Energy-Efficient Recurrent Neural Network With MRAM-Based Probabilistic Activation Functions
IEEE Transactions on Emerging Topics in Computing(2023)
摘要
Herein, we develop a programmable energy-efficient hardware implementation for Recurrent Neural Networks (RNNs) with Resistive Random-Access Memory (ReRAM) synapses and ultra-low power, area-efficient spin-based activation functions. To attain high energy-efficiency while maintaining accuracy, a novel Computing-in-Memory (CiM) architecture is proposed to leverage data-level parallelism during the evaluation phase. We employ an MRAM-based Adjustable Probabilistic Activation Function (APAF) via a low-power tunable activation mechanism, providing adjustable levels of accuracy to mimic ideal sigmoid and tanh thresholding along with a matching algorithm to regulate the neuron properties. Our hardware/software cross-layer simulation shows that our proposed design achieves up to 74.5x energy-efficiency with similar to 11x area reduction compared to its counterpart designs while keeping the accuracy comparable.
更多查看译文
关键词
Neurons,Recurrent neural networks,Computer architecture,Behavioral sciences,Probabilistic logic,Synapses,Energy efficiency,Binary stochastic neuron,recurrent neural networks,computing-in-memory,spintronics
AI 理解论文
溯源树
样例
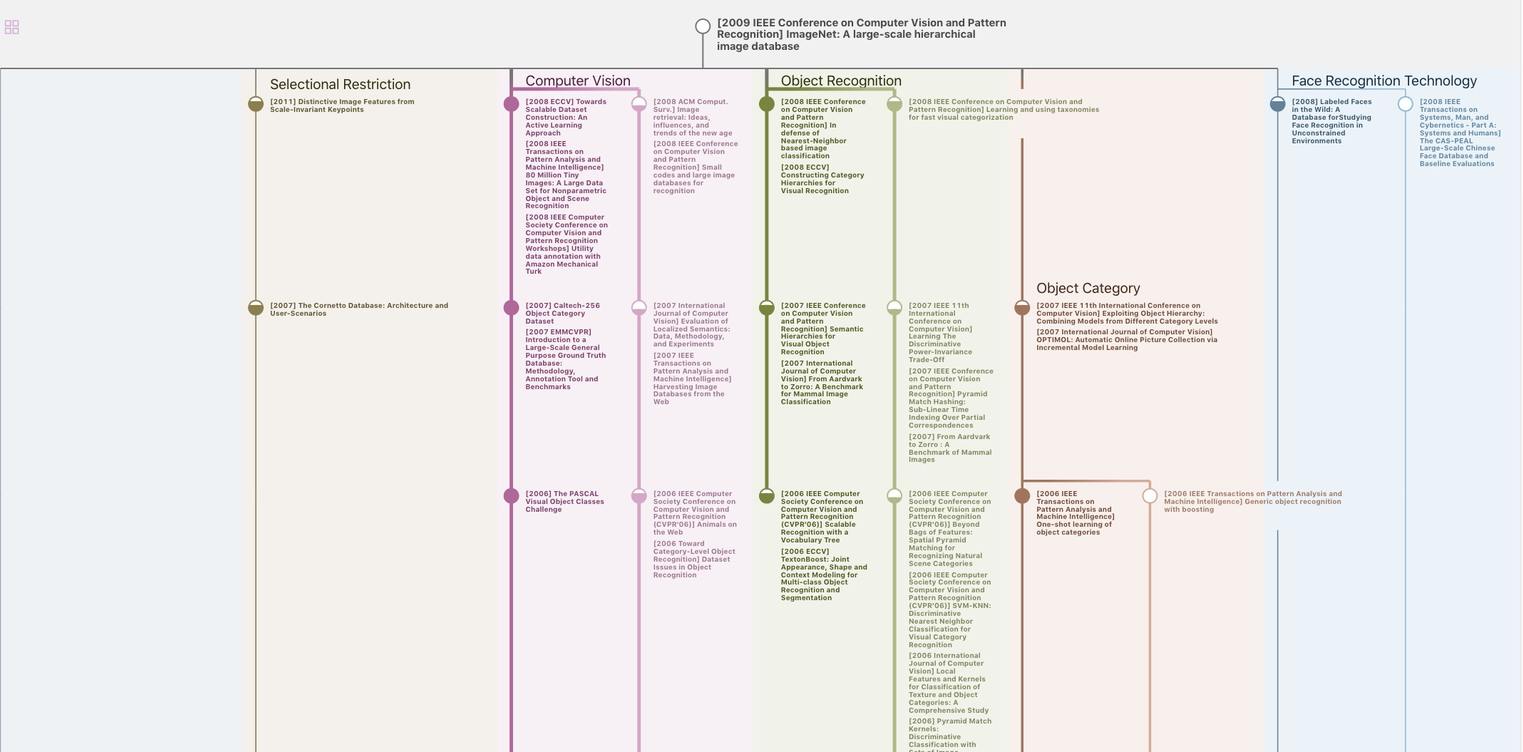
生成溯源树,研究论文发展脉络
Chat Paper
正在生成论文摘要