De Novo Prediction of Drug Targets and Candidates by Chemical Similarity-Guided Network-Based Inference
INTERNATIONAL JOURNAL OF MOLECULAR SCIENCES(2022)
摘要
Identifying drug-target interactions is a crucial step in discovering novel drugs and for drug repositioning. Network-based methods have shown great potential thanks to the straightforward integration of information from different sources and the possibility of extracting novel information from the graph topology. However, despite recent advances, there is still an urgent need for efficient and robust prediction methods. Here, we present SimSpread, a novel method that combines network-based inference with chemical similarity. This method employs a tripartite drug-drug-target network constructed from protein-ligand interaction annotations and drug-drug chemical similarity on which a resource-spreading algorithm predicts potential biological targets for both known or failed drugs and novel compounds. We describe small molecules as vectors of similarity indices to other compounds, thereby providing a flexible means to explore diverse molecular representations. We show that our proposed method achieves high prediction performance through multiple cross-validation and time-split validation procedures over a series of datasets. In addition, we demonstrate that our method performed a balanced exploration of both chemical ligand space (scaffold hopping) and biological target space (target hopping). Our results suggest robust and balanced performance, and our method may be useful for predicting drug targets, virtual screening, and drug repositioning.
更多查看译文
关键词
target prediction, drug-target interaction, network-based inference, drug repositioning, drug discovery
AI 理解论文
溯源树
样例
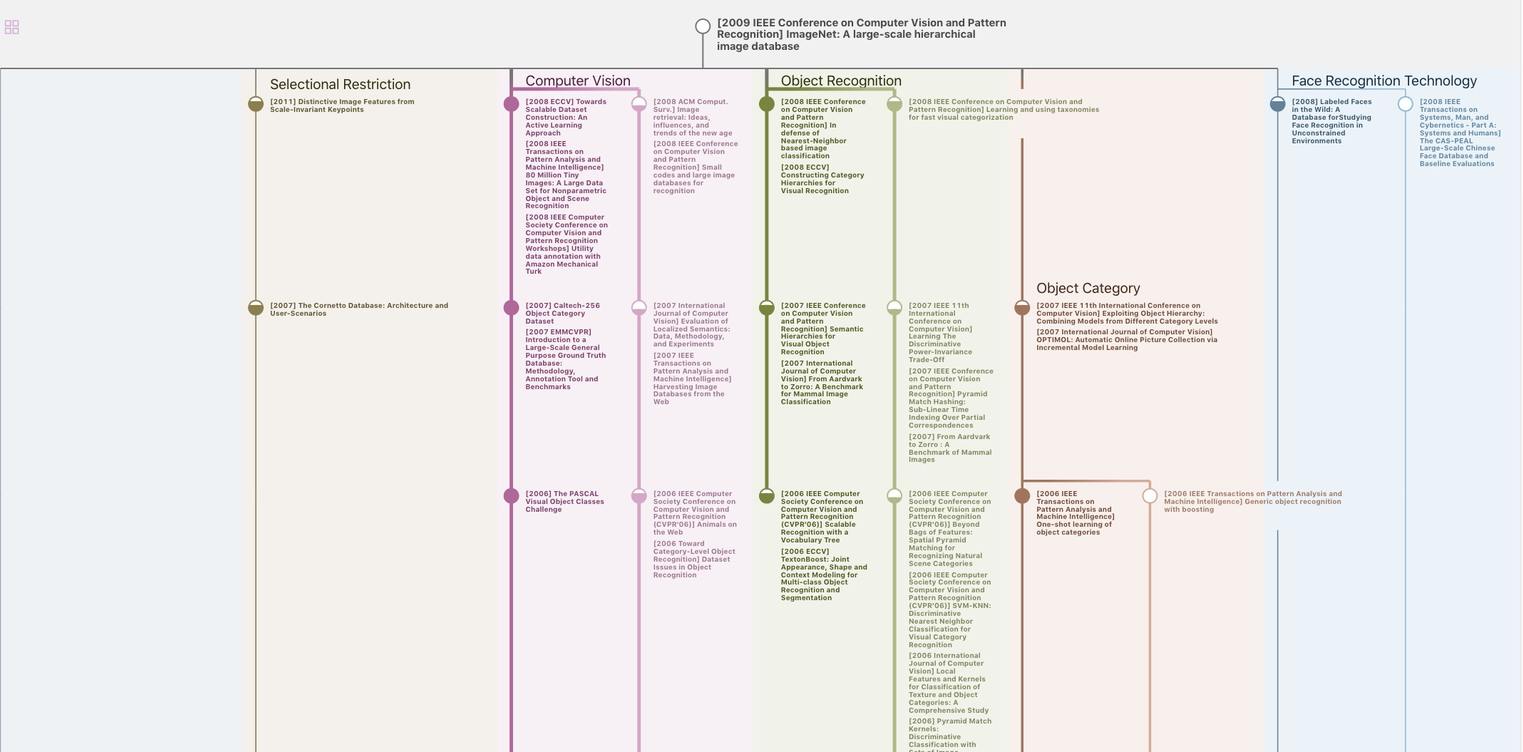
生成溯源树,研究论文发展脉络
Chat Paper
正在生成论文摘要