Domain-Adaptive Prototype-Recalibrated Network with Transductive Learning Paradigm for Intelligent Fault Diagnosis under Various Limited Data Conditions
SENSORS(2022)
摘要
In real industrial scenarios, intelligent fault diagnosis based on data-driven methods has been widely researched in the past decade. However, data scarcity is widespread in fault diagnosis tasks owning to the difficulties in collecting adequate data. As a result, there is an increasing demand for both researchers and engineers for fault identification with scarce data. To address this issue, an innovative domain-adaptive prototype-recalibrated network (DAPRN) based on a transductive learning paradigm and prototype recalibration strategy (PRS) is proposed, which has the potential to promote the generalization ability from the source domain to target domain in a few-shot fault diagnosis. Within this scheme, the DAPRN is composed of a feature extractor, a domain discriminator, and a label predictor. Concretely, the feature extractor is jointly optimized by the minimization of few-shot classification loss and the maximization of domain-discriminative loss. The cosine similarity-based label predictor, which is promoted by the PRS, is exploited to avoid the bias of naive prototypes in the metric space and recognize the health conditions of machinery in the meta-testing process. The efficacy and advantage of DAPRN are validated by extensive experiments on bearing and gearbox datasets compared with seven popular and well-established few-shot fault diagnosis methods. In practical application, the proposed DAPRN is expected to solve more challenging few-shot fault diagnosis scenarios and facilitate practical fault identification problems in modern manufacturing.
更多查看译文
关键词
intelligent fault diagnosis, transductive domain adaptation, prototype recalibration strategy, limited data conditions
AI 理解论文
溯源树
样例
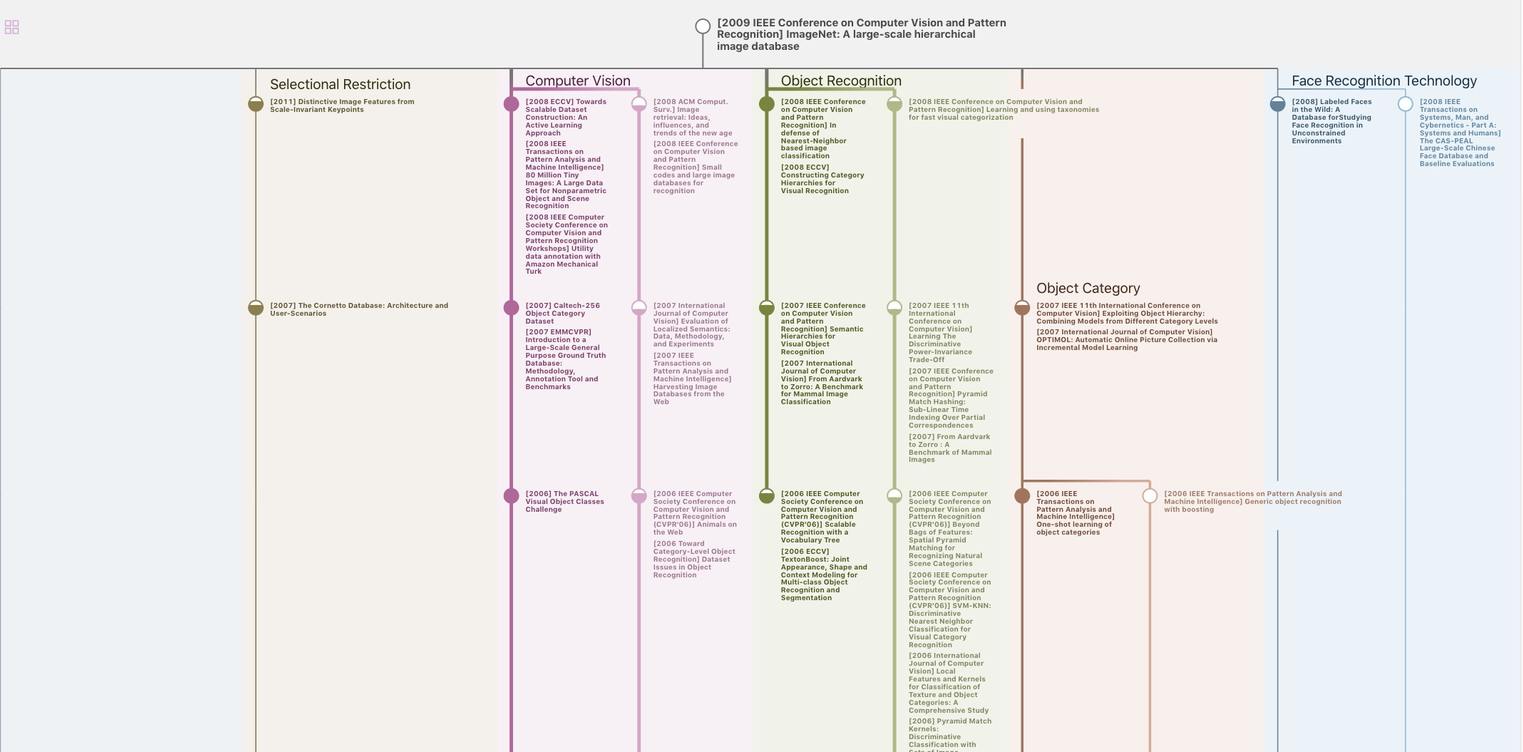
生成溯源树,研究论文发展脉络
Chat Paper
正在生成论文摘要