Situational continuity-based air combat autonomous maneuvering decision-making
DEFENCE TECHNOLOGY(2023)
Abstract
In order to improve the performance of UAV's autonomous maneuvering decision-making, this paper proposes a decision-making method based on situational continuity. The algorithm in this paper designs a situation evaluation function with strong guidance, then trains the Long Short-Term Memory (LSTM) under the framework of Deep Q Network (DQN) for air combat maneuvering decision-making. Considering the continuity between adjacent situations, the method takes multiple consecutive situations as one input of the neural network. To reflect the difference between adjacent situations, the method takes the difference of situation evaluation value as the reward of reinforcement learning. In different scenarios, the algorithm proposed in this paper is compared with the algorithm based on the Fully Neural Network (FNN) and the algorithm based on statistical principles respectively. The results show that, compared with the FNN algorithm, the algorithm proposed in this paper is more accurate and forwardlooking. Compared with the algorithm based on the statistical principles, the decision-making of the algorithm proposed in this paper is more efficient and its real-time performance is better. (c) 2022 China Ordnance Society. Publishing services by Elsevier B.V. on behalf of KeAi Communications Co. Ltd. This is an open access article under the CC BY-NC-ND license (http://creativecommons.org/ licenses/by-nc-nd/4.0/).
MoreTranslated text
Key words
UAV,Maneuvering decision-making,Situational continuity,Long short-term memory (LSTM),Deep Q network (DQN),Fully neural network (FNN)
AI Read Science
Must-Reading Tree
Example
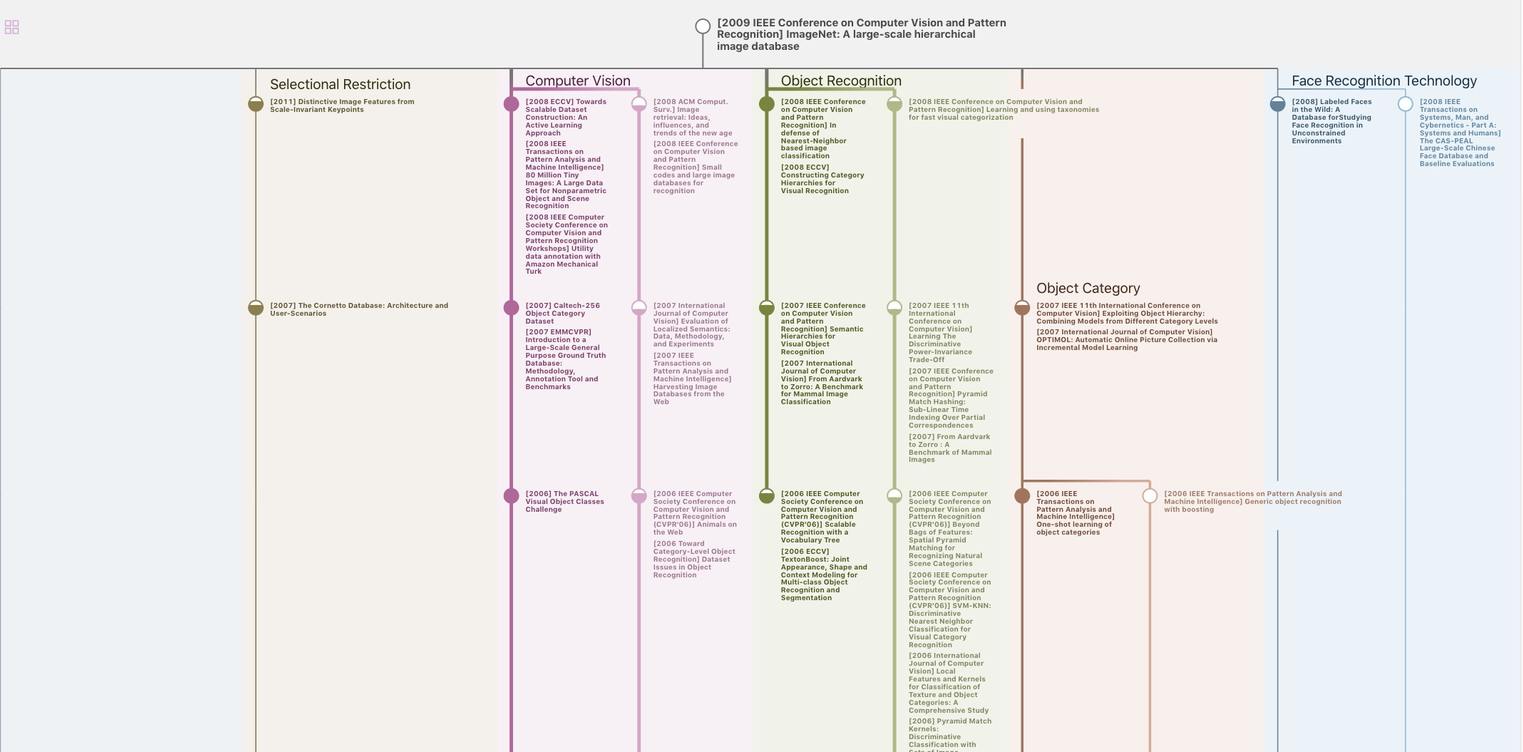
Generate MRT to find the research sequence of this paper
Chat Paper
Summary is being generated by the instructions you defined