Learning Graph Representation Based on Single Graph Frequent Pattern Mining
2022 8th International Conference on Big Data and Information Analytics (BigDIA)(2022)
摘要
Graph representation learning is crucial in a variety of real-life domains, and thus has received much attention from researchers. The traditional graph kernel-based method, while effective, has the problem of poor generalization ability because it relies on handcrafted features. Inspired by a neural embedding model for text (Doc2Vec), we propose an innovative approach named Fsg2Vec to learn graph representation. We obtain the unique features of each graph by extracting the frequent subgraphs in a specific single graph. Following the neural document embedding model, we use a graph instead of a document in the document embedding method, and replace words in the document with frequent subgraphs that are mined from the graph via a traditional pattern mining technique. There are mainly three points in our module superior to the latest graph embedding methods: completely unsupervised learning graph embedding, making the most of label information for nodes and edges, and mining the mutational characteristics of each graph for easy differentiation. Experimental data indicate our proposed module significantly outperforms baseline methods in graph classification task for small and medium-sized graphs.
更多查看译文
关键词
frequent sub graphs mining,representation learning,deep learning
AI 理解论文
溯源树
样例
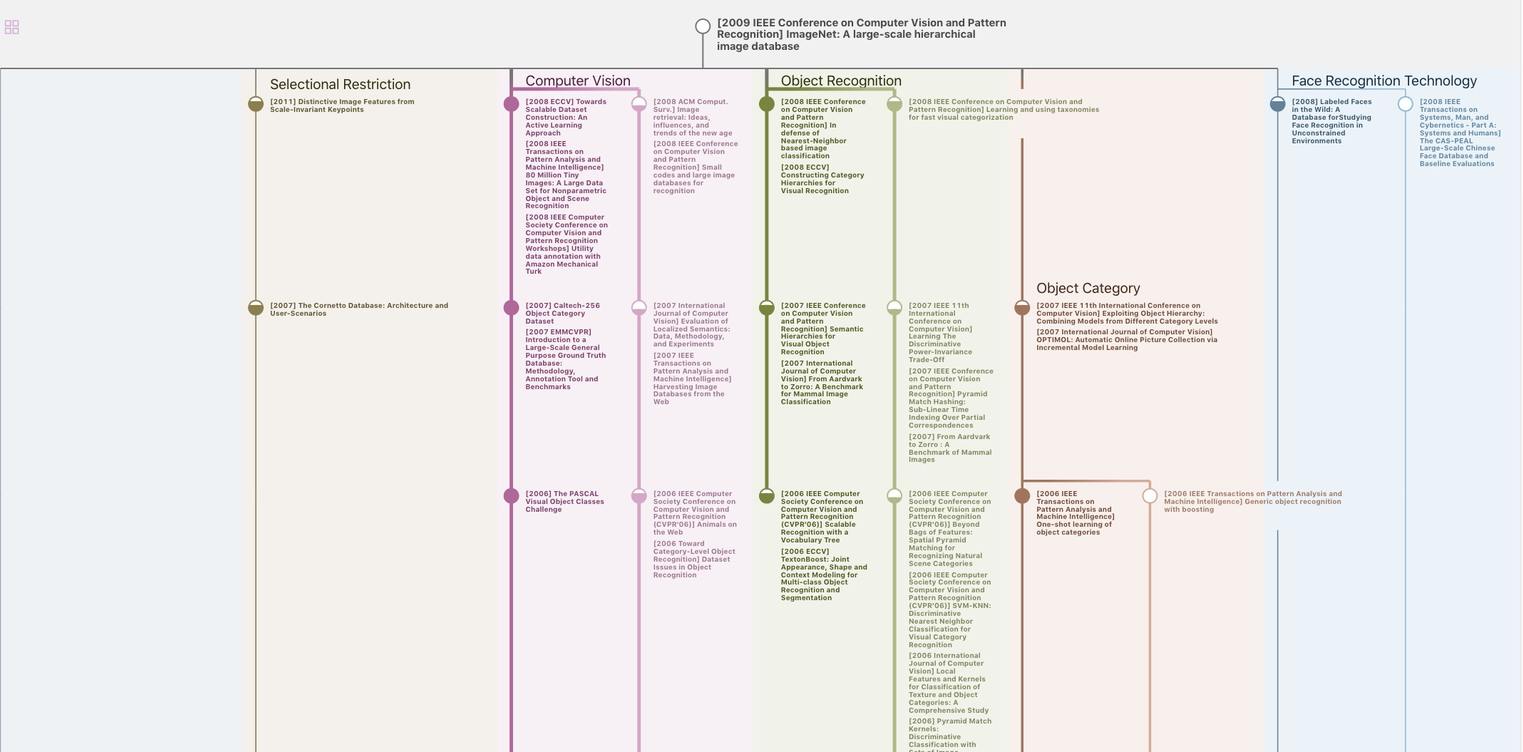
生成溯源树,研究论文发展脉络
Chat Paper
正在生成论文摘要