Normal and Abnormal Classification of Electrocardiogram: A Primary Screening Tool Kit.
Annual International Conference of the IEEE Engineering in Medicine and Biology Society (EMBC)(2022)
摘要
Cardiovascular diseases (CVDs) are one of the principal causes of death. Cardiac arrhythmia, a critical CVD, can be easily detected from an electrocardiogram (ECG) recording. Automated ECG analysis can help clinicians to identify arrhythmia and prevent untimely death. This paper presents a simple model to classify the ECG recordings into two classes: Normal and Abnormal based on morphological and heart rate variability (HRV) features. Before feature extraction, Signal quality analysis (SQA) is performed to abandon poor quality ECG signals. Several machine-learning classifiers such as Support Vector Machine (SVM), Adaboost (AB), Random Forest (RF), Extra-Tree Classifier (ET), Decision Tree (DT), Artificial Neural Network (ANN), K-Nearest Neighbors (KNN), Logistic Regression (LR), Naïve Bayes (NB), and Gradient Boosting (GB) are explored on the extracted feature space. To enhance the study, few feature selection algorithms such as F test, Least Absolute Shrinkage and Selection Operator (LASSO), and Minimal Redundancy Maximal Relevance (mRMR) algorithms are also applied and the outcomes of each algorithm along with the considered classifiers are analyzed and compared. The proposed algorithm is validated on 2648 Normal and 2518 Abnormal ECG recordings. The accuracy of our best classifier is found to be 95.25 %. It is anticipated that the proposed model will be helpful as a primary and mass screening tool kit in clinical settings.
更多查看译文
关键词
electrocardiogram,abnormal classification,screening
AI 理解论文
溯源树
样例
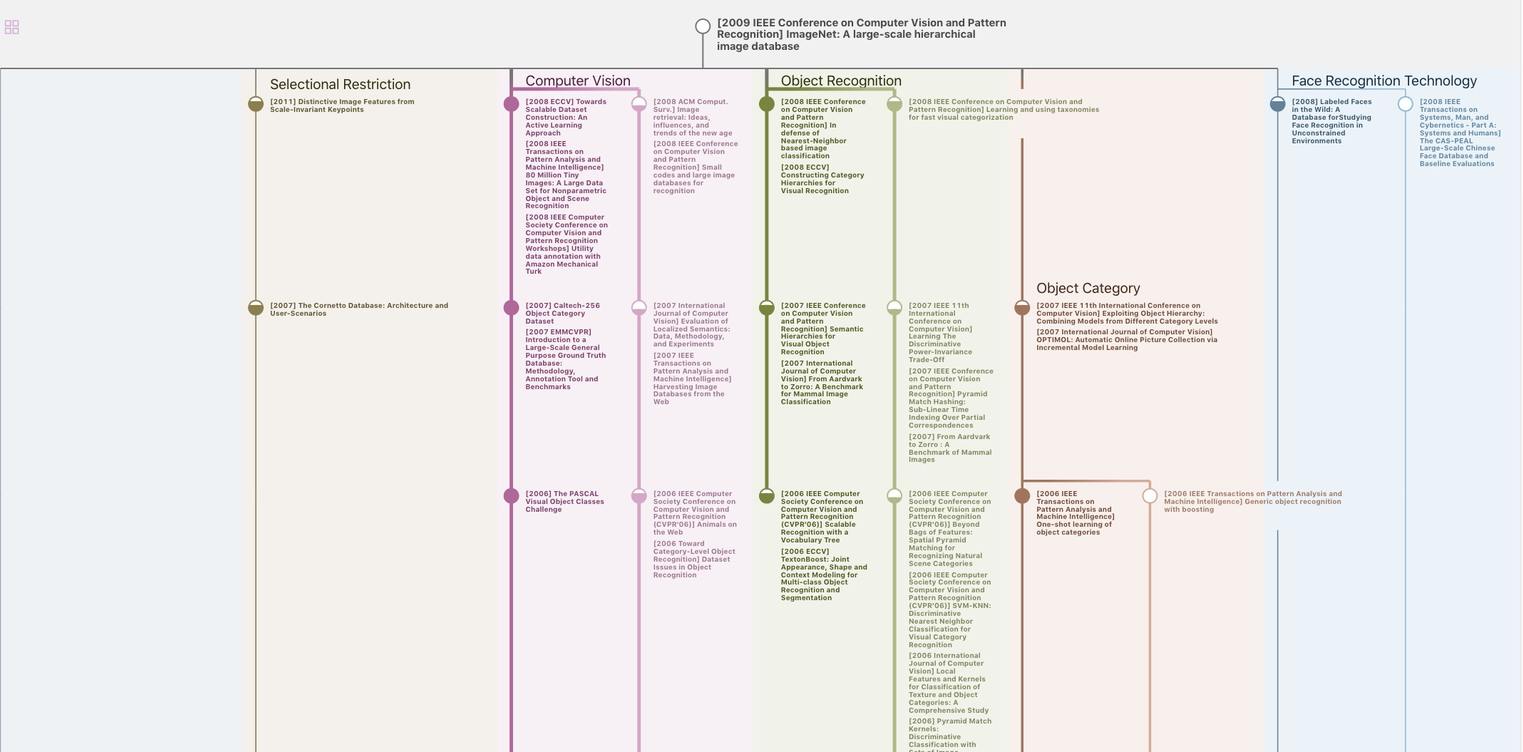
生成溯源树,研究论文发展脉络
Chat Paper
正在生成论文摘要