Pre-training and Transfer Learning for Training Set Reduction and Improving Automated Assessments of Clinical PET Image Quality
2021 IEEE Nuclear Science Symposium and Medical Imaging Conference (NSS/MIC)(2021)
摘要
Acquiring simultaneous positron emission tomography - magnetic resonance (PET-MR) images for memory clinic patients using a fraction of the usual injected dose can help reduce radiation dose concerns. However, this compromises the reconstructed image quality, thus determination of clinical usability is beneficial. A major challenge is the paucity of clinical quality readings. We hypothesise that exploiting easily available quantitative information or using established pre-trained networks to predict clinical assessments could reduce the number of clinically assessed datasets required for training convolutional neural networks (CNNs). In this study, a CNN was pre-trained to predict injected dose of patches extracted from six real patient datasets, reconstructed using 100% of the available data and different thinned datasets down to 0.5% of available data. Using transfer learning with five separate patients, the CNN was used to predict three clinically scored quality metrics: global quality rating. pattern recognition and diagnostic confidence, based on a four-point scale (0-3). This was compared to pre-training a VGG16 network with ImageNet at varying pre-training levels. This work shows test performance can be improved via pre-training compared to using no pre-training. Pre-training the last two convolutional blocks and fully connected layer of a VGG16 backbone achieves a 93 ± 3.7% broad agreement (predicted metric within 1 of clinician score) across all metrics, compared to 81 ± 2.6% when using no pre-training. Pre-training via dose inference achieves a maximum Pearson’s correlation coefficient of 0.90 for global quality rating, compared to 0.61 when using no pre-training. Easily accessible quantitative labels or pre-trained networks may be exploited to predict scarce clinical metrics. Future work will include increasing the clinical dataset number and applying the CNN to superior reconstruction algorithms.
更多查看译文
关键词
clinical pet image quality,transfer learning,pre-training set reduction,automated assessments
AI 理解论文
溯源树
样例
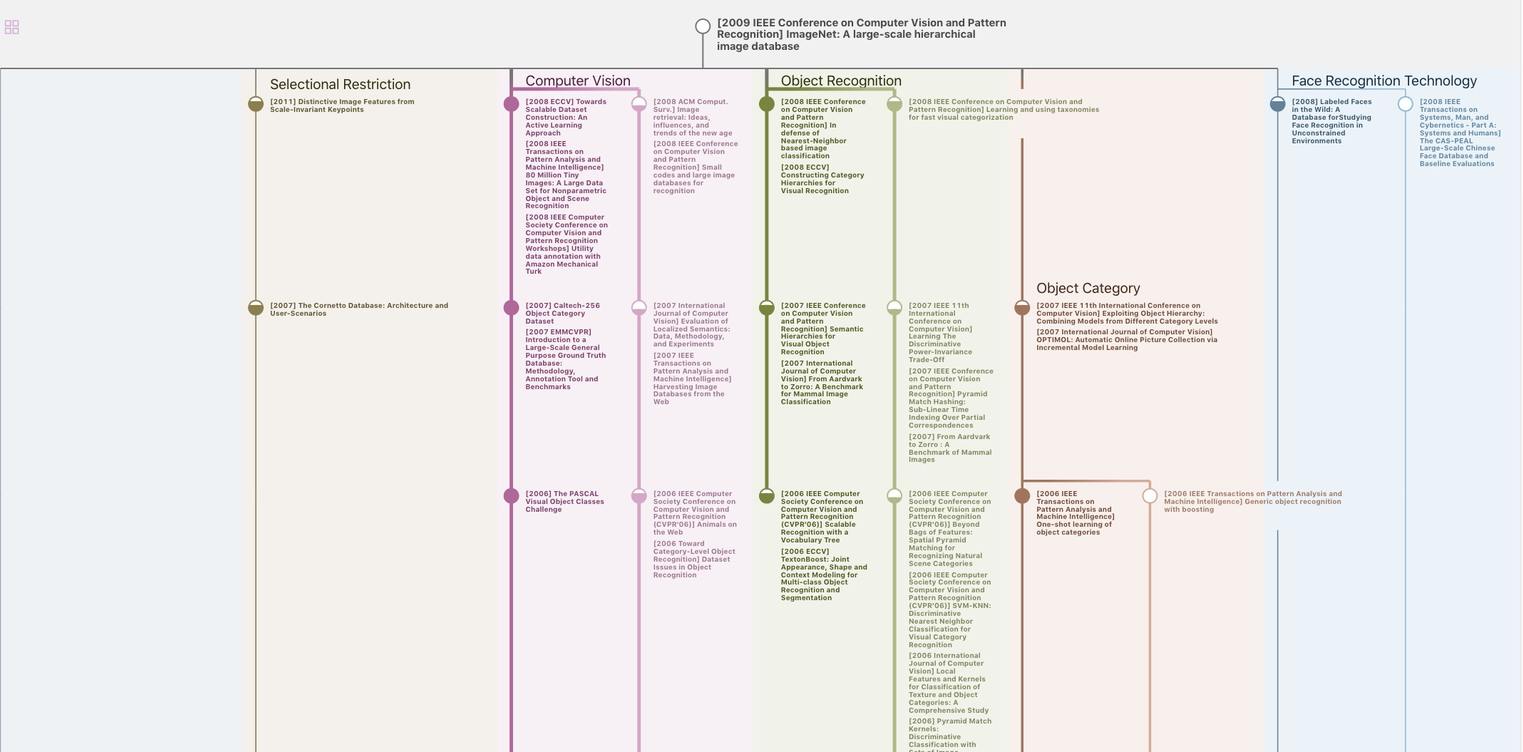
生成溯源树,研究论文发展脉络
Chat Paper
正在生成论文摘要