Wildfire Path Prediction Spread using Machine Learning
2022 International Conference on Electrical, Computer and Energy Technologies (ICECET)(2022)
Abstract
The severity and frequency of wildfires are expected to worsen due to climate change, an increase in ignition sources and old lire management practices. Therefore, it is crucial to have models that can predict the fire spread to aid in wildfire management and disaster preparedness. This paper evaluates the feasibility of using Machine Learning (ML) techniques to model the fire spread dynamics and predict the wildfire spread from the ignition point to the surrounding areas. The ML models, Asynchronous Advantage Actor-Critic (A3C) and Long-term Recurrent Convolutional Network (LRCN) are compared for efficiency and correctness. These models were trained, tested, and validated using data from wildfire events. The models’ outputs depict the predicted burn maps indicating areas burned or not burned as an output in future time as the fire propagates from the ignition point to the surrounding areas. The burn boundary similarity (BBS) and burn area ratio (BAR) validation techniques are recommended to validate the models’ performances. Based on the analysis of the ML models considered, the LRCN model is expected to be better as it includes spatial and temporal properties when modelling wildfire spread.
MoreTranslated text
Key words
Machine Learning,Wildfire spread,Satellite Imagery,Deep Learning,Reinforcement Learning,A3C,LRCN
AI Read Science
Must-Reading Tree
Example
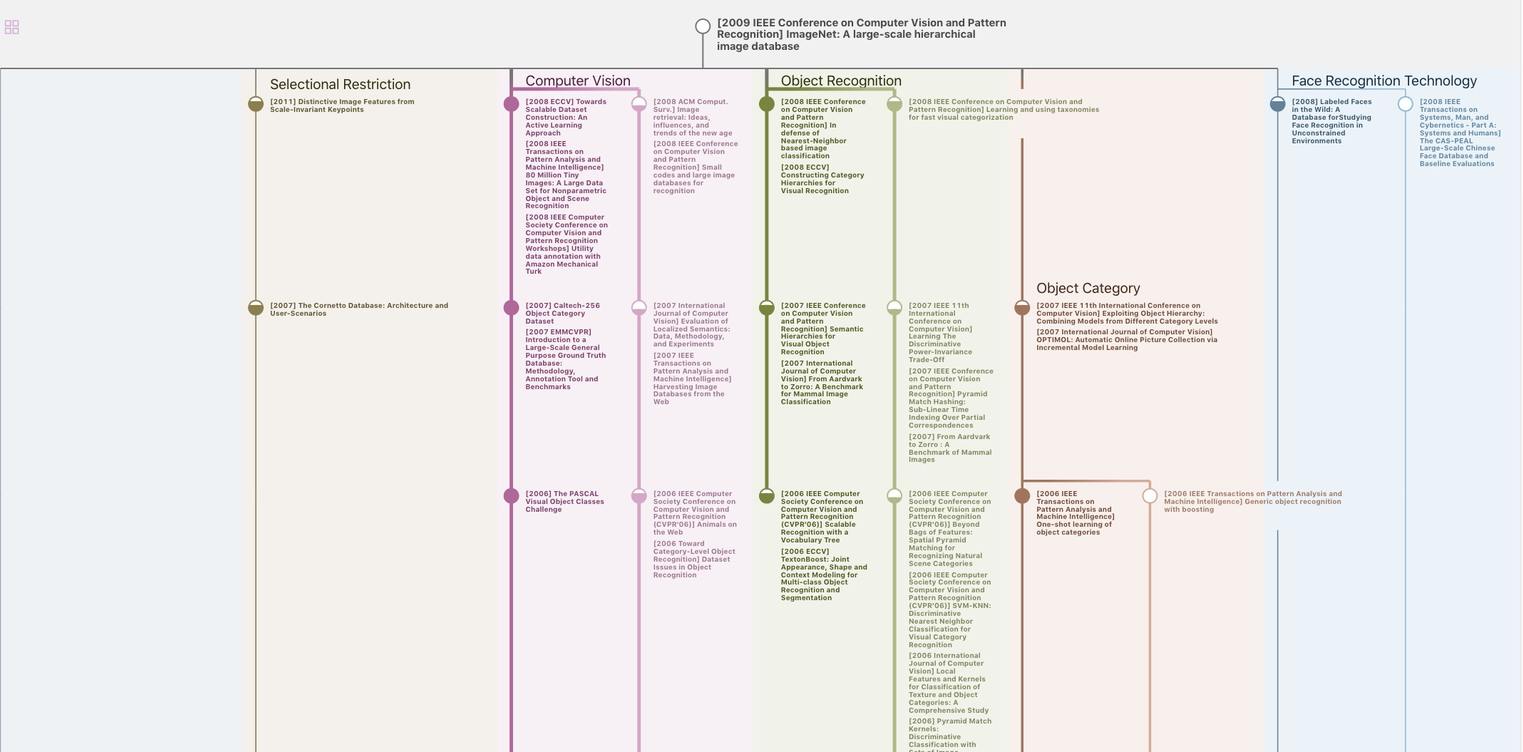
Generate MRT to find the research sequence of this paper
Chat Paper
Summary is being generated by the instructions you defined