Incremental permutation feature importance (iPFI): towards online explanations on data streams
arxiv(2023)
摘要
Explainable artificial intelligence has mainly focused on static learning scenarios so far. We are interested in dynamic scenarios where data is sampled progressively, and learning is done in an incremental rather than a batch mode. We seek efficient incremental algorithms for computing feature importance (FI). Permutation feature importance (PFI) is a well-established model-agnostic measure to obtain global FI based on feature marginalization of absent features. We propose an efficient, model-agnostic algorithm called iPFI to estimate this measure incrementally and under dynamic modeling conditions including concept drift. We prove theoretical guarantees on the approximation quality in terms of expectation and variance. To validate our theoretical findings and the efficacy of our approaches in incremental scenarios dealing with streaming data rather than traditional batch settings, we conduct multiple experimental studies on benchmark data with and without concept drift.
更多查看译文
关键词
incremental permutation feature importance,data streams
AI 理解论文
溯源树
样例
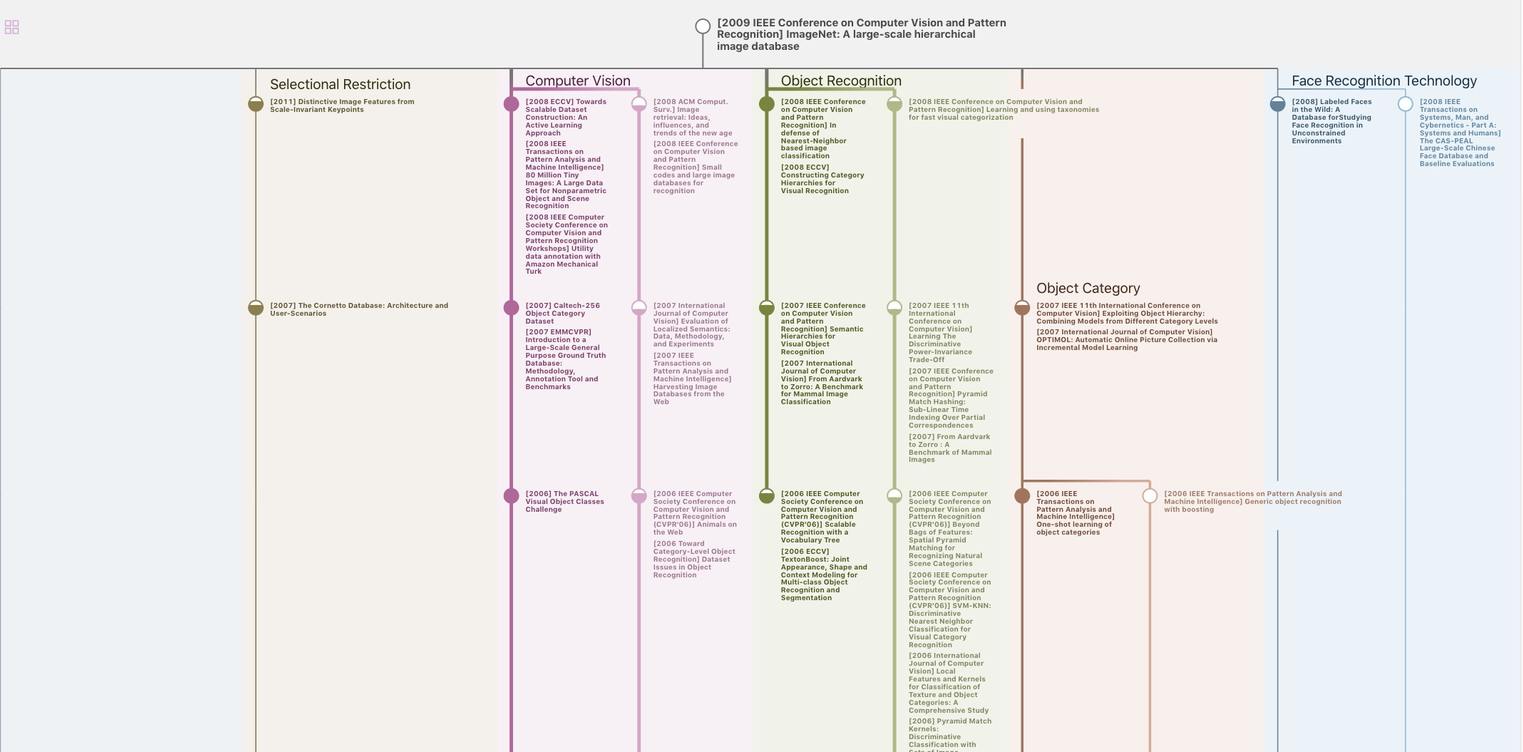
生成溯源树,研究论文发展脉络
Chat Paper
正在生成论文摘要