Multi-Aspect Deep Active Attention Network for Healthcare Explainable Adoption
IEEE journal of biomedical and health informatics(2023)
Abstract
Depression is a serious illness that significantly affects the lives of those affected. Recent studies have looked at the possibility of detecting and diagnosing this mental disorder using user-generated data from various forms of online media. Therefore, we address the issue of detecting sadness in social media by focusing on terms in personal remarks. To overcome the limitations in classifying depression texts, this study aims to develop attention networks that use covert levels of self-attention. Since nodes/words can express properties/emotions of their neighbors, this paper naturally assigns each node in a neighborhood a weight without performing costly matrix operations such as similarity or network architecture knowledge. This paper extends the emotion lexicon by using hypernyms. For this reason, our method is superior to the performance of other designs. According to the results of our experiments, the emotion lexicon combined with an attention network achieves an ROC of 0.87 while maintaining its interpretability and transparency level. Subsequently, the learned embedding is used to display the contribution of each symptom to the activated word, and the psychiatrist is polled to obtain his qualitative agreement with this representation. By using unlabeled forum language, the method increases the rate at which depression symptoms can be identified from information in Internet forums.
MoreTranslated text
Key words
Internet-delivered interventions,explain- ability,natural language processing,adaptive treatments,word sense identification
AI Read Science
Must-Reading Tree
Example
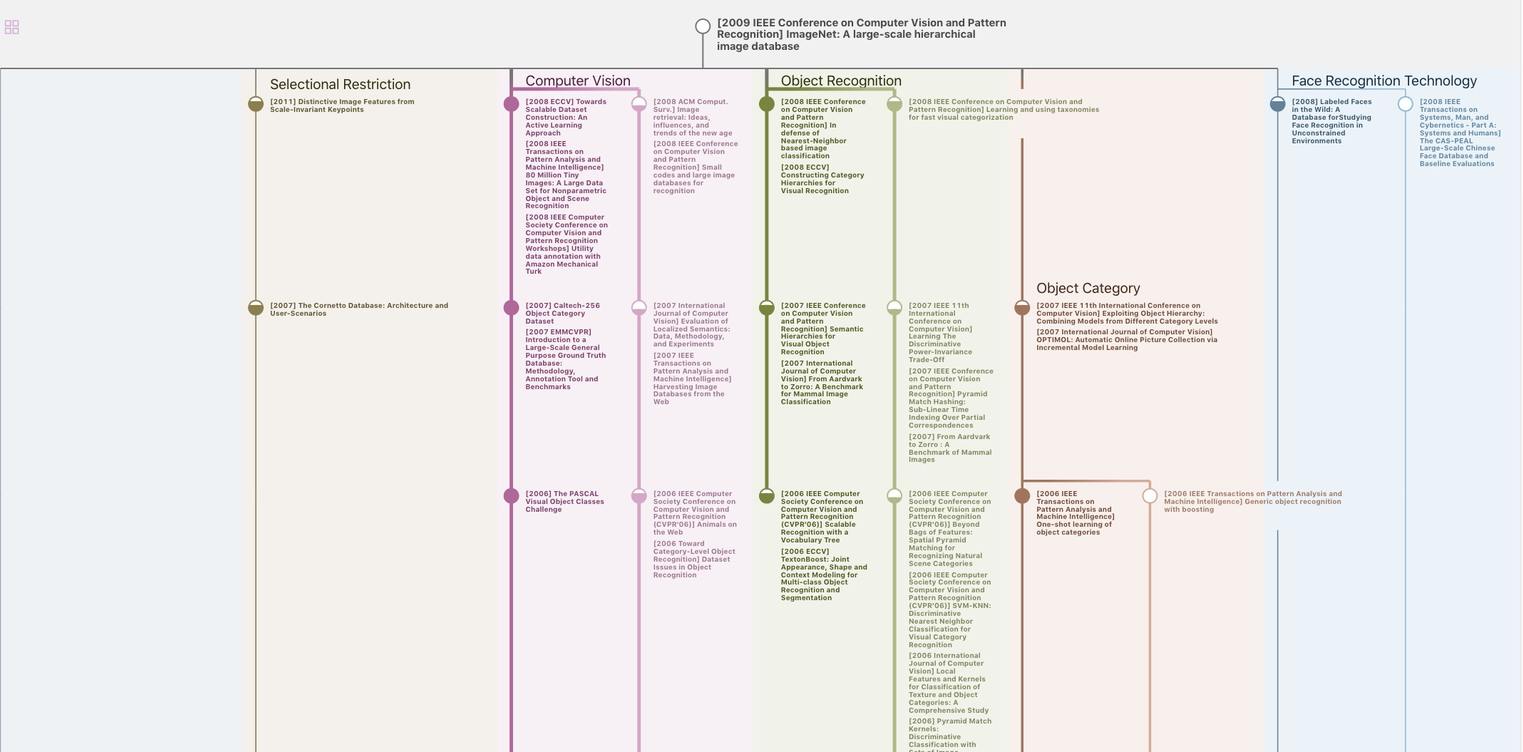
Generate MRT to find the research sequence of this paper
Chat Paper
Summary is being generated by the instructions you defined