Visual state estimation in unseen environments through domain adaptation and metric learning
FRONTIERS IN ROBOTICS AND AI(2022)
摘要
In robotics, deep learning models are used in many visual perception applications, including the tracking, detection and pose estimation of robotic manipulators. The state of the art methods however are conditioned on the availability of annotated training data, which may in practice be costly or even impossible to collect. Domain augmentation is one popular method to improve generalization to out-of-domain data by extending the training data set with predefined sources of variation, unrelated to the primary task. While this typically results in better performance on the target domain, it is not always clear that the trained models are capable to accurately separate the signals relevant to solving the task (e.g., appearance of an object of interest) from those associated with differences between the domains (e.g., lighting conditions). In this work we propose to improve the generalization capabilities of models trained with domain augmentation by formulating a secondary structured metric-space learning objective. We concentrate on one particularly challenging domain transfer task-visual state estimation for an articulated underground mining machine-and demonstrate the benefits of imposing structure on the encoding space. Our results indicate that the proposed method has the potential to transfer feature embeddings learned on the source domain, through a suitably designed augmentation procedure, and on to an unseen target domain.
更多查看译文
关键词
articulated pose estimation, joint state estimation, deep metric learning, domain augmentation, triplet loss
AI 理解论文
溯源树
样例
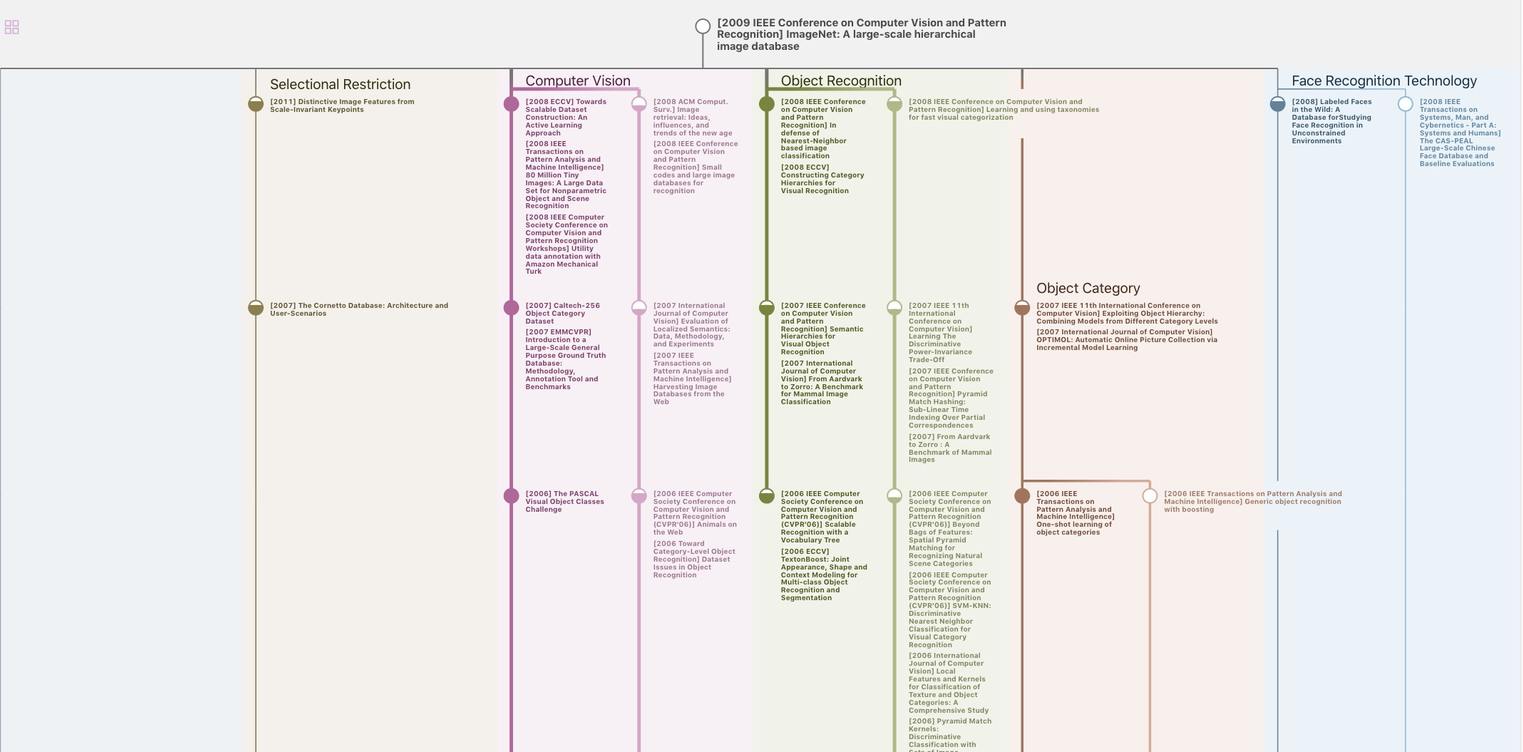
生成溯源树,研究论文发展脉络
Chat Paper
正在生成论文摘要