Prediction Based Decision Making for Autonomous Highway Driving
2022 IEEE 25th International Conference on Intelligent Transportation Systems (ITSC)(2022)
摘要
Autonomous driving decision-making is a challenging task due to the inherent complexity and uncertainty in traffic. For example, adjacent vehicles may change their lane or overtake at any time to pass a slow vehicle or to help traffic flow. Anticipating the intention of surrounding vehicles, estimating their future states and integrating them into the decision-making process of an automated vehicle can enhance the reliability of autonomous driving in complex driving scenarios. This paper proposes a Prediction-based Deep Reinforcement Learning (PDRL) decision-making model that considers the manoeuvre intentions of surrounding vehicles in the decision-making process for highway driving. The model is trained using real traffic data and tested in various traffic conditions through a simulation platform. The results show that the proposed PDRL model improves the decision-making performance compared to a Deep Reinforcement Learning (DRL) model by decreasing collision numbers, resulting in safer driving.
更多查看译文
关键词
traffic flow,automated vehicle,traffic data,traffic conditions,safety driving,autonomous highway driving decision-making,prediction-based deep reinforcement learning decision making,PDRL decision-making,simulation platform,collision number
AI 理解论文
溯源树
样例
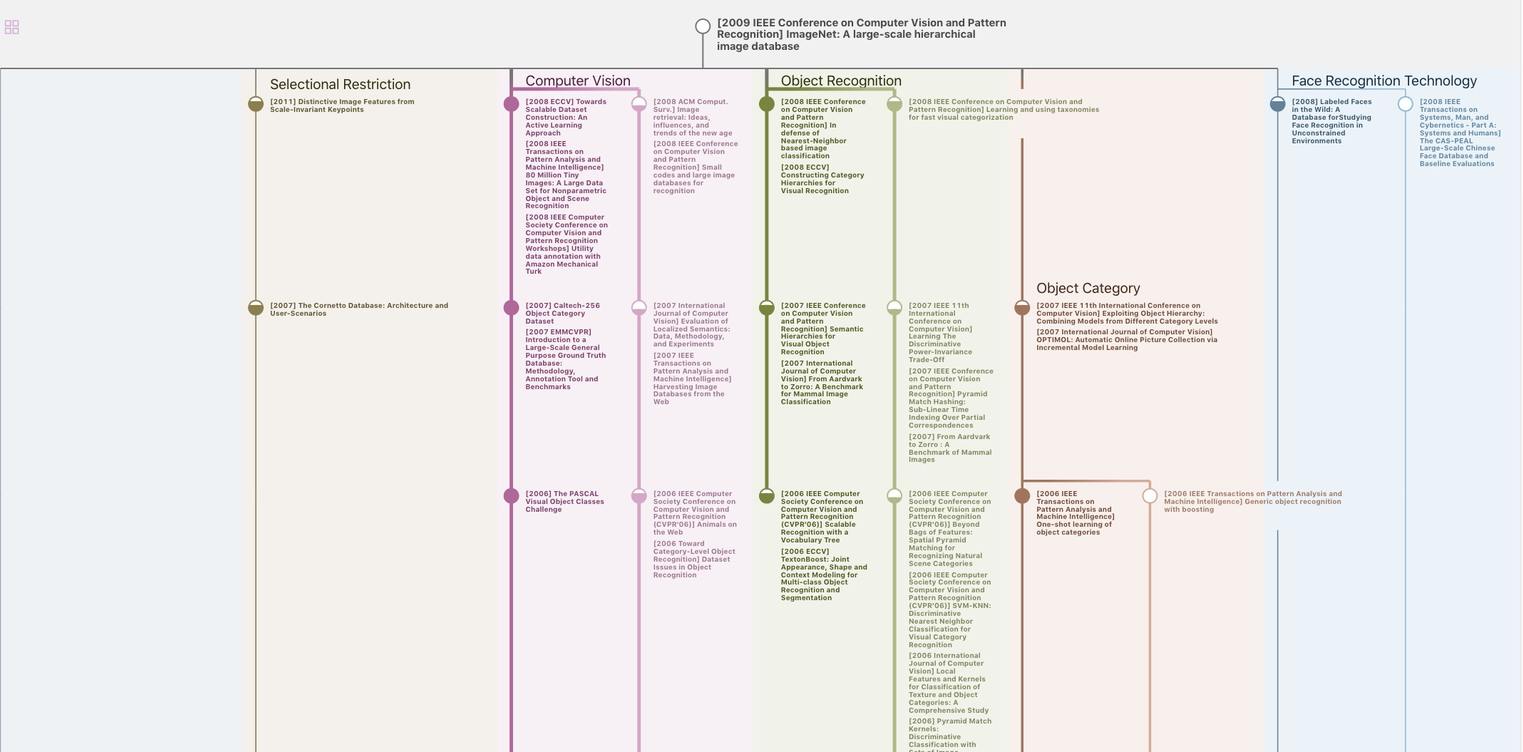
生成溯源树,研究论文发展脉络
Chat Paper
正在生成论文摘要