Bearing Fault Classification using Empirical Mode Decomposition and Machine Learning Approach
Journal of Mines, Metals and Fuels(2022)
摘要
Industrial machinery often breakdowns due to faults in rolling bearing. Bearing diagnosis plays a vital role in condition monitoring of machinery. Operating conditions and working environment of bearings make them prone to single or multiple faults. In this research, signals from both healthy and faulty bearings are extracted and decomposed into empirical modes. By analyzing different empirical modes from 8 derived empirical modes for healthy and faulty bearings under different fault sizes, the first mode has the most information to classify bearing condition. From the first empirical mode eight features in time domain were calculated for various bearing conditions like healthy, rolling element fault, outer and inner race fault. The feature extraction of vibration signal based on Empirical Mode Decomposition (EMD) is extensively explored and applied in diagnosis of fault in rolling bearings. This paper presents mathematical analysis for selection of valid Intrinsic Mode Functions (IMFs) of EMD. These chosen features are trained and classified using different classifiers. Among them K-star classifier is most reliable to categorize the bearing defects.
更多查看译文
AI 理解论文
溯源树
样例
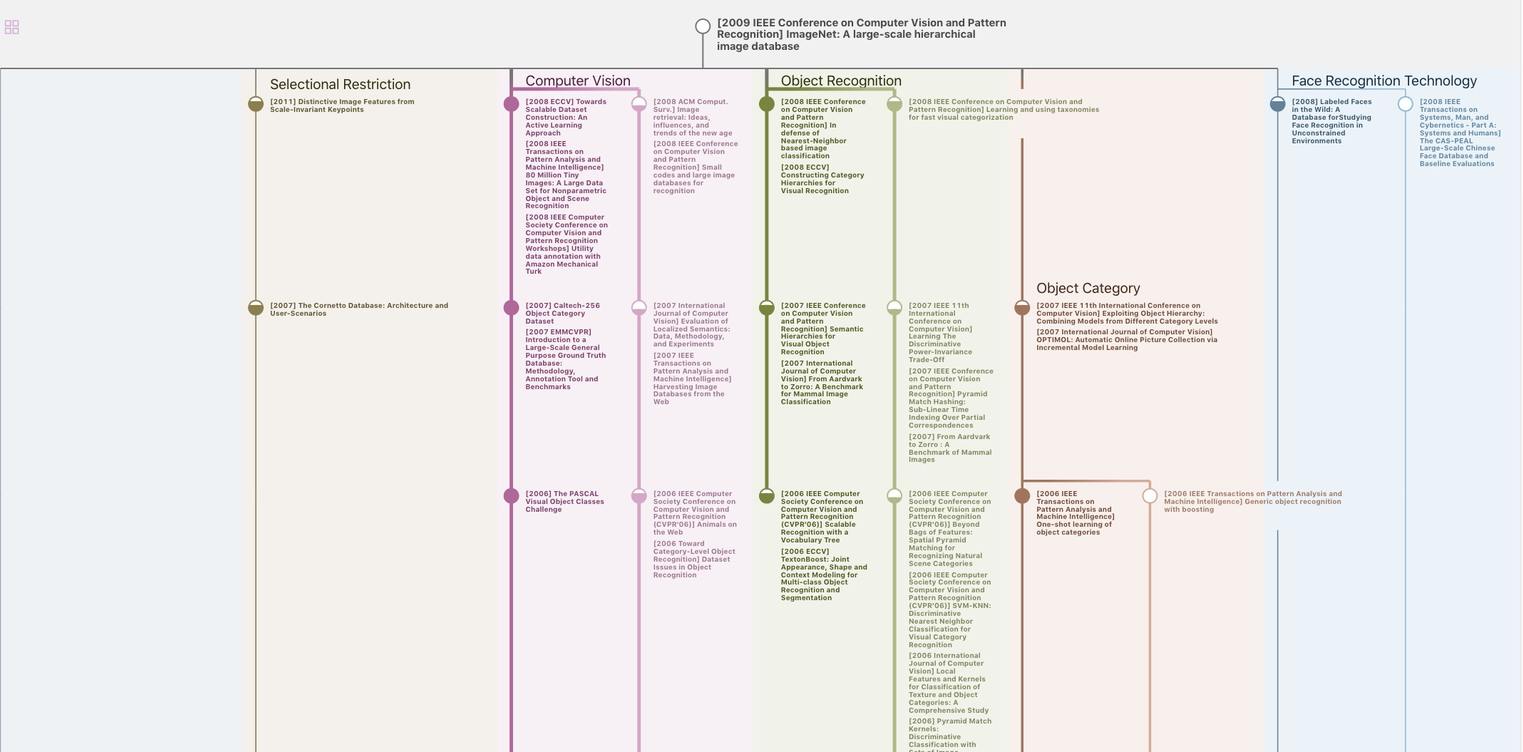
生成溯源树,研究论文发展脉络
Chat Paper
正在生成论文摘要