Neural Network-Assisted Design: A Study of Multi-Scale Topology Optimization with Smoothly Graded Cellular Structures
Journal of Mechanical Design(2022)
摘要
Abstract Integration of machine learning (ML) with topology optimization (TO) has been attempted in many works. However, most works employ ML in a data-driven paradigm, which requires well-defined training data, and the generalization is questionable. This paper aims to utilize the optimization capability of ML for high-resolution structure design. Rather than learning a priori, the neural network (NN) acts as an optimizer in the TO problem. Specifically, the density field is reparametrized into a function representation-based microstructure. The level surface is the design parameter that controls the microstructure's volume and shape. We reformulate the multi-scale TO problem with this new design variable. NN is utilized to map the microstructure parameters into the design domain. The input of NN is spatial coordinates, and the output is the microstructure level surface value. The network parameters are optimized through backpropagation, which leads to optimal design. In this framework, predicting the microstructure's parameter at any arbitrary point is possible by taking advantage of the mesh size-independent continuous NN. Once the network is optimized, the resolution of structures can be increased accordingly without increasing computational cost. This is crucial to address the sharp transition problem of adjacent microstructures--a common one in the multi-scale structure design. Several benchmarks have been studied to validate the proposed method's effectiveness. Experimental results demonstrate that our work yields high-resolution designs with smooth transitions and improves the overall performance of final structures compared to previous methods.
更多查看译文
关键词
computer-aided design,neural network-assisted design,design for manufacturing
AI 理解论文
溯源树
样例
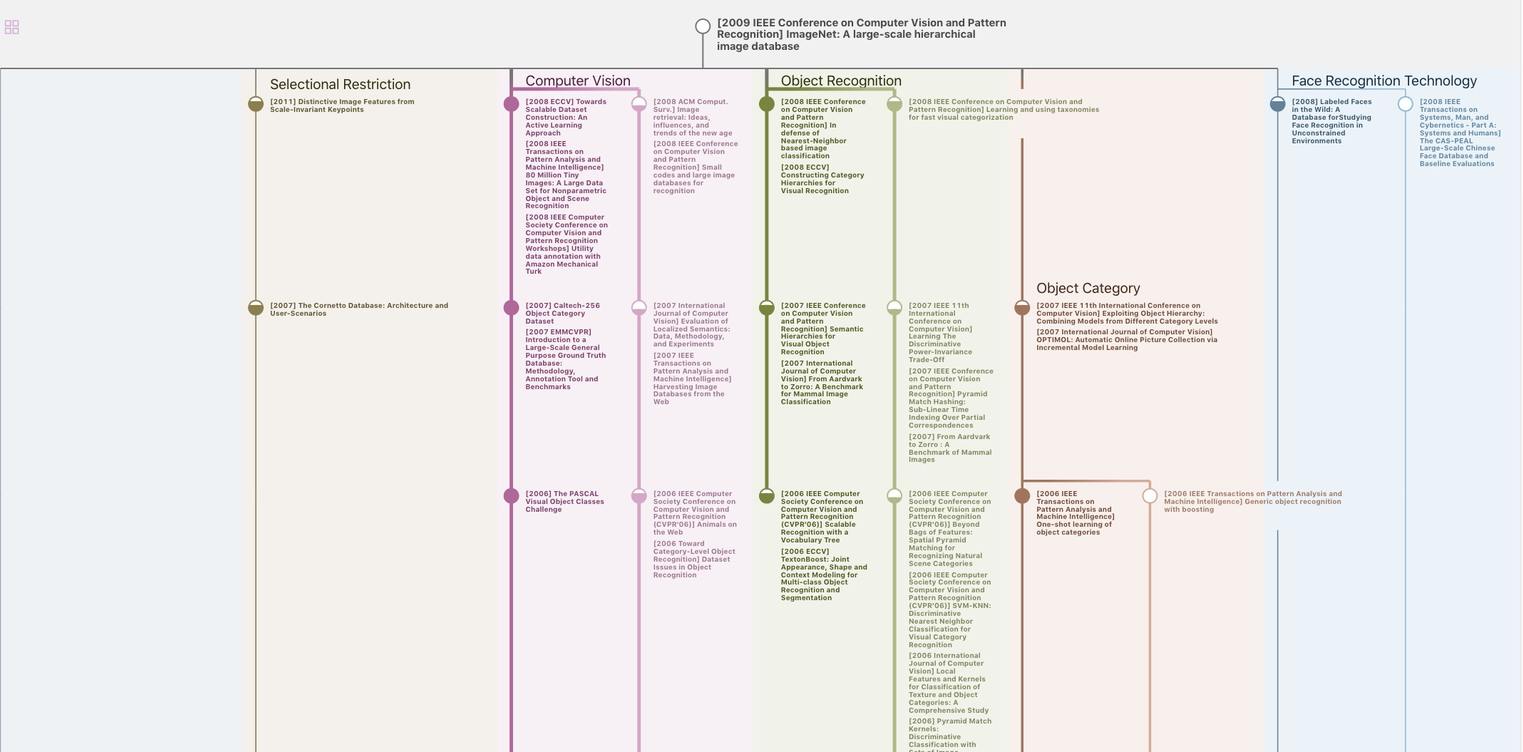
生成溯源树,研究论文发展脉络
Chat Paper
正在生成论文摘要