Extraction of multi-scale features enhances the deep learning-based daily PM2.5 forecasting in cities
Chemosphere(2022)
摘要
Characterising the daily PM2.5 concentration is crucial for air quality control. To govern the status of the atmospheric environment, a novel hybrid model for PM2.5 forecasting was proposed by introducing a two-stage decomposition technology of complete ensemble empirical mode decomposition with adaptive noise (CEEMDAN) and variational mode decomposition (VMD); subsequently, a deep learning approach of long short-term memory (LSTM) was proposed. Five cities with unique meteorological and economic characteristics were selected to assess the predictive ability of the proposed model. The results revealed that PM2.5 pollution was generally more severe in inland cities (66.98 ± 0.76 μg m−3) than in coastal cities (40.46 ± 0.40 μg m-3). The modelling comparison showed that in each city, the secondary decomposition algorithm improved the accuracy and prediction stability of the prediction models. When compared with other prediction models, LSTM effectively extracted featured information and achieved relatively accurate time-series prediction. The hybrid model of CEEMDAN-VMD-LSTM achieved a better prediction in the five cities (R2 = 0.9803 ± 0.01) compared with the benchmark models (R2 = 0.7537 ± 0.03). The results indicate that the proposed approach can identify the inherent correlations and patterns among complex datasets, particularly in time-series analysis.
更多查看译文
关键词
Multi-scale features extractions,Deep learning,Hybrid modelling,PM2.5,Two-stage decomposition
AI 理解论文
溯源树
样例
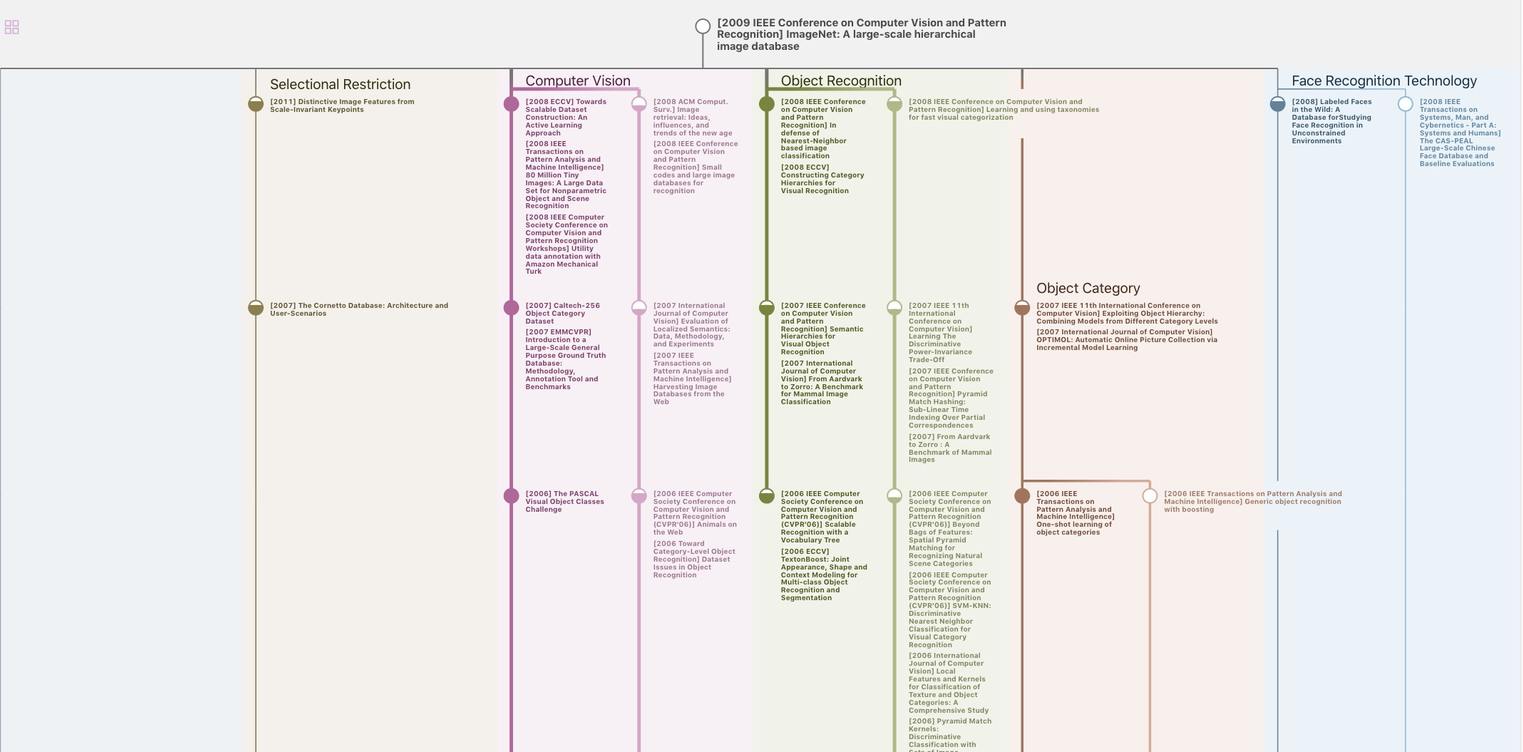
生成溯源树,研究论文发展脉络
Chat Paper
正在生成论文摘要