Application of a combined radiomics nomogram based on CE-CT in the preoperative prediction of thymomas risk categorization
FRONTIERS IN ONCOLOGY(2022)
摘要
ObjectiveThis study aimed to establish a combined radiomics nomogram to preoperatively predict the risk categorization of thymomas by using contrast-enhanced computed tomography (CE-CT) images. Materials and MethodsThe clinical, pathological, and CT data of 110 patients with thymoma (50 patients with low-risk thymomas and 60 patients with high-risk thymomas) collected in our Hospital from July 2017 to March 2022 were retrospectively analyzed. The study subjects were randomly divided into the training set (n = 77) and validation set (n = 33) in a 7:3 ratio. Radiomics features were extracted from the CT images, and the least absolute shrinkage and selection operator (LASSO) algorithm was performed to select 13 representative features. Five models, including logistic regression (LR), support vector machine (SVM), random forest (RF), decision tree (DT), and gradient boosting decision tree (GBDT) were constructed to predict thymoma risks based on these features. A combined radiomics nomogram was further established based on the clinical factors and radiomics scores. The performance of the models was evaluated using receiver operating characteristic (ROC) curve, DeLong tests, and decision curve analysis. ResultsMaximum tumor diameter and boundary were selected to build the clinical factors model. Thirteen features were acquired by LASSO algorithm screening as the optimal features for machine learning model construction. The LR model exhibited the highest AUC value (0.819) among the five machine learning models in the validation set. Furthermore, the radiomics nomogram combining the selected clinical variables and radiomics signature predicted the categorization of thymomas at different risks more effectively (the training set, AUC = 0.923; the validation set, AUC = 0.870). Finally, the calibration curve and DCA were utilized to confirm the clinical value of this combined radiomics nomogram. ConclusionWe demonstrated the clinical diagnostic value of machine learning models based on CT semantic features and the selected clinical variables, providing a non-invasive, appropriate, and accurate method for preoperative prediction of thymomas risk categorization.
更多查看译文
关键词
thymomas, risk categorization, contrast-enhanced computed tomography, radiomic nomogram, textural features, DeLong test, calibration curve, decision curve analysis
AI 理解论文
溯源树
样例
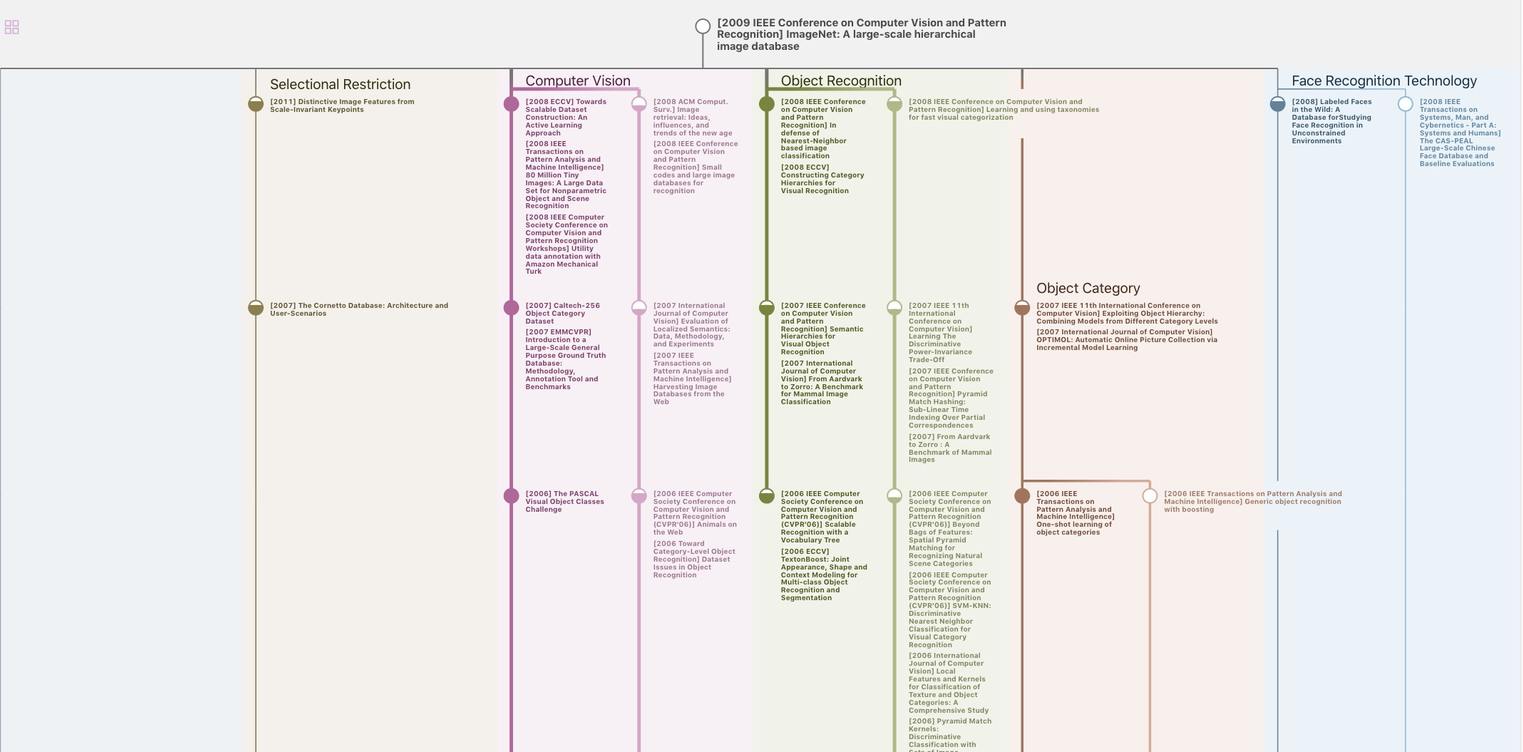
生成溯源树,研究论文发展脉络
Chat Paper
正在生成论文摘要