Chemical Space Localization for Unknown Metabolite Annotation via Semantic Similarity of Mass Spectral Language
crossref(2024)
Abstract
Untargeted metabolomics, employing liquid chromatography‒mass spectrometry (LC-MS), allows quantification of known and unidentified metabolites within biological systems. However, in practical metabolomic studies, the majority of metabolites often remain unidentified. Here, we developed a novel deep learning-based metabolite annotation approach via semantic similarity analysis of mass spectral language. This approach enables the prediction of structurally related metabolites for unknown compounds. By considering the chemical space, these structurally related metabolites provide valuable information about the potential location of the unknown metabolites and assist in ranking candidates obtained from molecular structure databases. Validated with benchmark datasets, our method has consistently demonstrated superior performance compared to existing methods for metabolite annotation. In a case study involving the Qianxi cultivar cherry tomato, our approach reaffirmed well-established biomarkers of ripening processes and identified a set of promising and rational new biomarker metabolites. Overall, presented method exhibits significant potential in annotating metabolites, particularly in revealing the "dark matter" in untargeted metabolomics.
MoreTranslated text
AI Read Science
Must-Reading Tree
Example
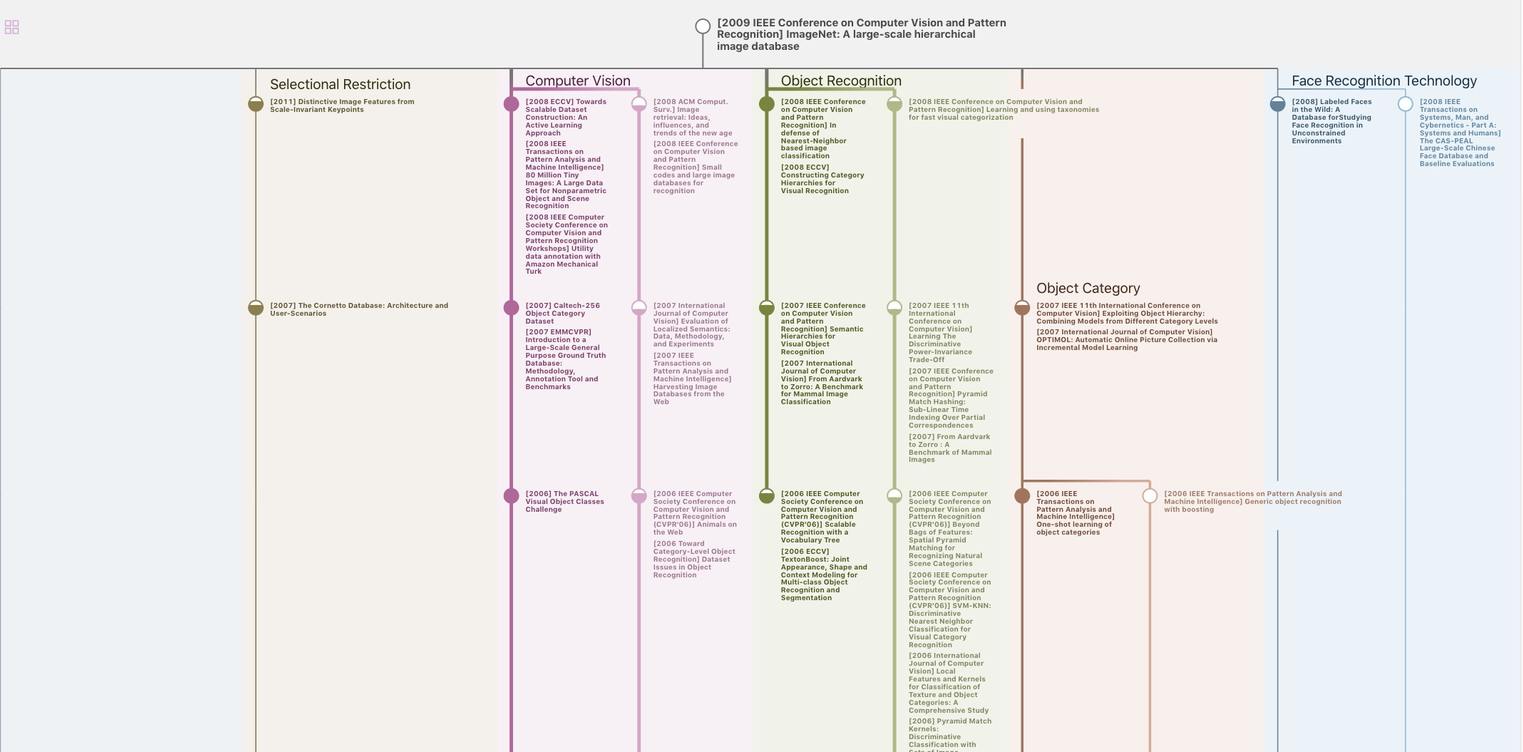
Generate MRT to find the research sequence of this paper
Chat Paper
Summary is being generated by the instructions you defined