Dual recurrent neural networks using partial linear dependence for multivariate time series
Expert Systems with Applications(2022)
摘要
The analysis of multivariate time series is still challenging due to the dynamic interrelationships and complex dependencies over time between features. Recently, various deep RNN-LSTM models have been used for the prediction for multivariate time series. Specifically, when a structural change of the model is needed, one often extracts new features by connecting additional neural networks. However, when connected and combined, simple concatenation of the features as the default choice is limited in that it hardly reflects the dependencies between them. Thus, in this paper we propose partial linear dependence for the connection of dual RNNs structures. Partial linear dependence is a statistical measure, driven by linear dependence and partial least squares, to measure the amount of dependency between two signals while excluding information from past signals. Then, we propose a dual RNN structure that reflects the relationship between time-fragment multivariate time series by maximizing this partial linear dependence. When features are extracted from the proposed dual RNNs and combined, the overall model aims to increase the dependency with future fragments without overlapping. We demonstrate the performance of the proposed method in real-life multivariate datasets by comparing it with existing models and some variants with difference base cells such as RNN and GRU. We found that the proposed method shows better performance even when the same number of parameters is used and that, compared to the model without partial linear dependence, our model reduced the mean squared error substantially.
更多查看译文
关键词
RNN,LSTM,Dual,Partial linear dependence
AI 理解论文
溯源树
样例
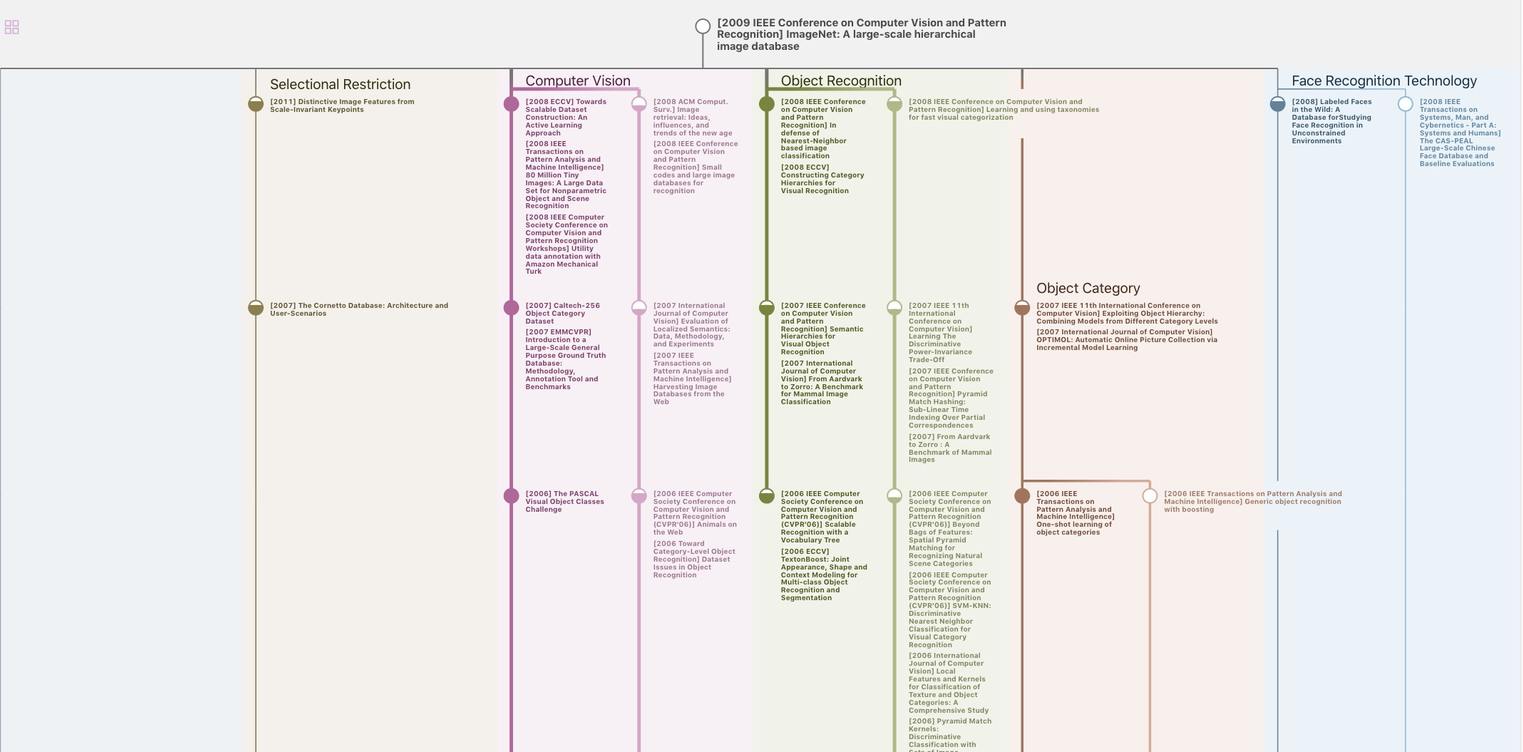
生成溯源树,研究论文发展脉络
Chat Paper
正在生成论文摘要