Estimating infant upper extremities motion with an RGB-D camera and markerless deep neural network tracking: A validation study.
Annual International Conference of the IEEE Engineering in Medicine and Biology Society (EMBC)(2022)
摘要
Quantitative biomarkers of infant motion may be predictive of the development of movement disorders. This study presents and validates a low cost, markerless motion tracking method for the estimation of upper body kinematics of infants from which proper biomarkers may be extracted. The method requires a single RGB-D camera, a 2D motion tracking software publicly available (DeepLabCut) and an algorithm generating 3D point coordinates from the 2D tracked points, dealing with missing data, originating from various sources, for estimating joint kinematics. The proposed method is validated using known point kinematics obtained from a doll, with size and shape of an infant, lying on a turntable rotating at 33⅓ rpm. Two camera image plane orientations are tested: parallel to the turntable motion plane and forming a 45° angle with respect to the motion plane. The latter enhances the occurrence of body parts occlusions during motion as expected in live infant motion recordings. The length of upper body segments, elbow and shoulder joint angles and the linear point velocity determined with the proposed method are evaluated against reference values obtained from the known motion of the turntable. The relevant Mean Absolute Errors (MAE) found indicate the margin of error to expect when processing live infant motion. The proposed method may be improved if enhanced hardware and tracking software are employed, therefore reducing the above-mentioned margin of error. Clinical Relevance - The validation of the proposed method carried out in this study allows clinicians to select proper quantitative biomarkers obtained from infants upper body motion that may be useful for predicting movement disorders.
更多查看译文
关键词
Algorithms,Humans,Infant,Margins of Excision,Motion,Movement Disorders,Neural Networks, Computer,Upper Extremity
AI 理解论文
溯源树
样例
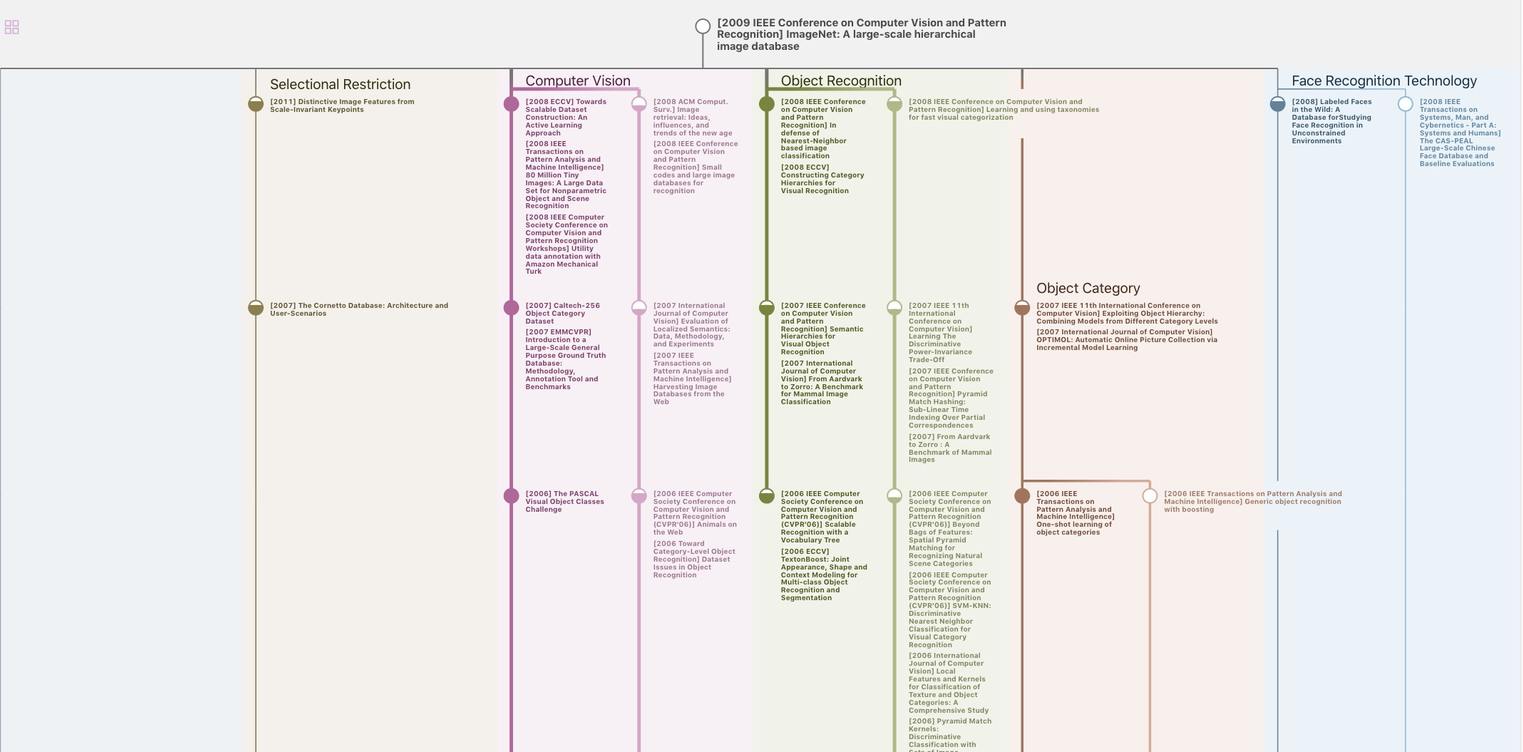
生成溯源树,研究论文发展脉络
Chat Paper
正在生成论文摘要