Increasing Trust for Data Spaces with Federated Learning
Data Spaces(2022)
摘要
AbstractDespite the need for data in a time of general digitization of organizations, many challenges are still hampering its shared use. Technical, organizational, legal, and commercial issues remain to leverage data satisfactorily, specially when the data is distributed among different locations and confidentiality must be preserved. Data platforms can offer “ad hoc” solutions to tackle specific matters within a data space. MUSKETEER develops an Industrial Data Platform (IDP) including algorithms for federated and privacy-preserving machine learning techniques on a distributed setup, detection and mitigation of adversarial attacks, and a rewarding model capable of monetizing datasets according to the real data value. The platform can offer an adequate response for organizations in demand of high security standards such as industrial companies with sensitive data or hospitals with personal data. From the architectural point of view, trust is enforced in such a way that data has never to leave out its provider’s premises, thanks to federated learning. This approach can help to better comply with the European regulation as confirmed from a legal perspective. Besides, MUSKETEER explores several rewarding models based on the availability of objective and quantitative data value estimations, which further increases the trust of the participants in the data space as a whole.
更多查看译文
关键词
federated learning,trust,data spaces
AI 理解论文
溯源树
样例
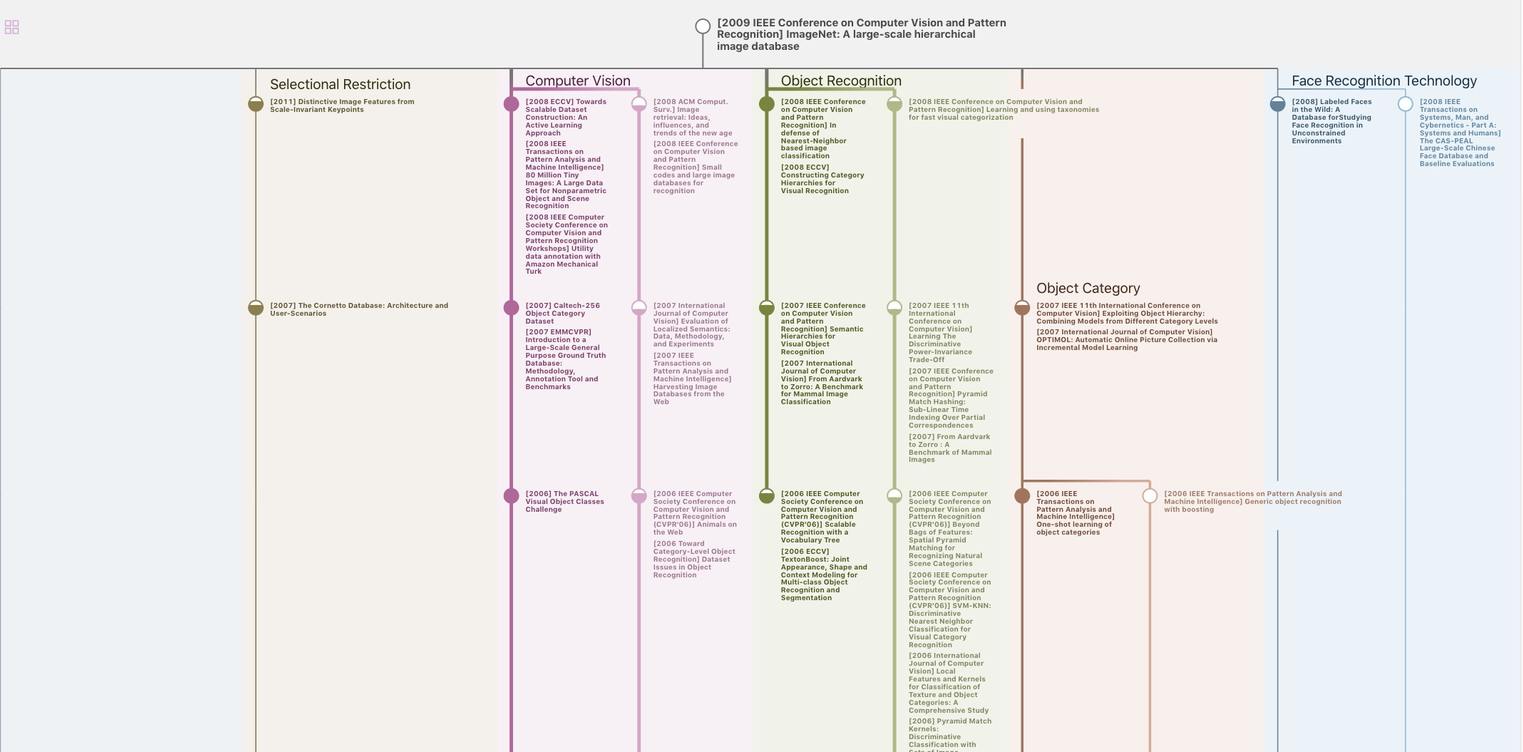
生成溯源树,研究论文发展脉络
Chat Paper
正在生成论文摘要