Improving penalized semi supervised nonnegative matrix factorization result’s confidence using deep residual learning approach in spectrum analysis
2022 International Conference on Electrical, Computer and Energy Technologies (ICECET)(2022)
摘要
Signa1 denoising is one of the most crucial tasks to progress in today’s main issues including edge Tokamak plasmas’ atomic spectra and astrophysical data analyses. In the study of radiative spectra, noise subtraction is the key to significantly enhance methods developed to identify spectra’s prominent constituent elements. One of these methods is the penalized semi supervised nonnegative matrix factorization (PSNMF): it extracts the spectra’s prominent elements successfully, but its results’ confidence are sensitive to noise and can ultimately hurt the method’s performance. On the other hand, artificial intelligence (AI) shows very good ability to disentangle complex inputs and allows image unblurring, pattern recognition and so on. The work presented here aims to use a specific 1D convolutional neural network in the residual learning approach to identify and subtract the noise from noisy spectra. This framework improves the confidence of the PSNMF output without hurting its already good recognition performance. For the most noisy data considered, we can infer the good element set with a confidence close to the noiseless case.
更多查看译文
关键词
NMF,atomic spectra,denoising,convolutional neural network,residual learning
AI 理解论文
溯源树
样例
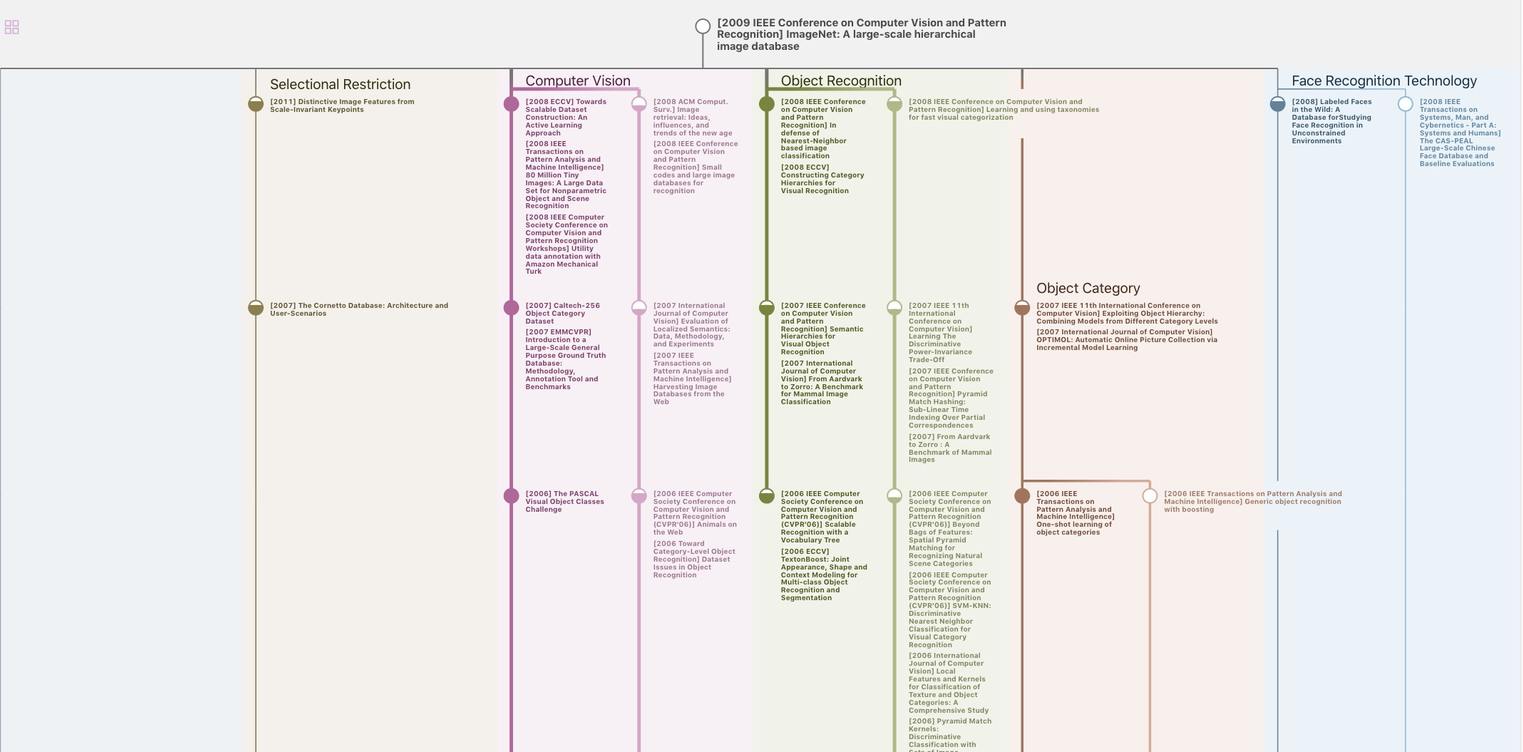
生成溯源树,研究论文发展脉络
Chat Paper
正在生成论文摘要