian language, in this paper, we propose a new deep learning framework to create large enough synthetic sentences that are grammatically incorrect for training Persian GEC systems. A modified version of sequence generative adversarial net with policy gradient is developed, in which the size of the model is scaled down and the hyperparameters are tuned. The generator is trained in an adversarial framework on a limited dataset of 8000 samples. Our proposed adversarial framework achieved bilingual evaluation understudy (BLEU) scores of 64.5% on BLEU-2, 44.2% on BLEU-3, and 21.4% on BLEU-4, and outperformed the conventional supervised-trained long short-term memory using maximum likelihood estimation and recently proposed sequence labeler using neural machine translation augmentation. This shows promise toward improving the performance of GEC systems by generating a large amount of training data.
更多查看译文
关键词
adversarial grammatical error generation,language
AI 理解论文
溯源树
样例
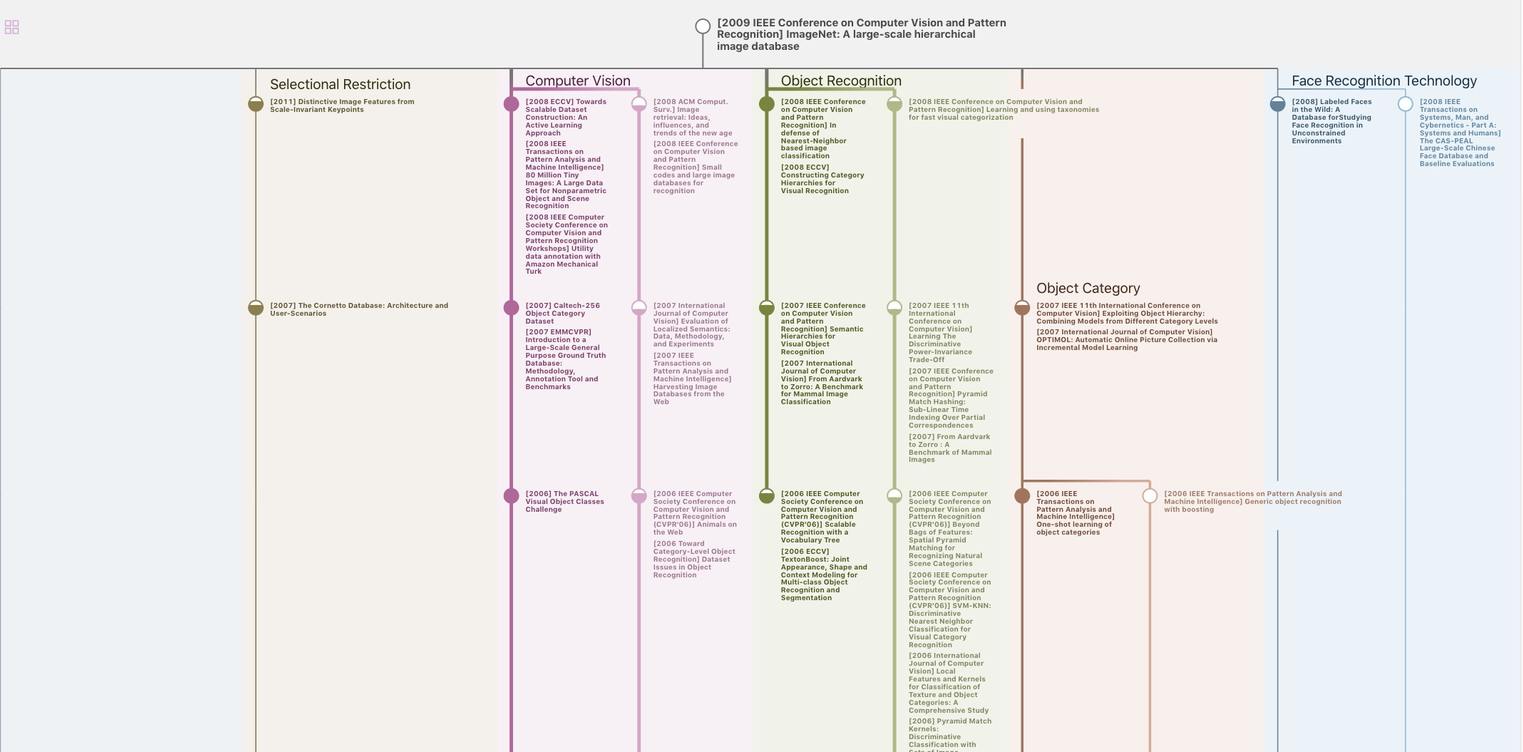
生成溯源树,研究论文发展脉络
Chat Paper
正在生成论文摘要