Transfer learning of an ensemble of DNNs for SSVEP BCI spellers without user-specific training.
Journal of neural engineering(2023)
摘要
Steady-state visually evoked potentials (SSVEPs), measured with electroencephalogram (EEG), yield decent information transfer rates (ITRs) in brain-computer interface (BCI) spellers. However, the current high performing SSVEP BCI spellers in the literature require an initial lengthy and tiring user-specific training for each new user for system adaptation, including data collection with EEG experiments, algorithm training and calibration (all are before the actual use of the system). This impedes the widespread use of BCIs. To ensure practicality, we propose a novel target identification method based on an ensemble of deep neural networks (DNNs), which does not require any sort of user-specific training.We exploit already-existing literature datasets from participants of previously conducted EEG experiments to train a global target identifier DNN first, which is then fine-tuned to each participant. We transfer this ensemble of fine-tuned DNNs to the new user instance, determine themost representative DNNs according to the participants' statistical similarities to the new user, and predict the target character through a weighted combination of the ensemble predictions.The proposed method significantly outperforms all the state-of-the-art alternatives for all stimulation durations in [0.2-1.0] s on two large-scale benchmark and BETA datasets, and achieves impressive 155.51 bits/min and 114.64 bits/min ITRs. Code is available for reproducibility:https://github.com/osmanberke/Ensemble-of-DNNs.Our Ensemble-DNN method has the potential to promote the practical widespread deployment of BCI spellers in daily lives as we provide the highest performance while enabling the immediate system use without any user-specific training.
更多查看译文
关键词
brain computer interfaces,deep learning,ensemble,steady state visually evoked potential,transfer learning.
AI 理解论文
溯源树
样例
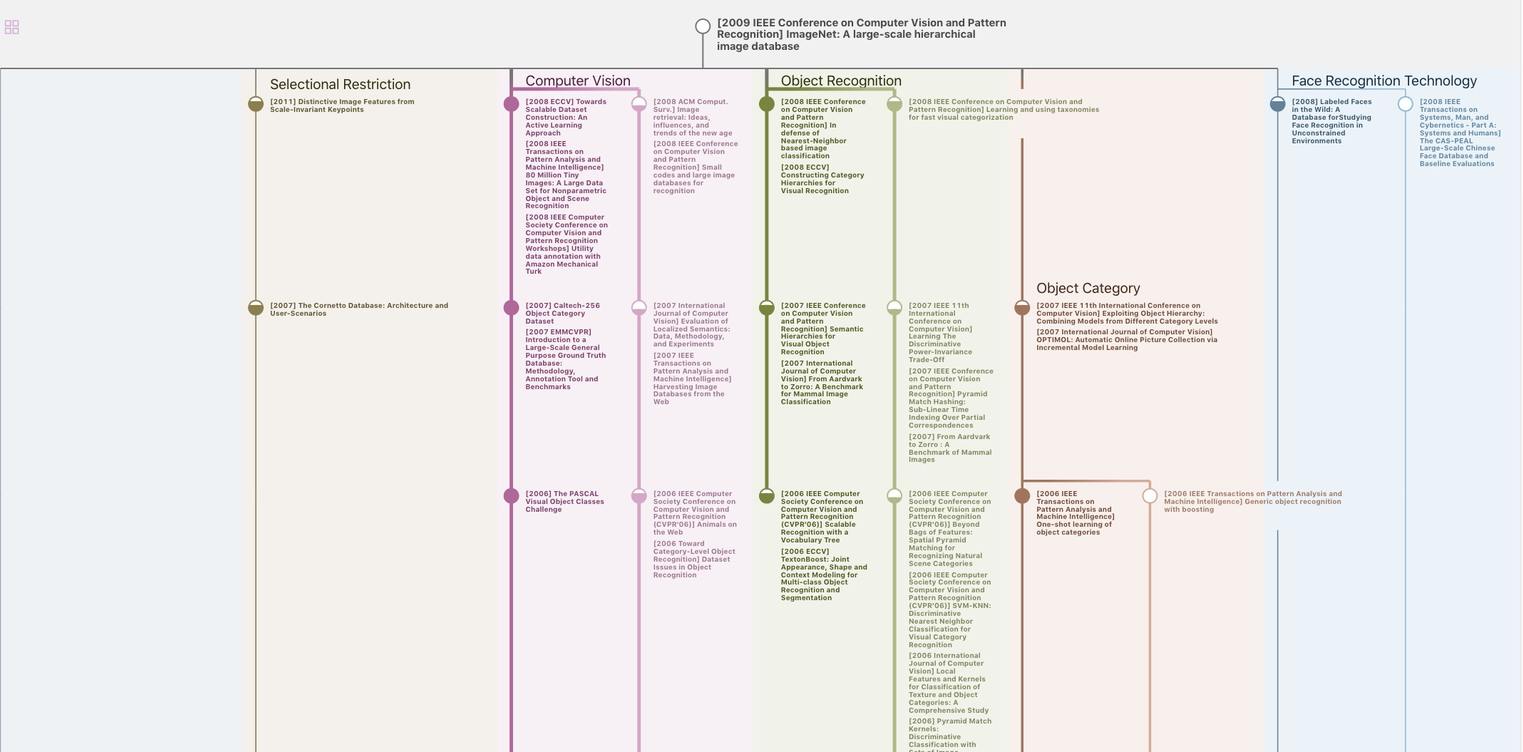
生成溯源树,研究论文发展脉络
Chat Paper
正在生成论文摘要