A Workflow Scheduling Strategy for Reasoning Tasks of Autonomous Driving.
Int. J. Grid High Perform. Comput.(2022)
Abstract
In different periods of time, the real-time reasoning tasks generated by autonomous vehicles are scheduled within the tolerance time, which is an important problem to be solved in autonomous driving. Traditionally, tasks are arranged on the on-board unit (OBU), which results in a long time to complete. Heuristic algorithm is widely used in task scheduling, which often leads to premature convergence. Task scheduling in the edge environment can effectively reduce the completion time of tasks. A workflow scheduling strategy in edge environment is designed. To optimize the completion time of reasoning tasks, this paper proposes a Q-learning algorithm based on simulated annealing (SA-QL). Moreover, this paper comprehensively reflects the performance of SA-RL and PSO algorithm from four aspects. Experimental results show that SA-RL algorithm and PSO algorithm have good performance in feasibility and effectiveness. TD(0) algorithms show better performance of exploration, TD(λ) algorithms show that of convergence.
MoreTranslated text
Key words
Autonomous Driving, Edge Computing, Reinforcement Learning, Workflow Scheduling
AI Read Science
Must-Reading Tree
Example
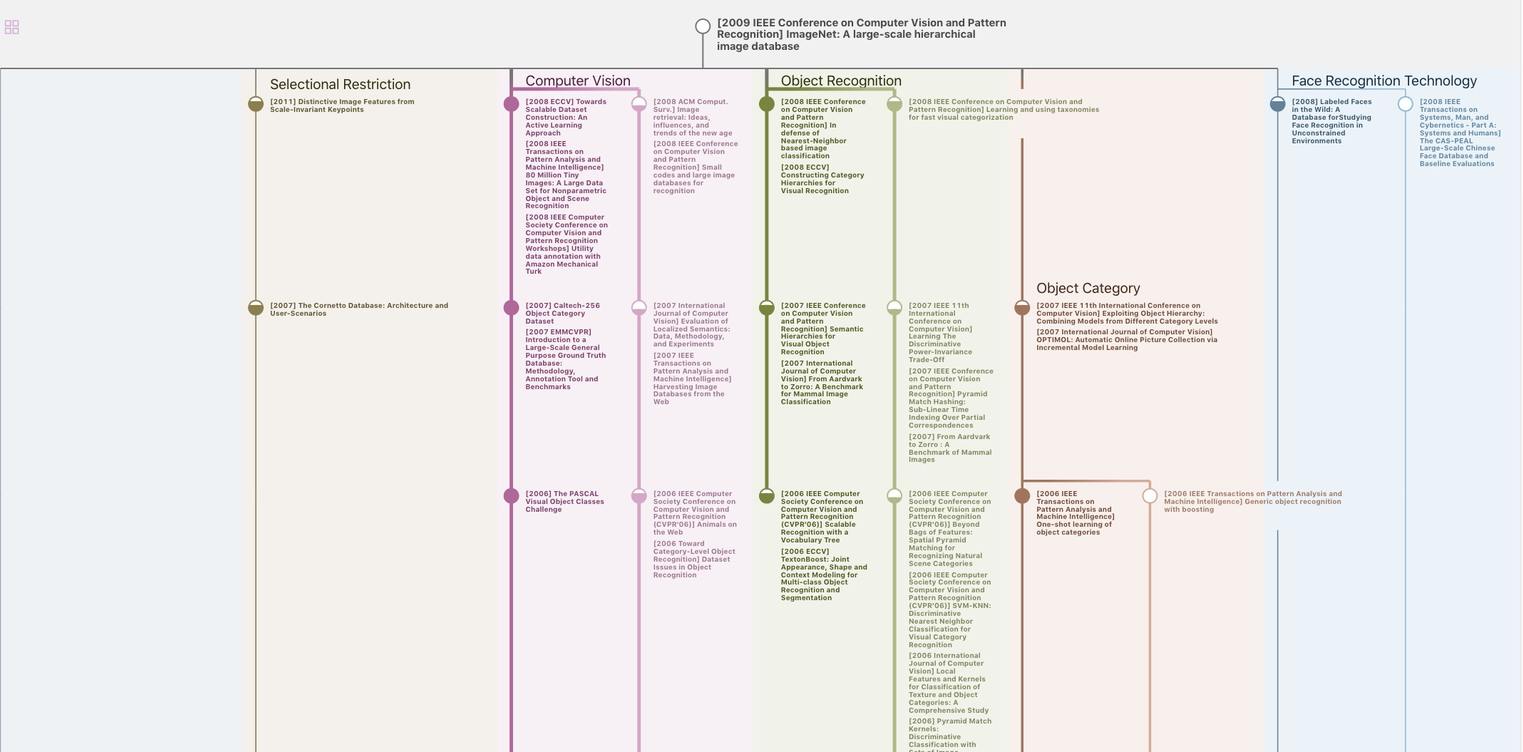
Generate MRT to find the research sequence of this paper
Chat Paper
Summary is being generated by the instructions you defined