Classification of Tonic Pain Experience based on Phase Connectivity in the Alpha Frequency Band of the Electroencephalogram using Convolutional Neural Networks.
Annual International Conference of the IEEE Engineering in Medicine and Biology Society (EMBC)(2022)
摘要
The complexity of brain activity involved in the generation of the experience of pain makes it hard to identify neural markers able to predict pain states. The within and between subjects variability of pain hinders the predictive potential of machine learning models trained across participants. This challenge can be tackled by implementing deep learning classifiers based on convolutional neural networks (CNNs). We targeted phase-based connectivity in the alpha band recorded with electroencephalography (EEG) during resting states and sensory conditions (eyes open [O] and closed [C] as resting states, and warm [W] and hot [H] water as sensory conditions). Connectivity features were extracted and re-organized as square matrices, because CNNs are effective in detecting the patterns from 2D data. To assess the classifier performance we implemented two complementary approaches: we 1) trained and tested the classifier with data from all participants, and 2) using a leave-one-out approach, that is excluding one participant at a time during training while using their data as a test set. The accuracy of binary classification between pain condition (H) and eyes open resting state (O) was 94.16% with the first approach, and 61.01 % with the leave-one-out approach. Clinical relevance-Further validation of the CNN classifier may help caregivers track the rehabilitation of chronic pain patients and dynamically modify the therapy. Further refinement of the model may allow its application in critical care setting with unresponsive patients to identify pain-like states otherwise incommunicable to medical personnel.
更多查看译文
关键词
Electroencephalography,Humans,Machine Learning,Neural Networks, Computer,Pain
AI 理解论文
溯源树
样例
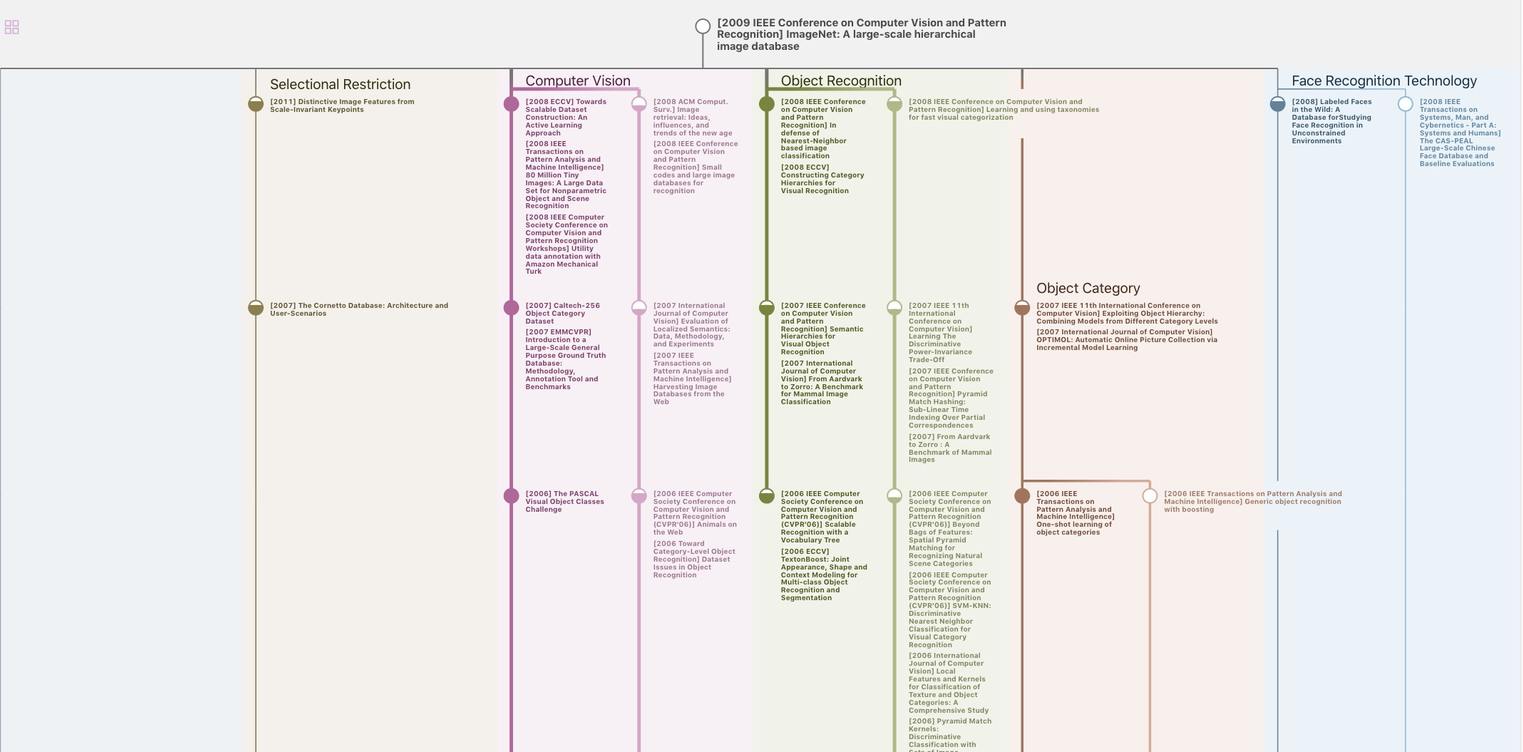
生成溯源树,研究论文发展脉络
Chat Paper
正在生成论文摘要