Fast estimation and choice of confidence interval methods for step regression
ENVIRONMENTAL AND ECOLOGICAL STATISTICS(2022)
摘要
In this paper we propose a new fast grid search algorithm for finding the least square estimators of a step regression model. This algorithm makes it practical to compute resampling-based confidence intervals for step regression models. We introduce five data generating models, including one where the mean model is a step model (model correctly specified) and four where the mean models are not step models (model misspecified), and use them to study the coverage probabilities of two new types of resampling-based confidence intervals for step regression: symmetric percentile bootstrap confidence intervals and subsampling confidence intervals using a new set of rules-of-thumb to select block size. Our results show that when the model is correctly specified, the symmetric percentile Efron bootstrap confidence intervals provide close-to-nominal coverage and have shorter intervals than the subsampling methods; when the model is misspecified, the subsampling method using the rules-of-thumb provides good coverage and shorter confidence intervals than the symmetric percentile Efron bootstrap method and the subsampling method using a double bootstrap-like procedure for block size selection. Finally, we apply the proposed methods to a real world environmental dataset on the relationship between grassland productivity, soil moisture anomalies and other hydro-climatic and land use variables to provide inference for the threshold in soil moisture anomalies, across which there is a jump in grassland productivity.
更多查看译文
关键词
Dynamic programming,Ecology,Subsampling,Symmetric percentile bootstrap confidence intervals
AI 理解论文
溯源树
样例
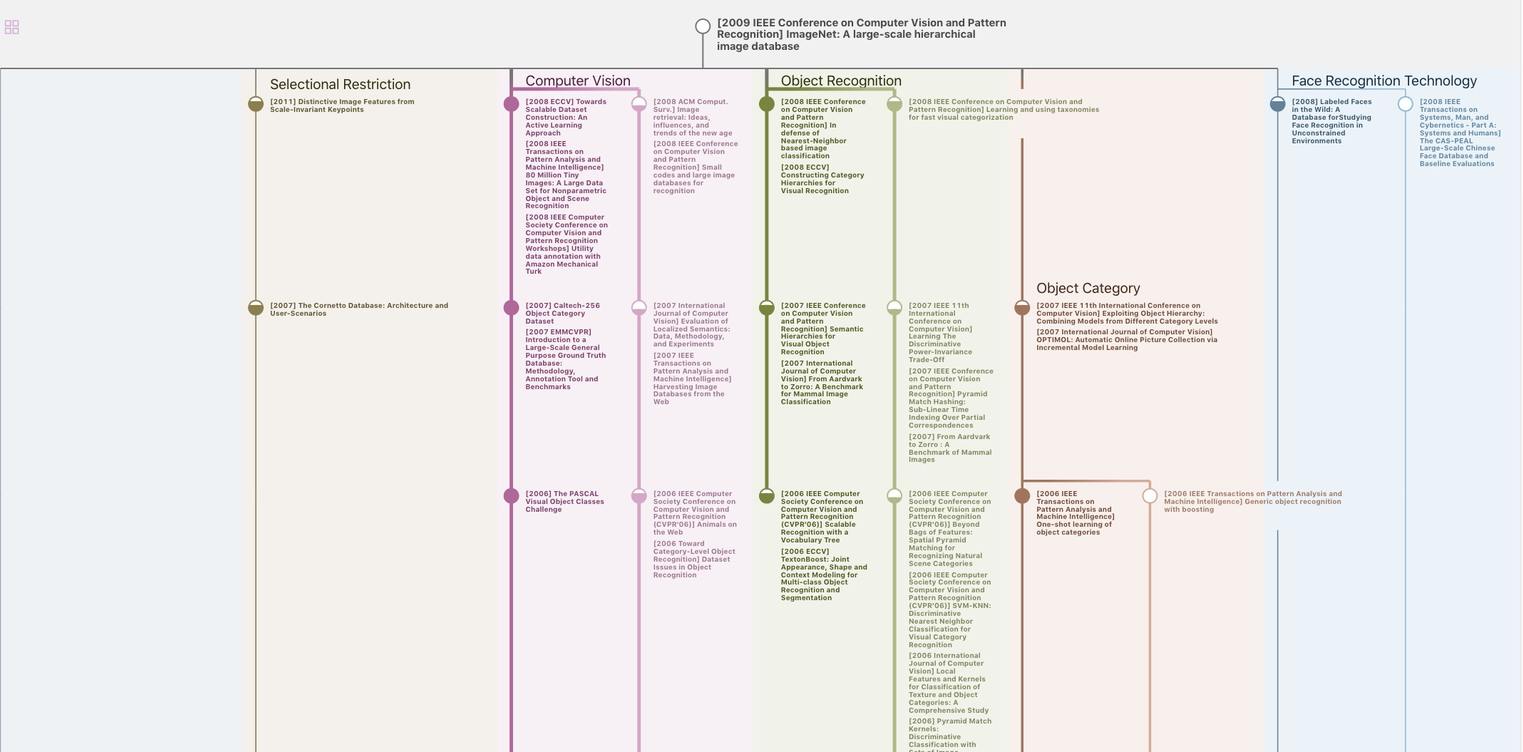
生成溯源树,研究论文发展脉络
Chat Paper
正在生成论文摘要