Risk of Bias in Chest X-ray Foundation Models
CoRR(2022)
摘要
Foundation models are considered a breakthrough in all applications of AI, promising robust and reusable mechanisms for feature extraction, alleviating the need for large amounts of high quality annotated training data for task-specific prediction models. However, foundation models may potentially encode and even reinforce existing biases present in historic datasets. Given the limited ability to scrutinize foundation models, it remains unclear whether the opportunities outweigh the risks in safety critical applications such as clinical decision making. In our statistical bias analysis of a recently published, and publicly accessible chest X-ray foundation model, we found reasons for concern as the model seems to encode protected characteristics including biological sex and racial identity. When used for the downstream application of disease detection, we observed substantial degradation of performance of the foundation model compared to a standard model with specific disparities in protected subgroups. While research into foundation models for healthcare applications is in an early stage, we hope to raise awareness of the risks by highlighting the importance of conducting thorough bias and subgroup performance analyses.
更多查看译文
AI 理解论文
溯源树
样例
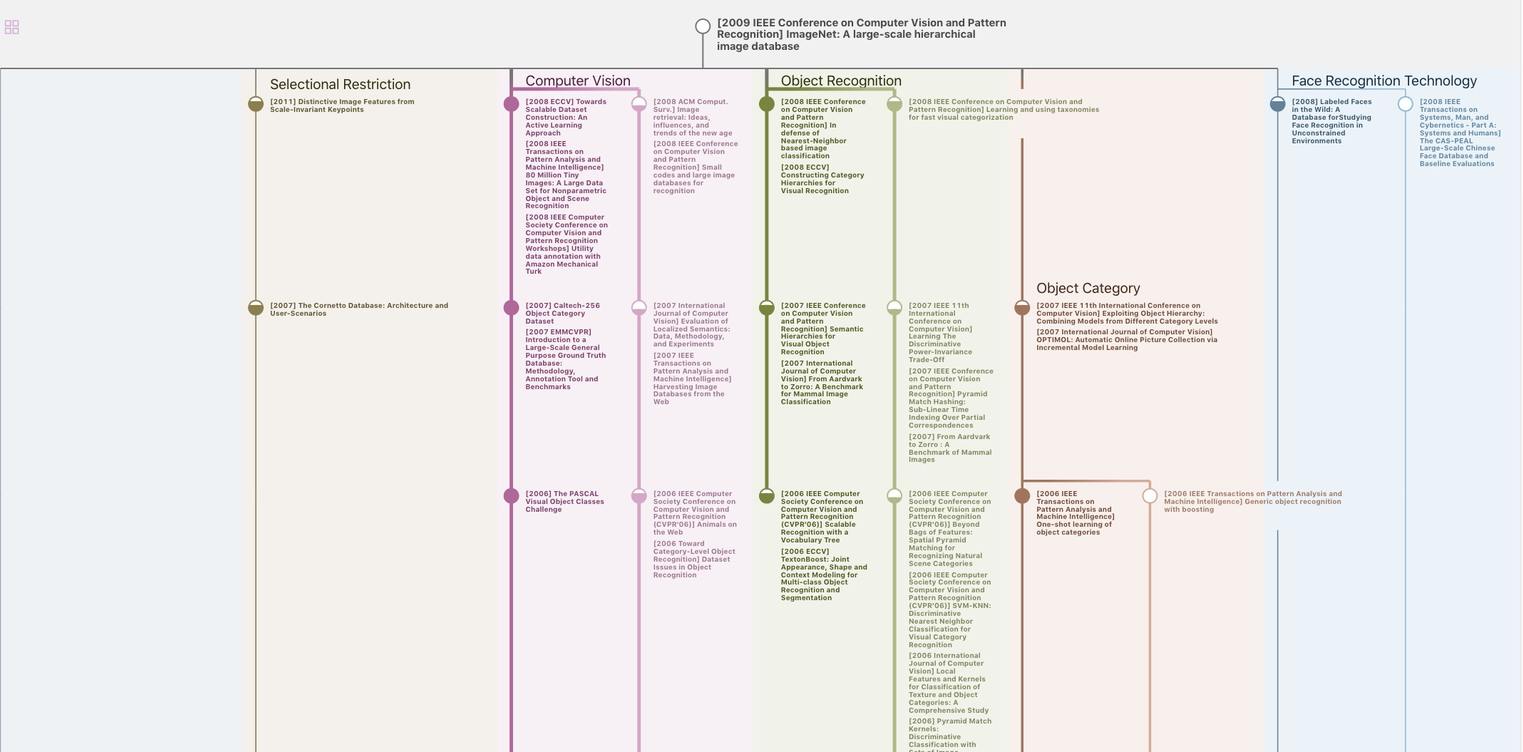
生成溯源树,研究论文发展脉络
Chat Paper
正在生成论文摘要