Deformable attention (DANet) for semantic image segmentation.
Annual International Conference of the IEEE Engineering in Medicine and Biology Society (EMBC)(2022)
Abstract
Deep learning based medical image segmentation is currently a widely researched topic. Attention mechanism used with deep networks significantly benefit semantic segmen-tation tasks. The recent criss-cross-attention module captures global self-attention while remaining memory and time efficient. However, capturing attention from only the pertinent non-local locations can cardinally boost the accuracy of semantic segmentation networks. We propose a new Deformable Attention Network (DANet) that enables a more accurate contextual information computation in a similarly efficient way. Our novel technique is based on learning the deformation of the query, key and value attention feature maps in a continuous way. A deep segmentation network with this attention mechanism is able to capture attention from germane non-local locations. This boosts the segmentation performance of COVID-19 lesion segmentation compared to criss-cross attention within aU-Net. Our validation experiments show that the performance gain of the recursively applied deformable attention blocks comes from their ability to capture dynamic and precise (wider) attention context. DANet achieves Dice scores of 60.17% for COVID-19 lesions segmentation and improves the accuracy by 4.4% points compared to a baseline U-Net.
MoreTranslated text
Key words
deformable attention,semantic image segmentation,danet
AI Read Science
Must-Reading Tree
Example
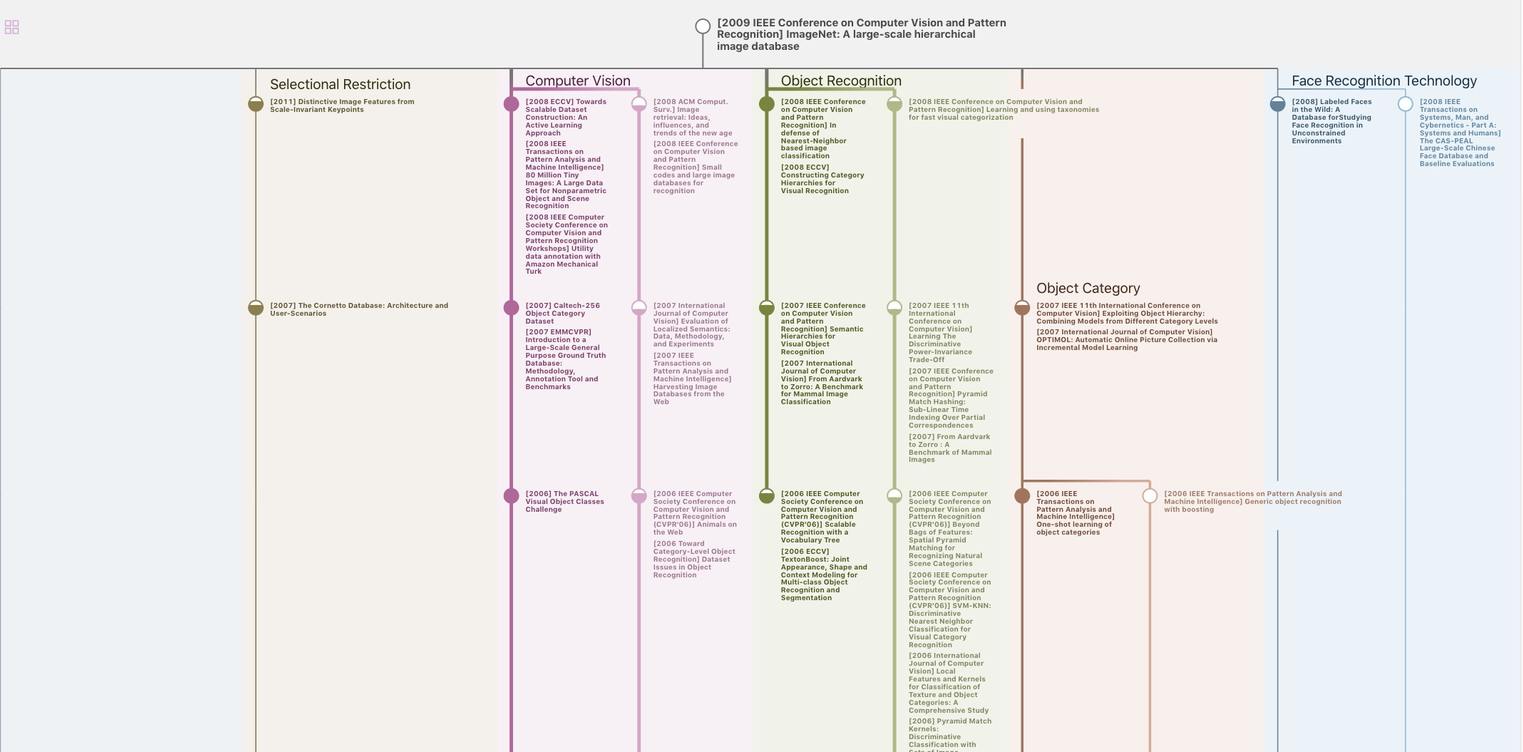
Generate MRT to find the research sequence of this paper
Chat Paper
Summary is being generated by the instructions you defined