Seq-UPS: Sequential Uncertainty-aware Pseudo-label Selection for Semi-Supervised Text Recognition
WACV(2023)
摘要
This paper looks at semi-supervised learning (SSL) for image-based text recognition. One of the most popular SSL approaches is pseudo-labeling (PL). PL approaches assign labels to unlabeled data before re-training the model with a combination of labeled and pseudo-labeled data. However, PL methods are severely degraded by noise and are prone to over-fitting to noisy labels, due to the inclusion of erroneous high confidence pseudo-labels generated from poorly calibrated models, thus, rendering threshold-based selection ineffective. Moreover, the combinatorial complexity of the hypothesis space and the error accumulation due to multiple incorrect autoregressive steps posit pseudo-labeling challenging for sequence models. To this end, we propose a pseudo-label generation and an uncertainty-based data selection framework for semi-supervised text recognition. We first use Beam-Search inference to yield highly probable hypotheses to assign pseudo-labels to the unlabelled examples. Then we adopt an ensemble of models, sampled by applying dropout, to obtain a robust estimate of the uncertainty associated with the prediction, considering both the character-level and word-level predictive distribution to select good quality pseudo-labels. Extensive experiments on several benchmark handwriting and scene-text datasets show that our method outperforms the baseline approaches and the previous state-of-the-art semi-supervised text-recognition methods.
更多查看译文
关键词
Algorithms: Machine learning architectures,formulations,and algorithms (including transfer),low-shot,semi-,self-,and un-supervised learning
AI 理解论文
溯源树
样例
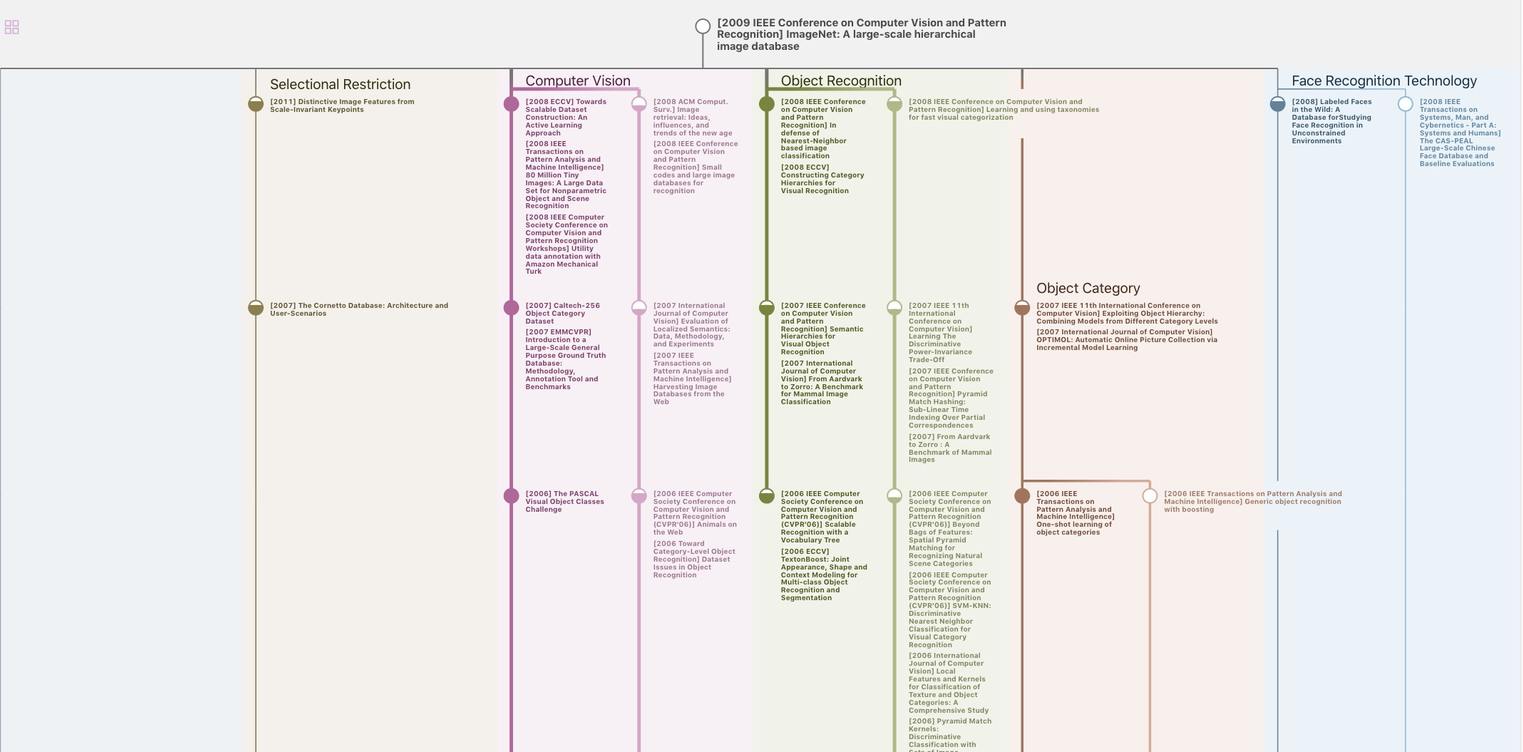
生成溯源树,研究论文发展脉络
Chat Paper
正在生成论文摘要