Deep Reinforcement Learning for Control of Time-Varying Musculoskeletal Systems With High Fatigability: A Feasibility Study
IEEE TRANSACTIONS ON NEURAL SYSTEMS AND REHABILITATION ENGINEERING(2022)
摘要
Functional electrical stimulation (FES) can be used to restore motor function to people with paralysis caused by spinal cord injuries (SCIs). However, chronically-paralyzed FES-stimulated muscles can fatigue quickly, which may decrease FES controller performance. In this work, we explored the feasibility of using deep neural network (DNN) controllers trained with reinforcement learning (RL) to control FES of upper-limb muscles after SCI. We developed upper-limb biomechanical models that exhibited increased muscle fatigability, decreased muscle recovery, and decreased muscle strength, as observed in people with chronic SCIs. Simulations confirmed that controller training time and controller performance are impaired to varying degrees by muscle fatigability. Also, the simulations showed that large muscle strength asymmetries between opposing muscles can substantially impair controller performance. However, the results of this study suggest that controller performance for highly-fatigable musculoskeletal systems can be preserved by allowing for rest between movements. Overall, the results suggest that RL can be used to successfully train FES controllers, even for highly-fatigable musculoskeletal systems.
更多查看译文
关键词
Muscles, Fatigue, Iron, Arms, Adaptation models, Biological system modeling, Biomechanics, Reinforcement learning, functional electrical stimulation, motor control, spinal cord injury, biomechanical model
AI 理解论文
溯源树
样例
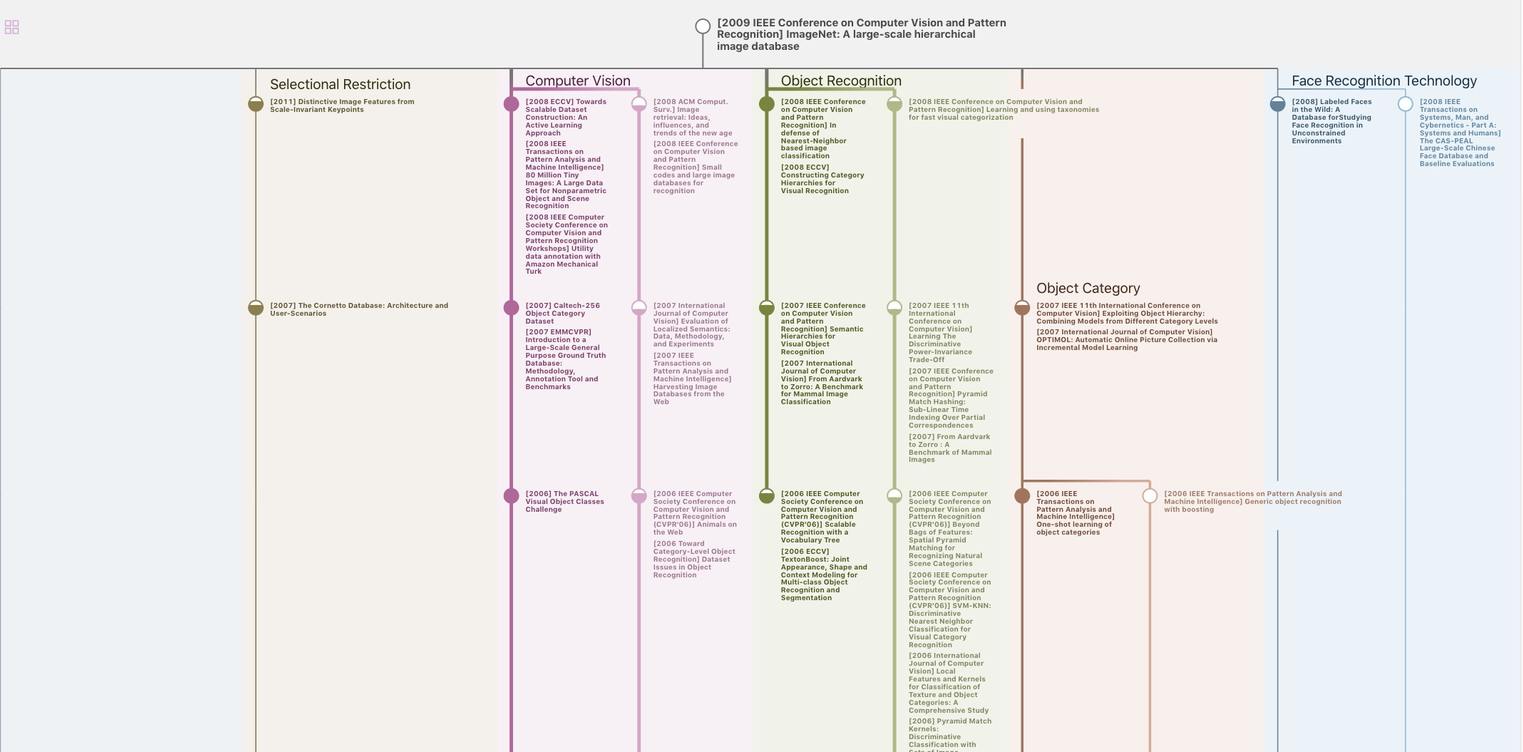
生成溯源树,研究论文发展脉络
Chat Paper
正在生成论文摘要