Reducing Scan Duration and Radiation Dose in Cerebral CT Perfusion Imaging Using a Recurrent Neural Network
2021 IEEE Nuclear Science Symposium and Medical Imaging Conference (NSS/MIC)(2021)
Abstract
Cerebral CT Perfusion (CTP) imaging is widely used for diagnosis and treatment decisions in acute stroke. The ability to reduce the scan duration is desirable but may lead to truncation of the contrast agent concentration measurements and inaccurate image-based stroke analysis. In this study, we present a novel application of a Stochastic Adversarial Video Prediction approach to reduce the acquisition time of CTP imaging. A variational autoencoder (VAE) and generative adversarial networks (GANs) VAE-GANs in a recurrent framework was implemented to predict the last 18 image frames (38 s) from the first 15 image frames (22 s). The model was trained on 65 CTP studies and tested on 10 studies. The results clearly show that prediction is possible. However, as expected, there was gradual degradation of average image quality metrics from early (measured) frames to late (predicted) frames: peak signal-to-noise ratio (PNSR) and structural similarity index (SSIM) decreased by 10.98 dB (from 47.48 dB to 36.5 dB) and 0.061 (from 0.997 to 0.936), respectively, and root mean squared error (RMSE) increased by 0.011 (from 0.004 to 0.015). Quantitative assessment of the hemodynamic maps showed that cerebral blood flow was easiest to predict and time-to-peak map was most difficult. The method potentially enables scan time and radiation dose to be reduced by 62% and 55%, respectively, but further investigation is needed to determine the impact on the quality of CTP images and resulting hemodynamic maps.
MoreTranslated text
Key words
cerebral ct perfusion imaging,scan duration,radiation dose
AI Read Science
Must-Reading Tree
Example
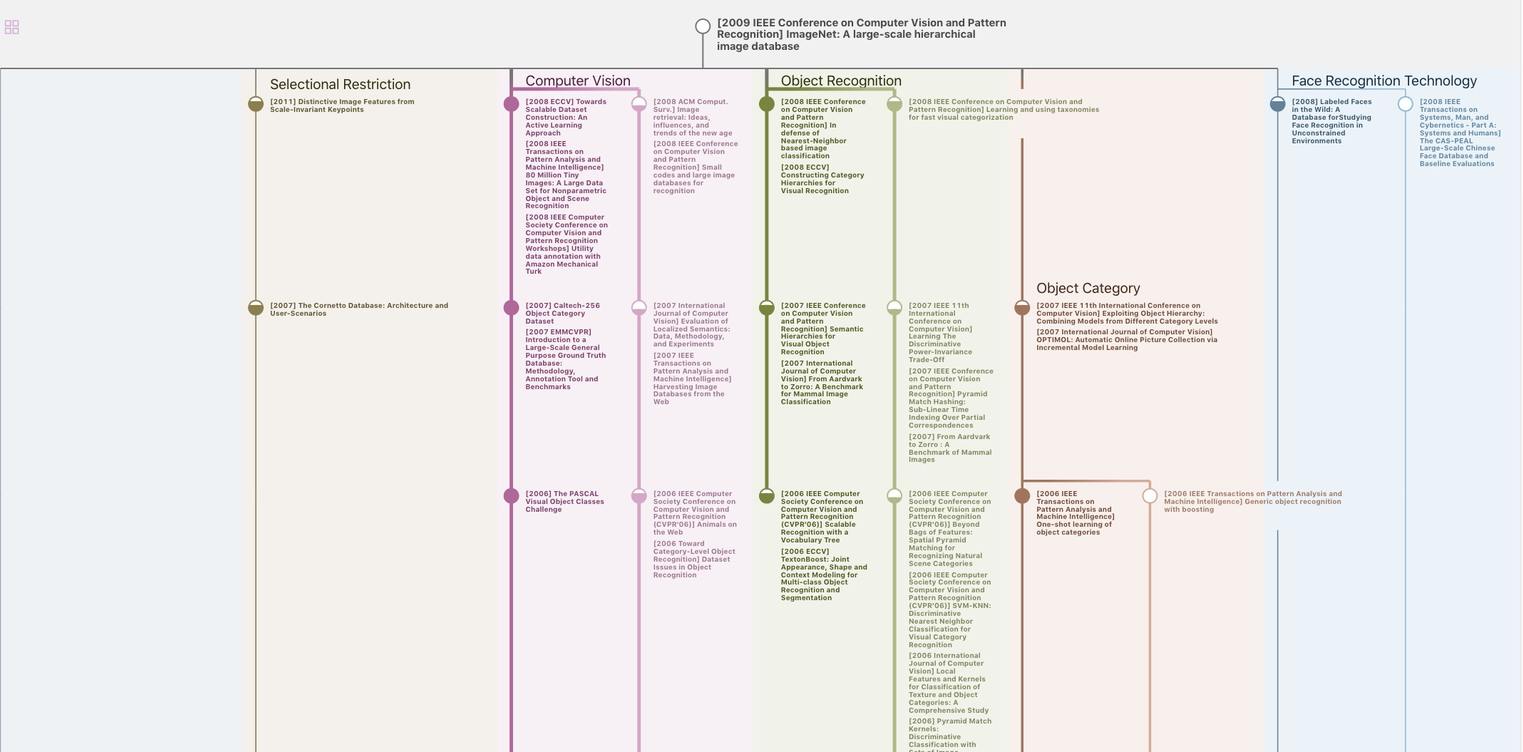
Generate MRT to find the research sequence of this paper
Chat Paper
Summary is being generated by the instructions you defined