An Explainer for Temporal Graph Neural Networks.
GLOBECOM(2022)
摘要
Temporal graph neural networks (TGNNs) have been widely used for modeling time-evolving graph-related tasks due to their ability to capture both graph topology dependency and non-linear temporal dynamic. The explanation of TGNNs is of vital importance for a transparent and trustworthy model. However, the complex topology structure and temporal dependency make explaining TGNN models very challenging. In this paper, we propose a novel explainer framework for TGNN models. Given a time series on a graph to be explained, the framework can identify dominant explanations in the form of a probabilistic graphical model in a time period. Case studies on the transportation domain demonstrate that the proposed approach can discover dynamic dependency structures in a road network for a time period.
更多查看译文
关键词
TGNN,graph explanations,interpretable DL
AI 理解论文
溯源树
样例
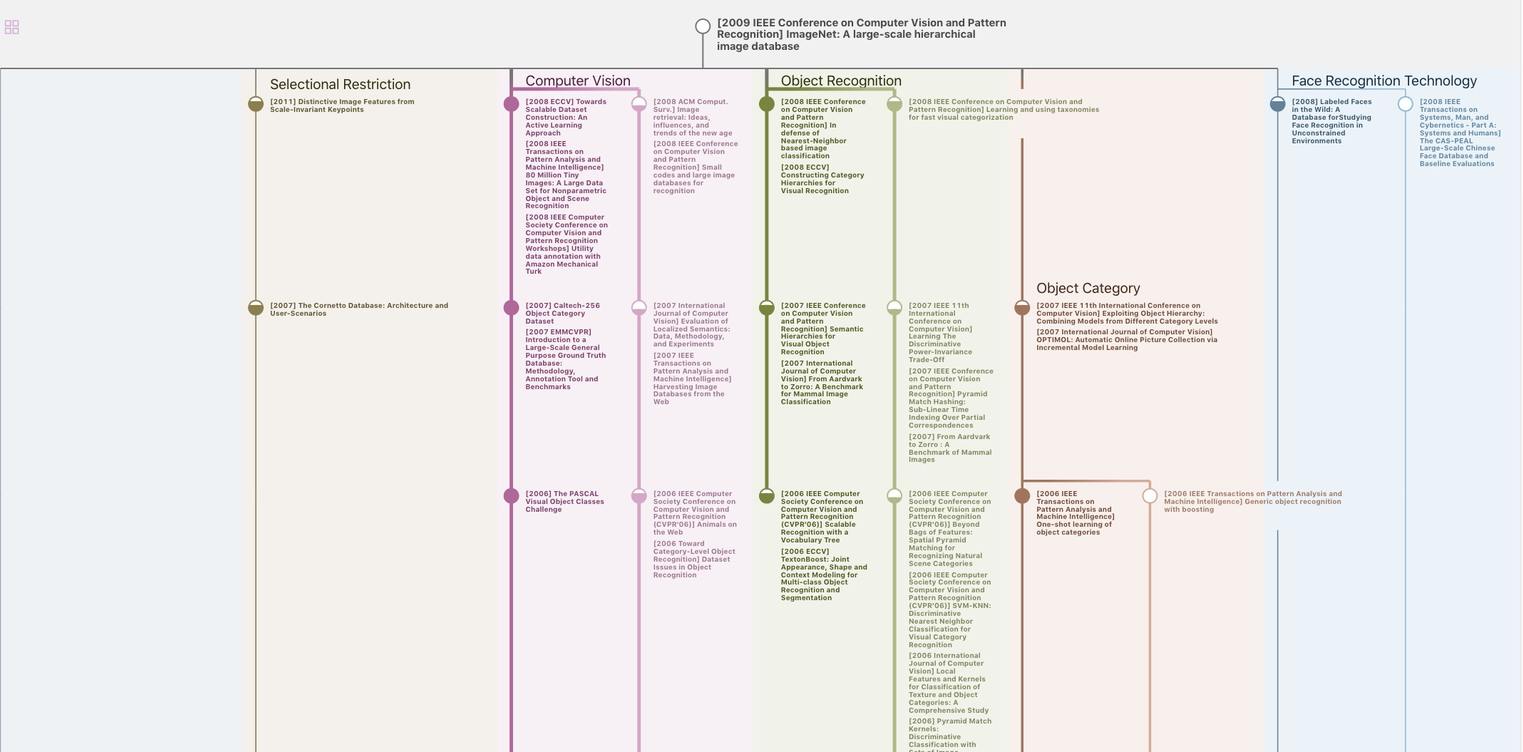
生成溯源树,研究论文发展脉络
Chat Paper
正在生成论文摘要