Beyond Classifiers: Remote Sensing Change Detection with Metric Learning
REMOTE SENSING(2022)
摘要
For change detection in remote sensing images, supervised learning always relies on bi-temporal images as inputs and 2-class/multi-class classifiers as outputs. On the other hand, change detection can be viewed as a metric learning problem, i.e., changed areas should be dissimilar while unchanged areas should be similar. In this paper, we study several metric learning formulations for change detection. A strong baseline is achieved by training on pair-wise images with Reverted Contrastive Loss (RCL) with hard mining. Motivated by the success of triplet loss, we seek two sources of triplet pairs from the bi-temporal images, and a novel Spatial-Temporal Triplet Loss (STTL) is proposed. The proposed triplet loss is further validated on semantic change detection, where semantic labels are provided for the changed areas. The experimental results prove state-of-the-art performance on both binary and semantic change detection.
更多查看译文
关键词
change detection,metric learning,triplet loss
AI 理解论文
溯源树
样例
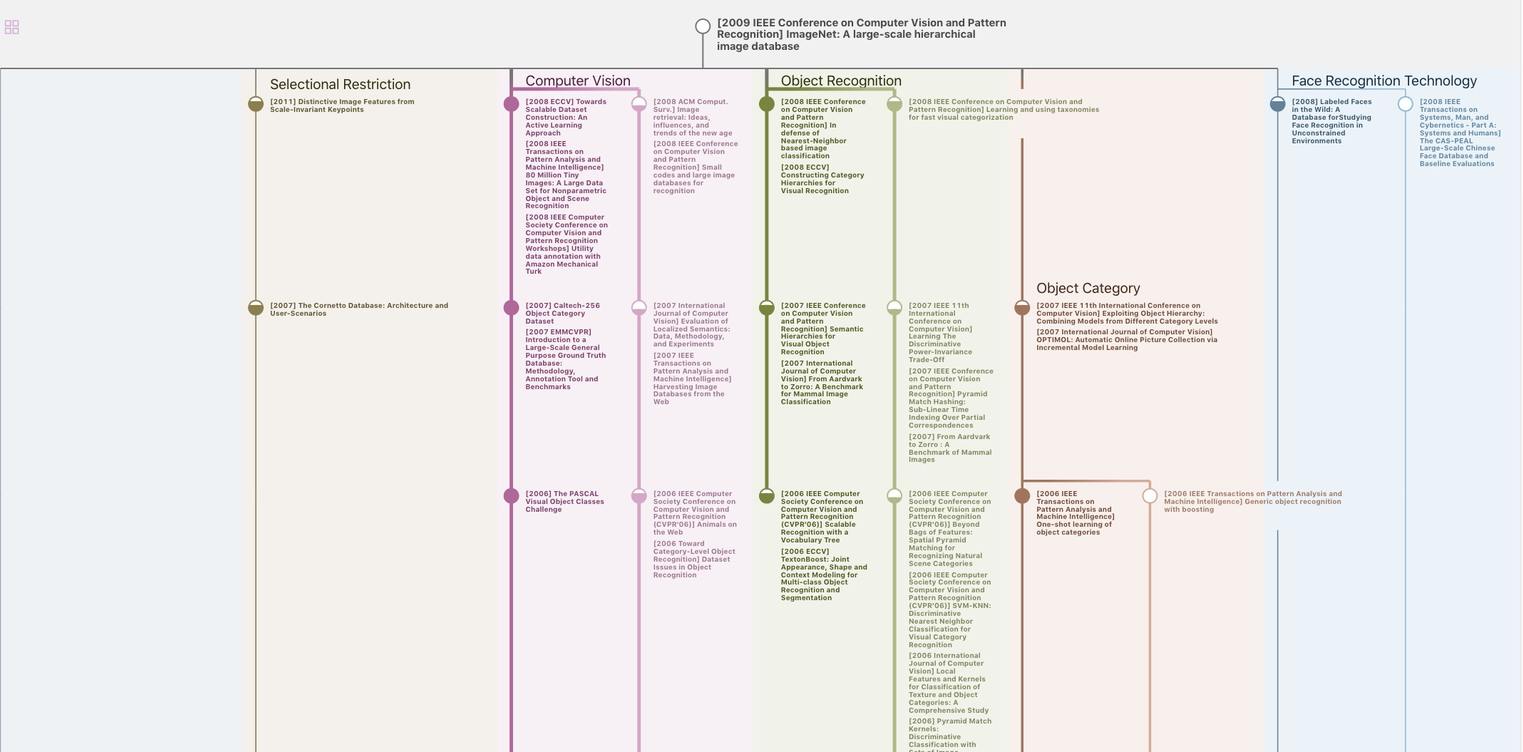
生成溯源树,研究论文发展脉络
Chat Paper
正在生成论文摘要