Online Meta-Learning for Model Update Aggregation in Federated Learning for Click-Through Rate Prediction
arxiv(2022)
摘要
In Federated Learning (FL) of click-through rate (CTR) prediction, users' data is not shared for privacy protection. The learning is performed by training locally on client devices and communicating only model changes to the server. There are two main challenges: (i) the client heterogeneity, making FL algorithms that use the weighted averaging to aggregate model updates from the clients have slow progress and unsatisfactory learning results; and (ii) the difficulty of tuning the server learning rate with trial-and-error methodology due to the big computation time and resources needed for each experiment. To address these challenges, we propose a simple online meta-learning method to learn a strategy of aggregating the model updates, which adaptively weighs the importance of the clients based on their attributes and adjust the step sizes of the update. We perform extensive evaluations on public datasets. Our method significantly outperforms the state-of-the-art in both the speed of convergence and the quality of the final learning results.
更多查看译文
关键词
federated meta-learning,model update aggregation,prediction,click-through
AI 理解论文
溯源树
样例
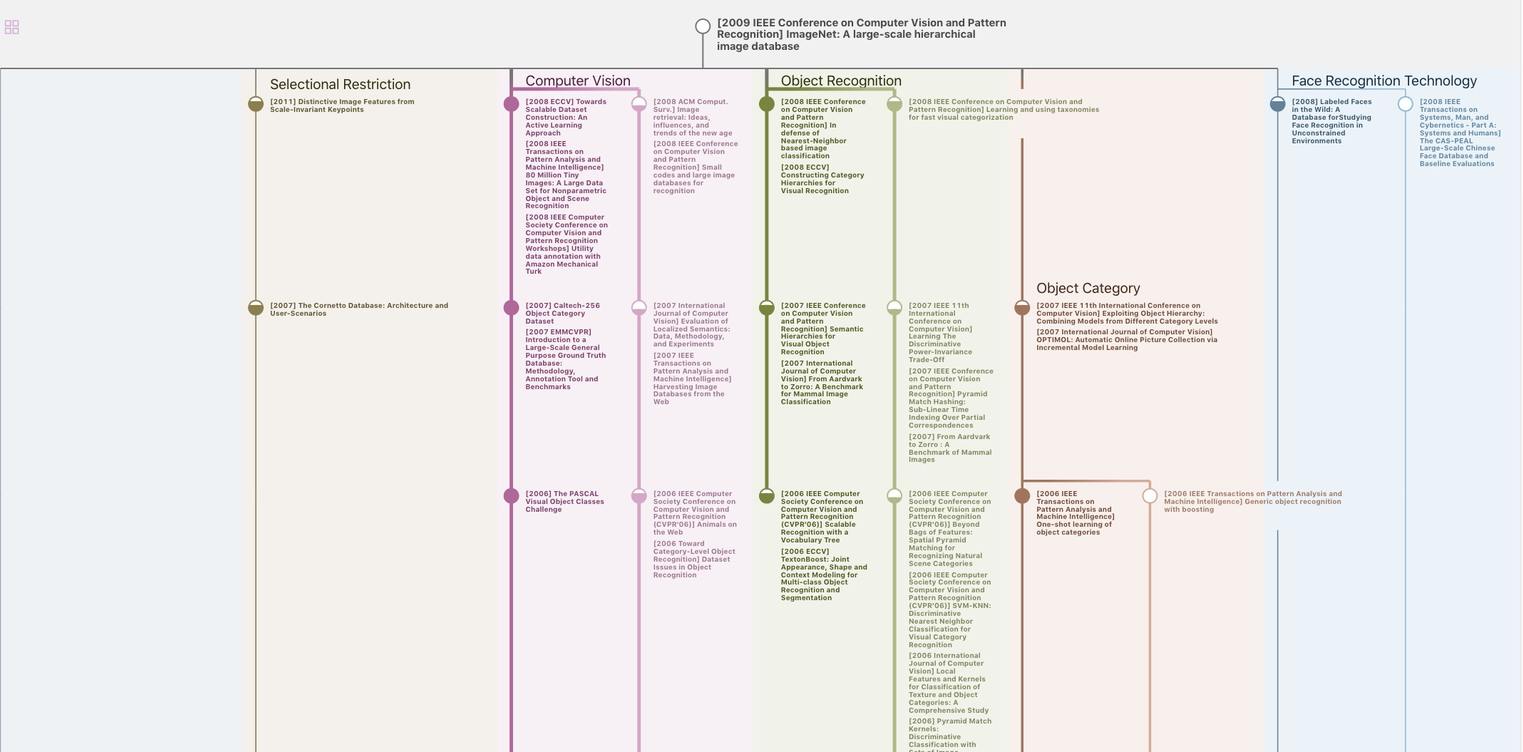
生成溯源树,研究论文发展脉络
Chat Paper
正在生成论文摘要