Use of machine learning on clinical questionnaires data to support the diagnostic classification of Attention DeficitHyperactivity Disorder: a personalized medicine approach
European Psychiatry(2022)
摘要
Introduction Attention Deficit / Hyperactivity Disorder (ADHD) is a highly prevalent neurodevelopmental condition characterized by inattention, motor hyperactivity and impulsivity. ADHD cognitive and behavioral presentation is characterized by a high heterogeneity (APA, 2013). Indeed, a complex diagnostic process, that considers several validated tools, is, to date, necessary. Objectives The main aim is to develop supervised machine learning (ML) algorithms that could be used to support the diagnostic process for ADHD, by identifying the most relevant features in discriminating between the presence or absence of the ADHD diagnosis in children. Methods We analyzed data from 342 children (Mean age: 8y 8m ± 1y; 61 F) referred for possible ADHD symptomatology. Assessments were performed by an expert clinician and through questionnaires: Social Responsiveness Scale (SRS), Child Behavior Checklist (CBCL), Conners Rating Scale for Parents (CPRS) and for Teachers (CTRS). Data were analyzed using a decision tree classifier and random forest algorithms. Results The decision tree model performed an accuracy of 0.71. The random forest model that was identified as the best tested, performed an accuracy of 0.77 (Figure 1) and it identified as most informative parent- and teacher-rated DSM-oriented ADHD symptoms (Figure 2). Figure 1: Random forest confusion matrix and statistics. Figure 2: Ranking of variables importance. Conclusions A random forest classifier could represent an effective algorithm to support the identification of ADHD children and to simplify the diagnostic process as an initial step. The use of supervised machine learning algorithms could be useful in helping the diagnostic process, highlighting the importance of a personalized medicine approach. Disclosure No significant relationships.
更多查看译文
关键词
machine learning, Personalized medicine, Attention, Deficit Hyperactivity Disoder, Diagnostic classification
AI 理解论文
溯源树
样例
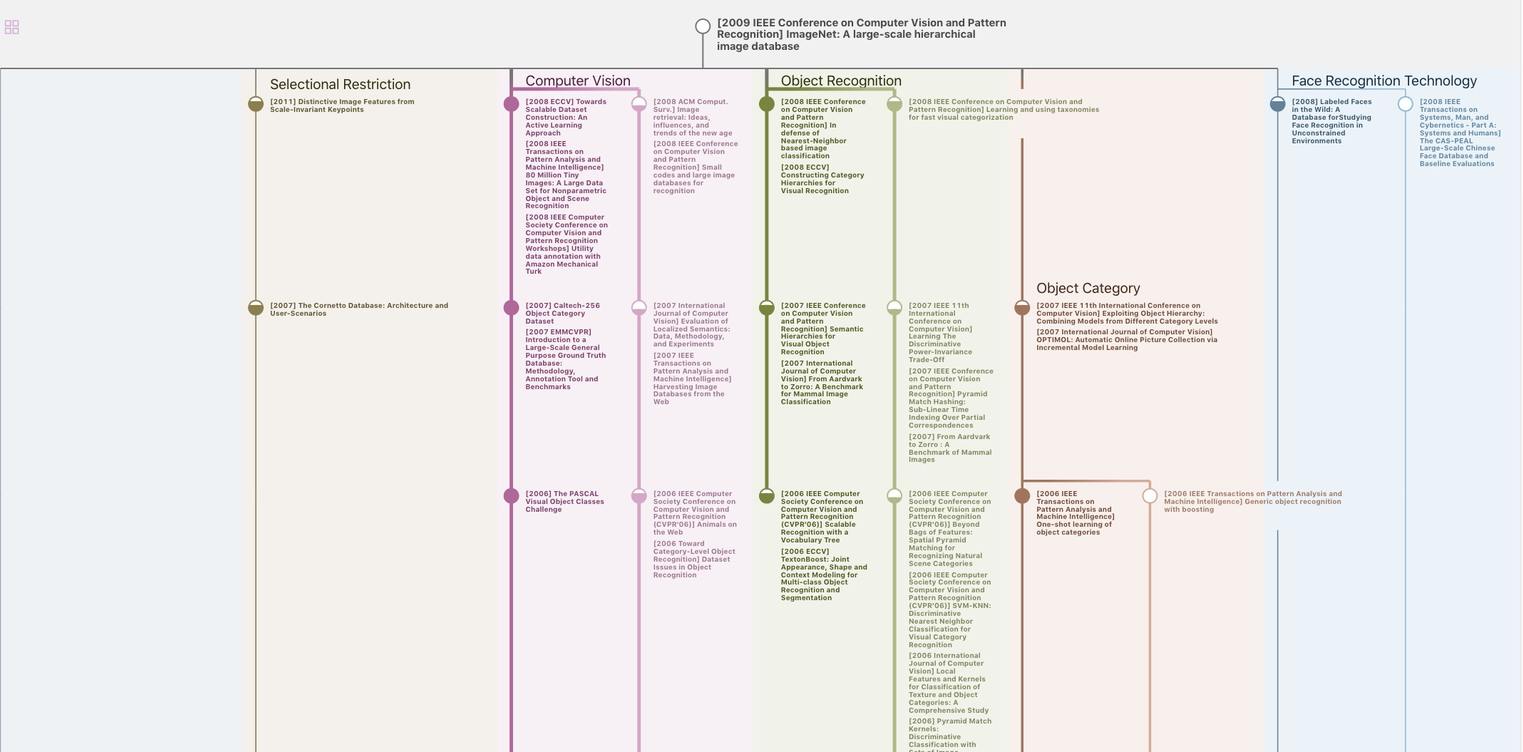
生成溯源树,研究论文发展脉络
Chat Paper
正在生成论文摘要