LADFN: Learning Actions for Drift-Free Navigation in Highly Dynamic Scenes
2022 American Control Conference (ACC)(2022)
摘要
We embark on a hitherto unreported problem of an autonomous robot (self-driving car) navigating in dynamic scenes in a manner that reduces its localization error and eventual cumulative drift or Absolute Trajectory Error, which is pronounced in such dynamic scenes. With the hugely popular Velodyne-16 3D LIDAR as the main sensing modality, and the accurate LIDAR-based Localization and Mapping algorithm, LOAM, as the state estimation framework, we show that in the absence of a navigation policy, drift rapidly accumulates in the presence of moving objects. To overcome this, we learn actions that lead to drift-minimized navigation through a suitable set of reward and penalty functions. We use Proximal Policy Optimization, a class of Deep Reinforcement Learning methods, to learn the actions that result in drift-minimized trajectories. We show by extensive comparisons on a variety of synthetic, yet photo-realistic scenes made available through the CARLA Simulator the superior performance of the proposed framework vis-a-vis methods that do not adopt such policies.
更多查看译文
关键词
dynamic scenes,learning,drift-free
AI 理解论文
溯源树
样例
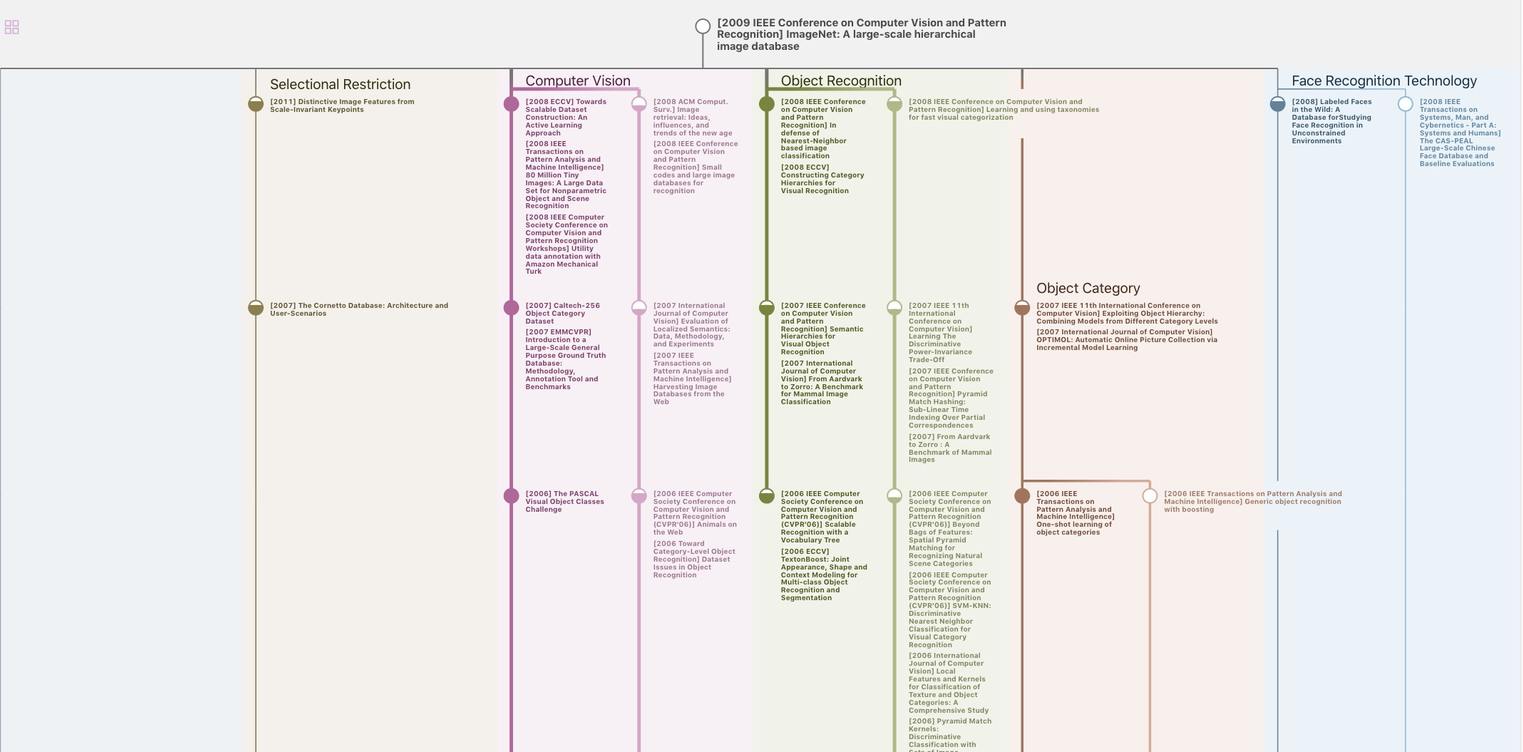
生成溯源树,研究论文发展脉络
Chat Paper
正在生成论文摘要