Back-to-Bones: Rediscovering the Role of Backbones in Domain Generalization
arxiv(2022)
摘要
Domain Generalization (DG) studies the capability of a deep learning model to generalize to out-of-training distributions. In the last decade, literature has been massively filled with a collection of training methodologies that claim to obtain more abstract and robust data representations to tackle domain shifts. Recent research has provided a reproducible benchmark for DG, pointing out the effectiveness of naive empirical risk minimization (ERM) over existing algorithms. Nevertheless, researchers persist in using the same outdated feature extractors, and no attention has been given to the effects of different backbones yet. In this paper, we start back to backbones proposing a comprehensive analysis of their intrinsic generalization capabilities, so far ignored by the research community. We evaluate a wide variety of feature extractors, from standard residual solutions to transformer-based architectures, finding an evident linear correlation between large-scale single-domain classification accuracy and DG capability. Our extensive experimentation shows that by adopting competitive backbones in conjunction with effective data augmentation, plain ERM outperforms recent DG solutions and achieves state-of-the-art accuracy. Moreover, our additional qualitative studies reveal that novel backbones give more similar representations to same-class samples, separating different domains in the feature space. This boost in generalization capabilities leaves marginal room for DG algorithms and suggests a new paradigm for investigating the problem, placing backbones in the spotlight and encouraging the development of consistent algorithms on top of them.
更多查看译文
关键词
backbones,domain
AI 理解论文
溯源树
样例
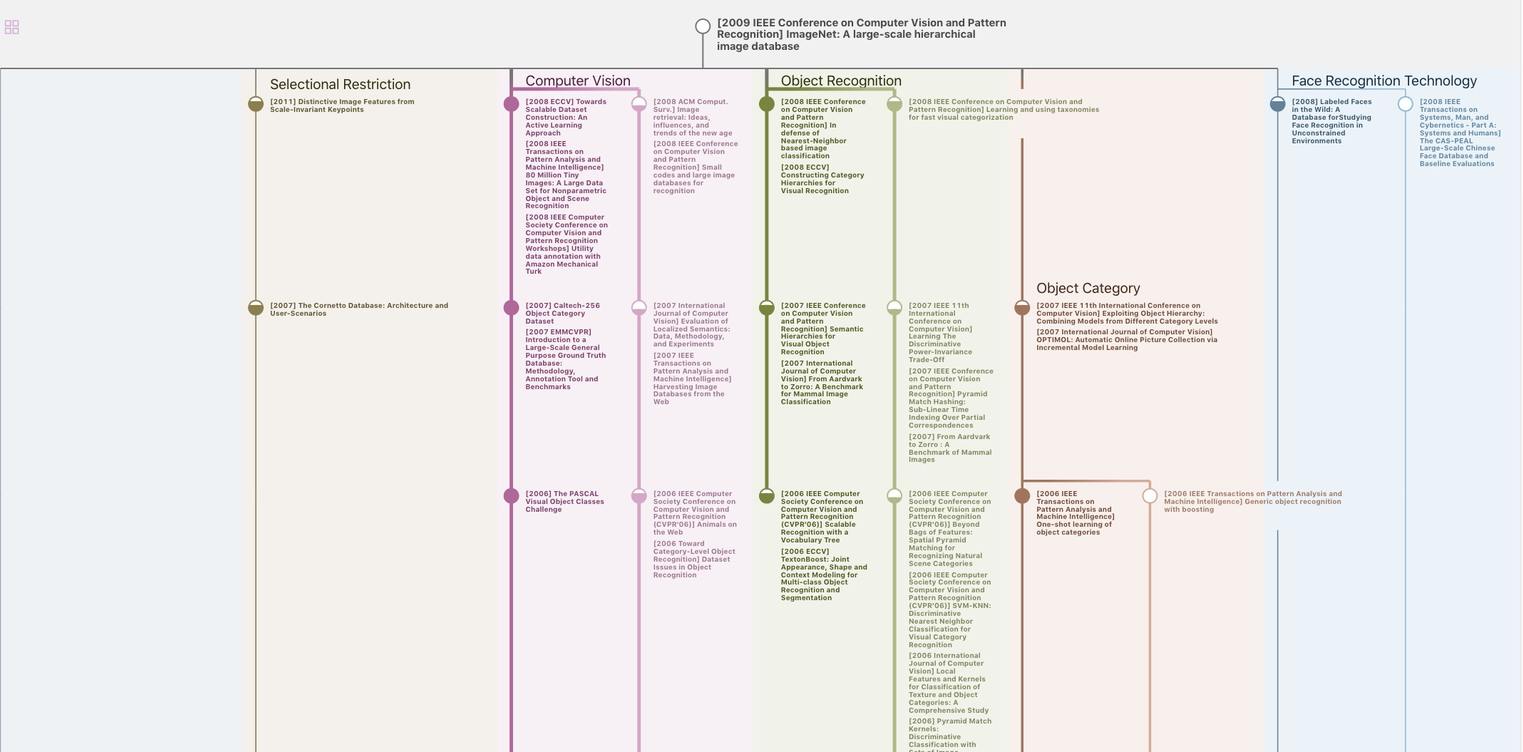
生成溯源树,研究论文发展脉络
Chat Paper
正在生成论文摘要