MV-GPRNet: Multi-View Subsurface Defect Detection Network for Airport Runway Inspection Based on GPR
REMOTE SENSING(2022)
摘要
The detection and restoration of subsurface defects are essential for ensuring the structural reliability of airport runways. Subsurface inspections can be performed with the aid of a robot equipped with a Ground Penetrating Radar (GPR). However, interpreting GPR data is extremely difficult, as GPR data usually contains severe clutter interference. In addition, many different types of subsurface defects present similar features in B-scan images, making them difficult to distinguish. Consequently, this makes later maintenance work harder as different subsurface defects require different restoration measures. Thus, to automate the inspection process and improve defect identification accuracy, a novel deep learning algorithm, MV-GPRNet, is proposed. Instead of traditionally using GPR B-scan images only, MV-GPRNet utilizes multi-view GPR data to robustly detect regions with defects despite significant interference. It originally fuses the 3D feature map in C-scan data and the 2D feature map in Top-scan data for defect classification and localization. With our runway inspection robot, a large number of real runway data sets from three international airports have been used to extensively test our method. Experimental results indicate that the proposed MV-GPRNet outperforms state-of-the-art (SOTA) approaches. In particular, MV-GPRNet achieves F1 measurements for voids, cracks, subsidences, and pipes at 91%, 69%, 90%, and 100%, respectively.
更多查看译文
关键词
GPR,subsurface defect detection,multi-view CNN model,airport runway inspection
AI 理解论文
溯源树
样例
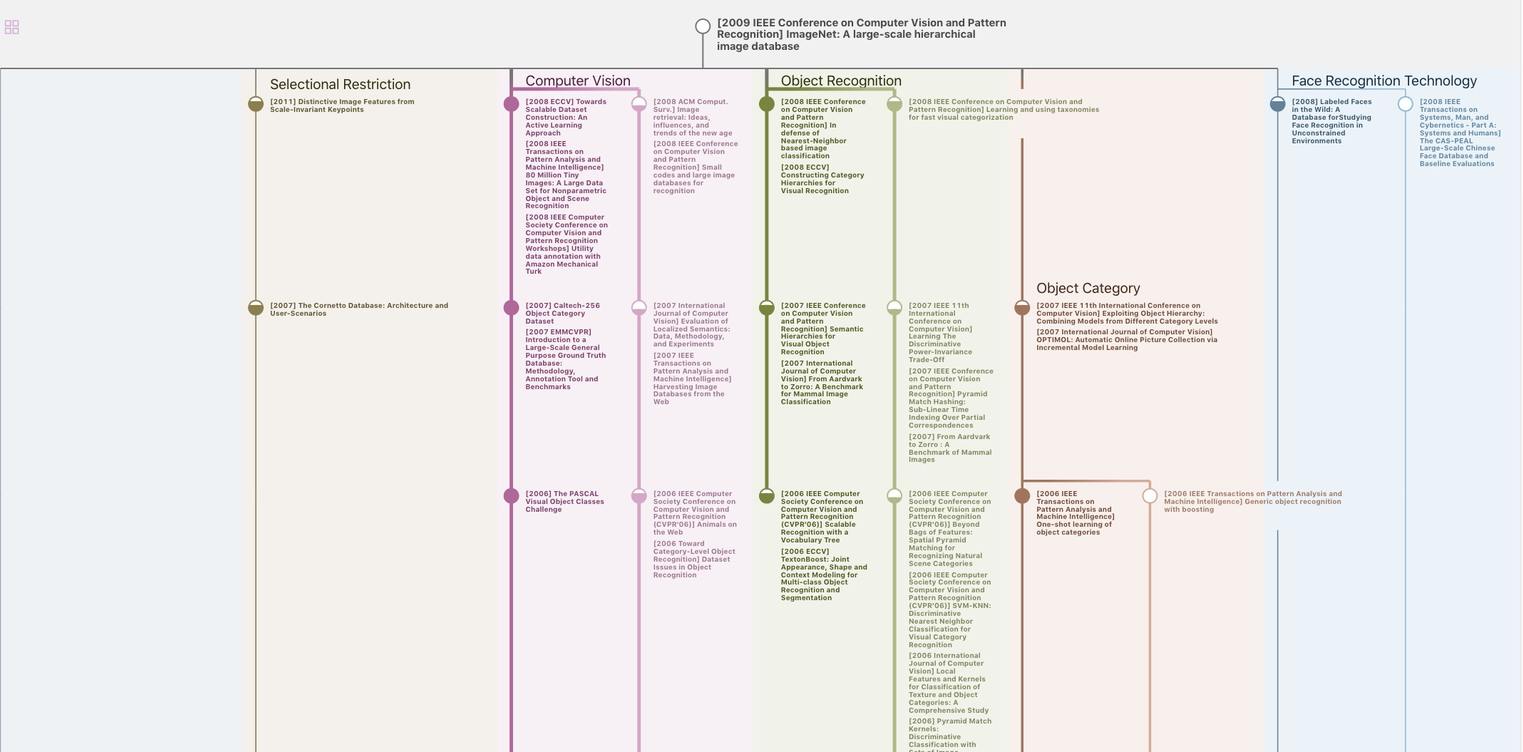
生成溯源树,研究论文发展脉络
Chat Paper
正在生成论文摘要