BGL-Net: A Brain-Inspired Global-Local Information Fusion Network for Alzheimers Disease Based on sMRI
IEEE Transactions on Cognitive and Developmental Systems(2023)
摘要
Alzheimer's disease (AD) is an irreversible neurodegenerative disease, the most common form of dementia, affecting millions worldwide. Neuroimaging-based early AD diagnosis has become an effective approach, especially by using structural magnetic resonance imaging (sMRI). The convolutional neural network (CNN)-based method is challenging to learn dependencies between spatially distant positions in the various brain regions due to its local convolution operation. In contrast, the graph convolutional network (GCN)-based work can connect the brain regions to capture global information but is not sensitive to the local information in a single brain region. Unlike a separate CNN or GCN-based method, we proposed a brain-inspired global-local information fusion network (BGL-Net) to diagnose AD. It essentially inherits the advantages of both CNN and GCN. The experiments on three public data sets demonstrate the effectiveness and robustness of our BGL-Net. Our method achieved the best performance on three popular public data sets compared with the existing CNN and GCN-based methods. In addition, our visualization results of the learned brain connection on AD and normal people agree with many current AD clinical research.
更多查看译文
关键词
Alzheimer's disease (AD), cognitive assessment, convolutional neural networks (CNNs), graph neural networks, structural magnetic resonance imaging (sMRI)
AI 理解论文
溯源树
样例
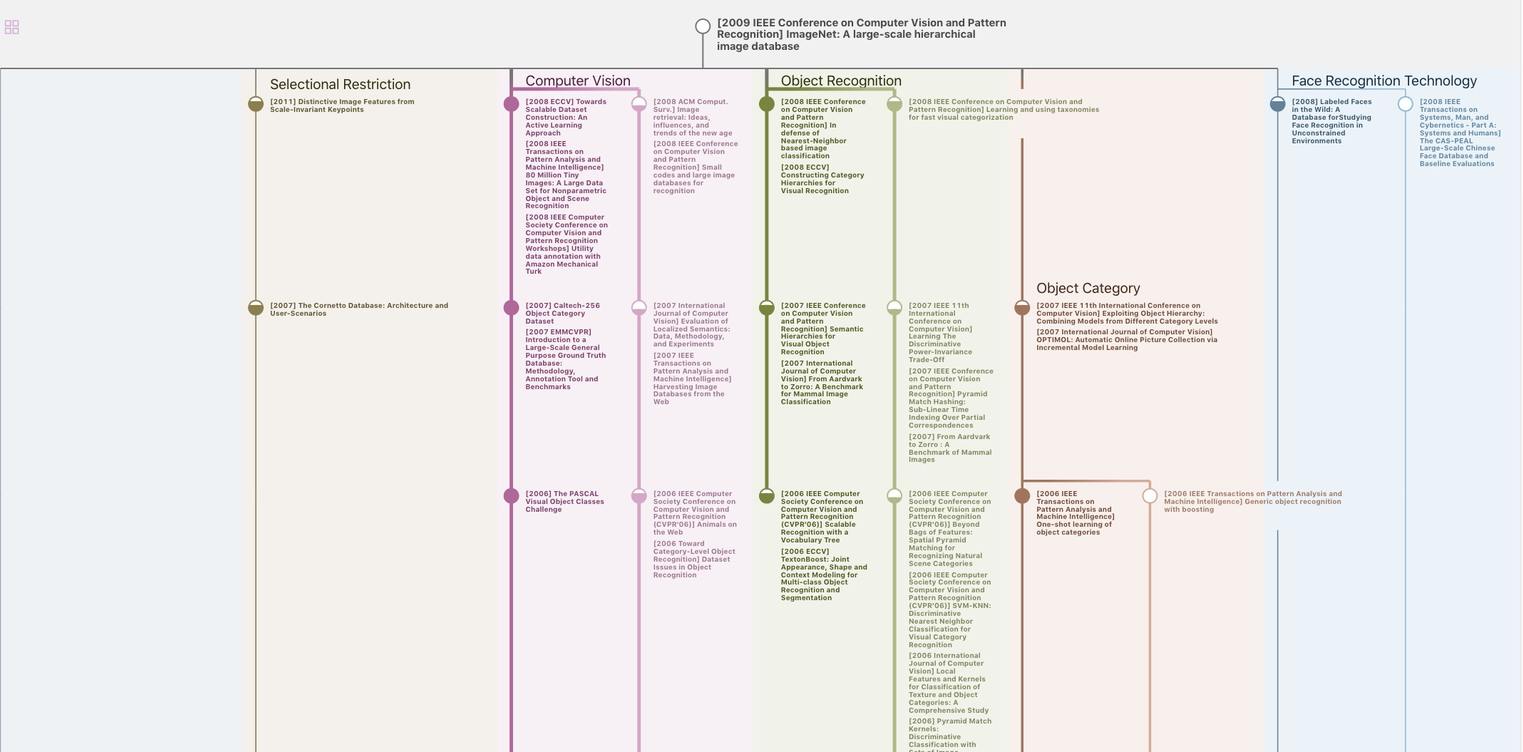
生成溯源树,研究论文发展脉络
Chat Paper
正在生成论文摘要